Agent Evaluation
Agent evaluation is especially challenging because LLMs are non-deterministic—agents can follow strange paths and still arrive at the right answer, making debugging difficult. Effective agent evaluation requires looking beyond final outputs to assess what the agent knows, what actions it takes, and how it plans. We’ve created agent evaluation templates for every stage of the process, from tool use to planning and reflection, combining traditional LLM evaluation methods with agent-specific diagnostics.
How Do I Evaluate AI Agents?
Building a good agent is hard.
Setting up a basic agent on the other hand, is straightforward – especially if you use a framework and a common architecture. However, the difficulty lies in taking that basic agent and turning it into a robust, production-ready tool. This is also where much of the value in your agent comes from.
Evaluation is one of the main tools that will help you transform your agent from a simple demo project into a production tool. Using a thoughtful and structured approach to evaluation is one of the easiest ways to streamline this otherwise challenging process.
Agent Development is Cyclical, Not Linear
Building any LLM application involves some amount of cyclical iteration, and agents are no different. It is impossible to anticipate all of the queries your agent will receive, or all of the possible outputs of your models. Only by properly monitoring your production system and integrating the data generated by it into your development processes can you create a truly robust system.
That cycle of iteration typically involves:
- Creating an initial set of representative test cases
- Breaking down your agent into individual steps (e.g. router, skill 1, skill 2, etc.)
- Creating evaluators for each step
- Experimenting with different iterations of your agent, maximizing your eval scores
- Monitoring your agent in production
- Revising your test cases, steps, and evaluators based on production data
- Repeating steps 4-6
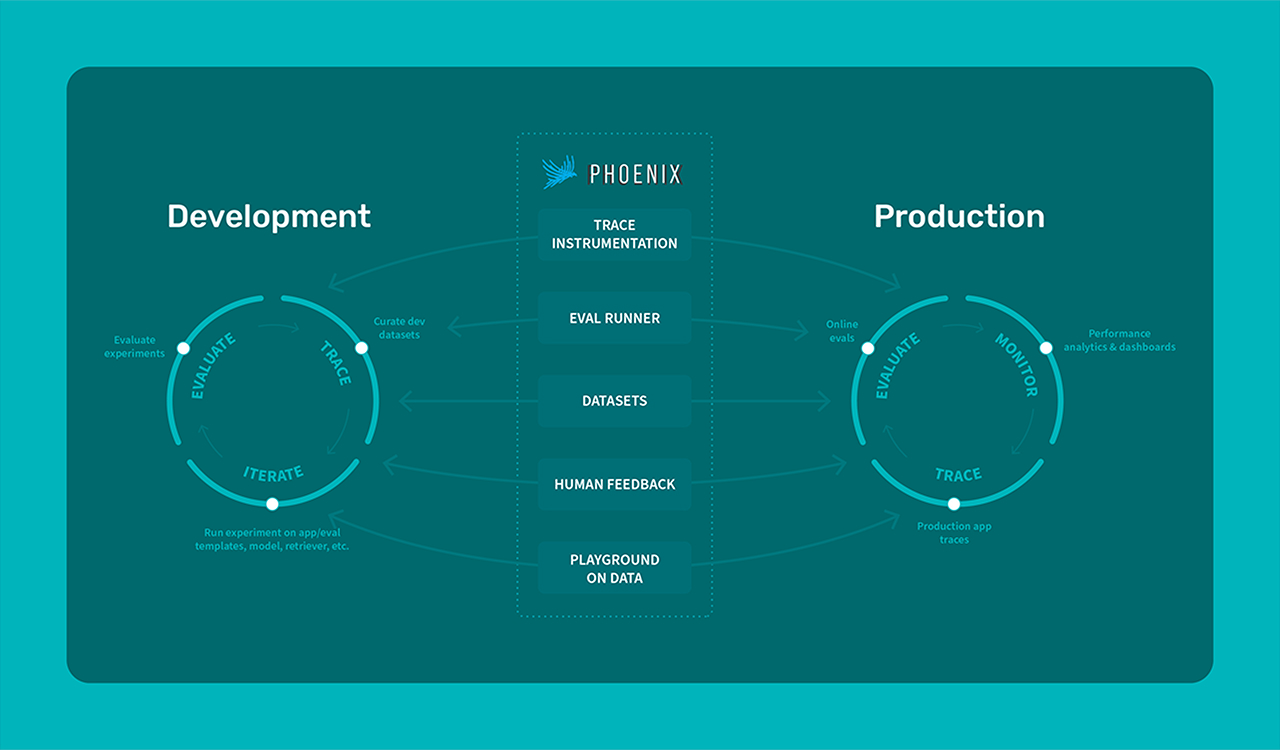
Building a Set of Test Cases
Having a standard set of test cases allows us to test changes to our agent, avoid unexpected regressions, and provides a benchmark for evaluation.
