A New Standard for Evaluating Hallucinations in Retrieval-Augmented Generation (RAG)
Hallucinations—responses that appear credible but are not grounded in retrieved information—pose significant challenges, especially in high-stakes domains like healthcare, finance, and law. LibreEval addresses this with stronger evaluators, a flexible dataset creation pipeline, and support for tuning and evaluation using both custom and prebuilt datasets. Alongside the platform, we release LibreEval1.0, the largest open-source RAG hallucination dataset, and fine-tuned Qwen2-1.5B-Instruct models that improve hallucination detection at a fraction of the cost of SOTA LLMs.
How it works:
- Generate RAG Hallucination Datasets
Upload your reference data and let LibreEval generate structured hallucination evaluation datasets. Or use - Evaluate Models on Open Benchmarks
Run hallucination detection tests on LibreEval1.0 or external datasets like HaluEval1.0 and ARES. - Fine-Tune Open Models for Better Detection
Use LibreEval’s fine-tuning scripts to improve recall and precision on open models like Qwen2-1.5B.
Overview
The Largest Open RAG Hallucination Dataset
LibreEval1.0 includes 72,155 samples across seven languages and six key domains:
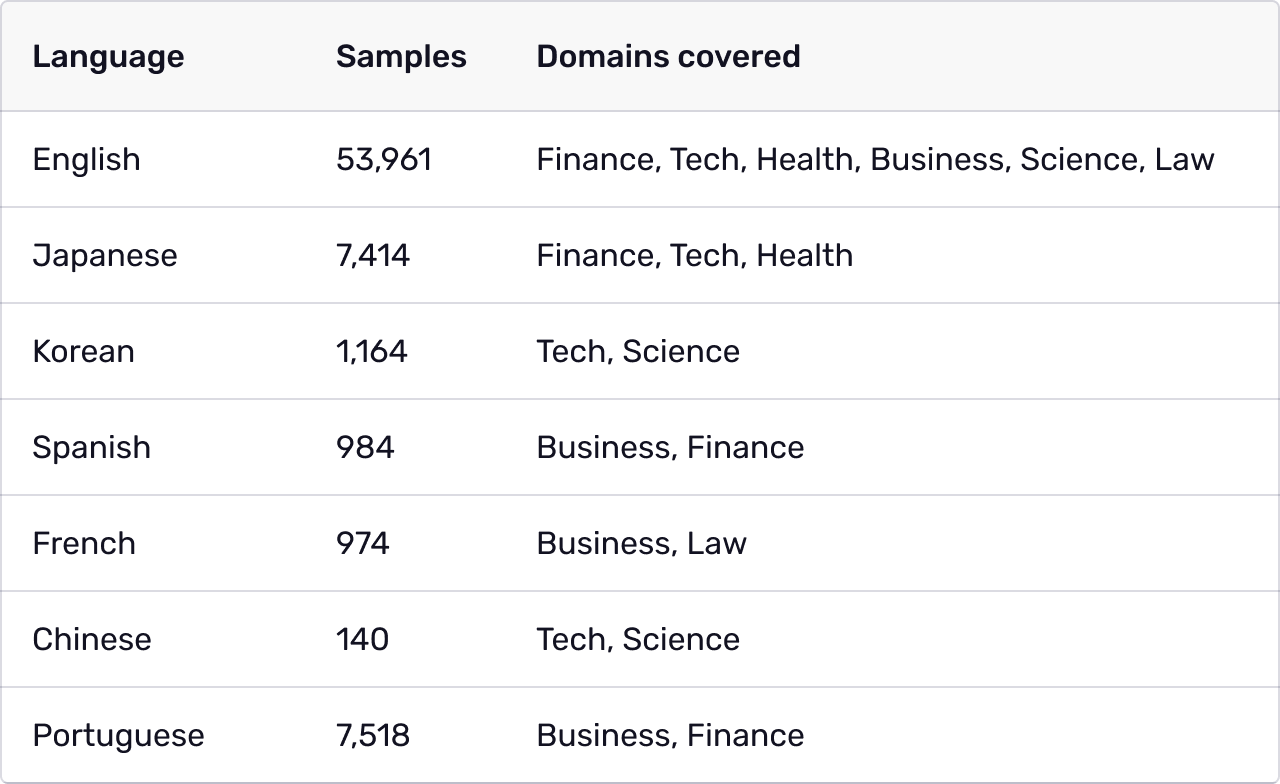
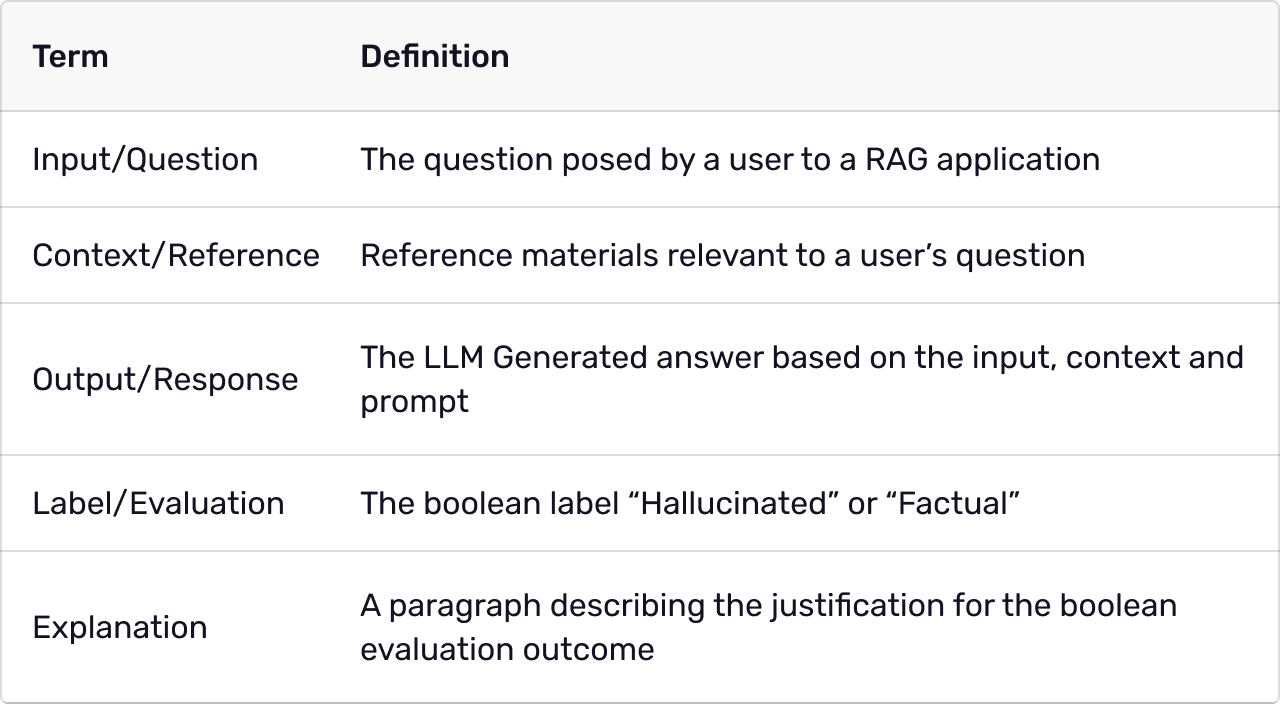