This set doesn’t need to be long but should be comprehensive. For example, if you’re building a chatbot agent for your website, your test cases should include all types of queries the agent supports. This might involve queries that trigger each of your functions or skills, some general information queries, and a set of off-topic queries that your agent should not answer.
Your test cases should cover all the paths your agent can take. You don’t need thousands of test cases if they are just rephrasings of other cases.
Test cases will evolve over time as you discover new types of inputs. Don’t be afraid to add to them, even if doing so makes some evaluation results less comparable to historical runs.
Choosing Which Steps of Your Agent to Evaluate
Next, we split our agent into manageable steps that we want to evaluate individually. These steps should be fairly granular, encompassing individual operations.
Each of your agent’s functions, skills, or execution branches should have some form of evaluation to benchmark their performance. You can get as granular as you’d like. For example, you could evaluate the retrieval step of a RAG skill or the response of an internal API call.
Beyond the skills, it’s critical to evaluate the router on a few axes, which we’ll touch on below. If you’re using a router, this is often where the biggest performance gains can be achieved.
As you can see, the list of “pieces” to evaluate can grow quickly, but that’s not necessarily a bad thing. We recommend starting with many evaluations and trimming down over time, especially if you’re new to agent development
Building Evaluators for Each Step
With our steps defined, we can now build evaluators for each one. Many frameworks, including Arize’s Phoenix library, can help with this. You can also code your own evaluations, which can be as simple as a string comparison depending on the type of evaluation.
Generally, evaluation can be performed by either comparing outputs to expected outputs or using a separate LLM as a judge. The former approach is great if you have expected outputs to compare to, as it is a deterministic approach. LLM-as-a-judge is helpful when there is no ground truth or when you’re aiming for more qualitative evaluation.
Evaluating the skill steps of an agent is similar to evaluating those skills outside of the agent. If your agent has a RAG skill, for example, you would still evaluate both the retrieval and response generation steps, calculating metrics like document relevance and hallucinations in the response.
Common Skill Evaluations
For RAG Skills:
- Retrieval Relevance
- QA Correctness
- Hallucination
- Reference / Citation
For Code-Gen Skills:
- Code readability
- Code correctness
For API Skills:
- Code-based integration tests and unit tests
For All skills:
- Comparison against ground truth data
- For more on skill evaluations, see our LLM Evaluation handbook.
Evaluating a Router
Beyond skills, agent evaluation becomes more unique.
In addition to evaluating the agent’s skills, you need to evaluate the router and the path the agent takes.
The router should be evaluated on two axes: first, its ability to choose the right skill or function for a given input; second, its ability to extract the right parameters from the input to populate the function call.
Choosing the right skill is perhaps the most important task and one of the most difficult. This is where your router prompt (if you have one) will be put to the test. Low scores at this stage usually stem from a poor router prompt or unclear function descriptions, both of which are challenging to improve.
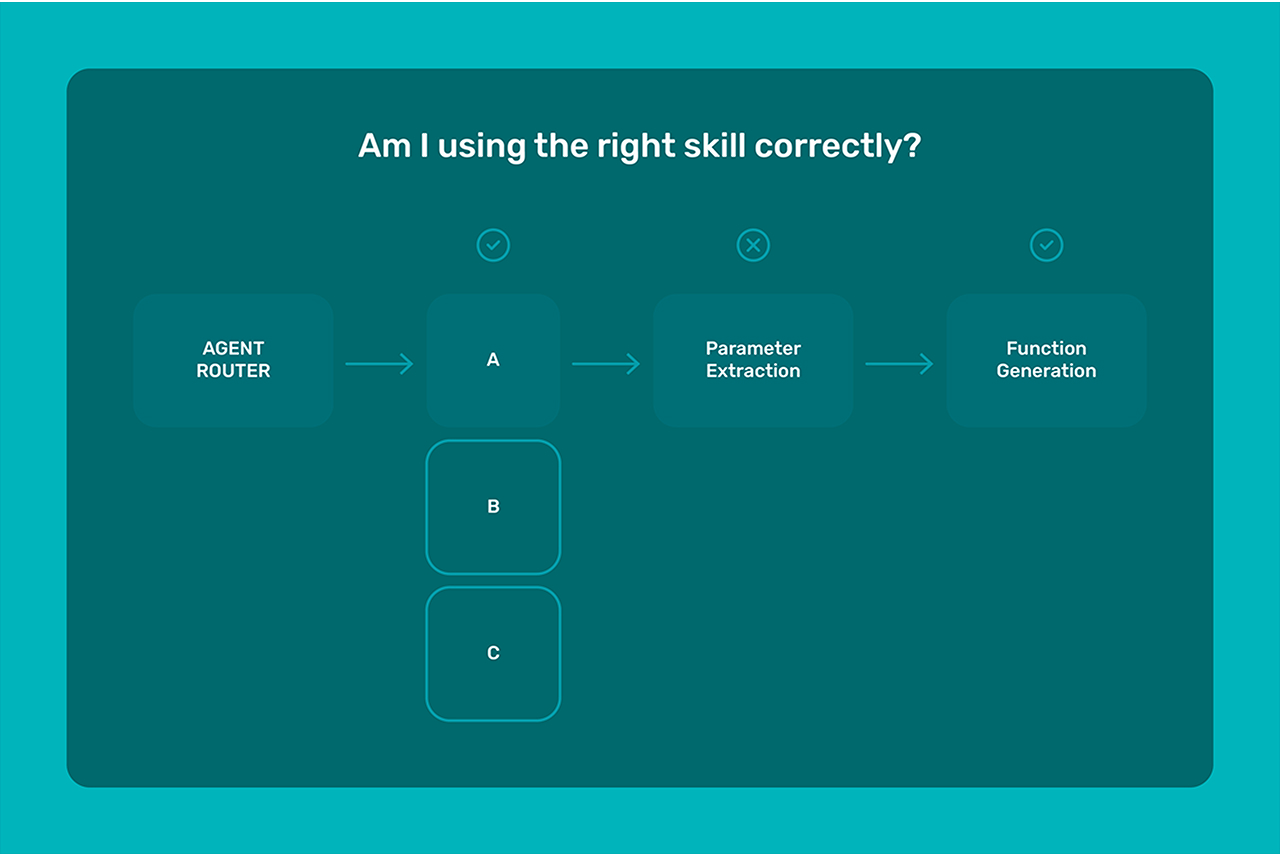
Extracting the right parameters is also tricky, especially when parameters overlap. Consider adding some curveballs into your test cases, like a user asking for an order status while providing a shipping tracking number, to stress-test your agent.
Arize provides built-in evaluators to measure tool call accuracy using an LLM as a judge, which can assist at this stage.
Evaluating your Agent’s Path
Lastly, evaluate the path the agent takes during execution. Does it repeat steps? Get stuck in loops? Return to the router unnecessarily? These “path errors” can cause the worst bugs in agents.
To evaluate the path, we recommend adding an iteration counter as an evaluation. Tracking the number of steps it takes for the agent to complete different types of queries can provide a useful statistic.
However, the best way to debug agent paths is by manually inspecting traces. Especially early in development, using an observability platform and manually reviewing agent executions will provide valuable insights for improvement.
Convergence
This can then be extend to measure convergence. Convergence in the context of agents refers to how often your agent takes the optimal path for a given query. Is your agent “converging” towards a critical path for a given query? Convergence allows you to measure this.
To calculate this numeric score:
- Run your agent on a set of similar queries
- Record the number of steps taken for each, as well as the overall minimum number of steps for a given run
- Calculate the convergence score: ∑ (minimum steps taken for this type of query / steps in the run)
This will give you a numeric 0-1 value of how often your agent is taking the optimal path for that type of query, and by how much it diverges when it takes a suboptimal path.
It’s important to note however that because the optimal path is calculated by the shortest run of your agent, this technique will miss cases where every run of your agent takes a suboptimal path.
Experimenting and Iterating
With your evaluators and test cases defined, you’re ready to modify your agent. After each major modification, run your test cases through the agent, then run each of your evaluators on the output or traces. You can track this process manually or use Arize to track experiments and evaluation results.
While there’s no one-size-fits-all approach to improving your agent, this evaluation framework gives you much greater visibility into your agent’s behavior and performance. It will also give you peace of mind that changes haven’t inadvertently broken other parts of your application.
More Agent Evaluation Resources
- Watch our webinar on how to systematically evaluate agents
- Check out this post on how to trace and evaluate LangGraph agents
- Watch an overview of agent evaluation and assistant evaluation
Congratulations, you’ve reached the end of Arize’s Agent Handbook! 🎉
You’ve learned what agents are, how they’re made, different agent architectures and organization structures, and how to evaluate your agents.
If you have any questions, or just want to share what you’re working on, come join our team in Slack.
Now, go forth and build!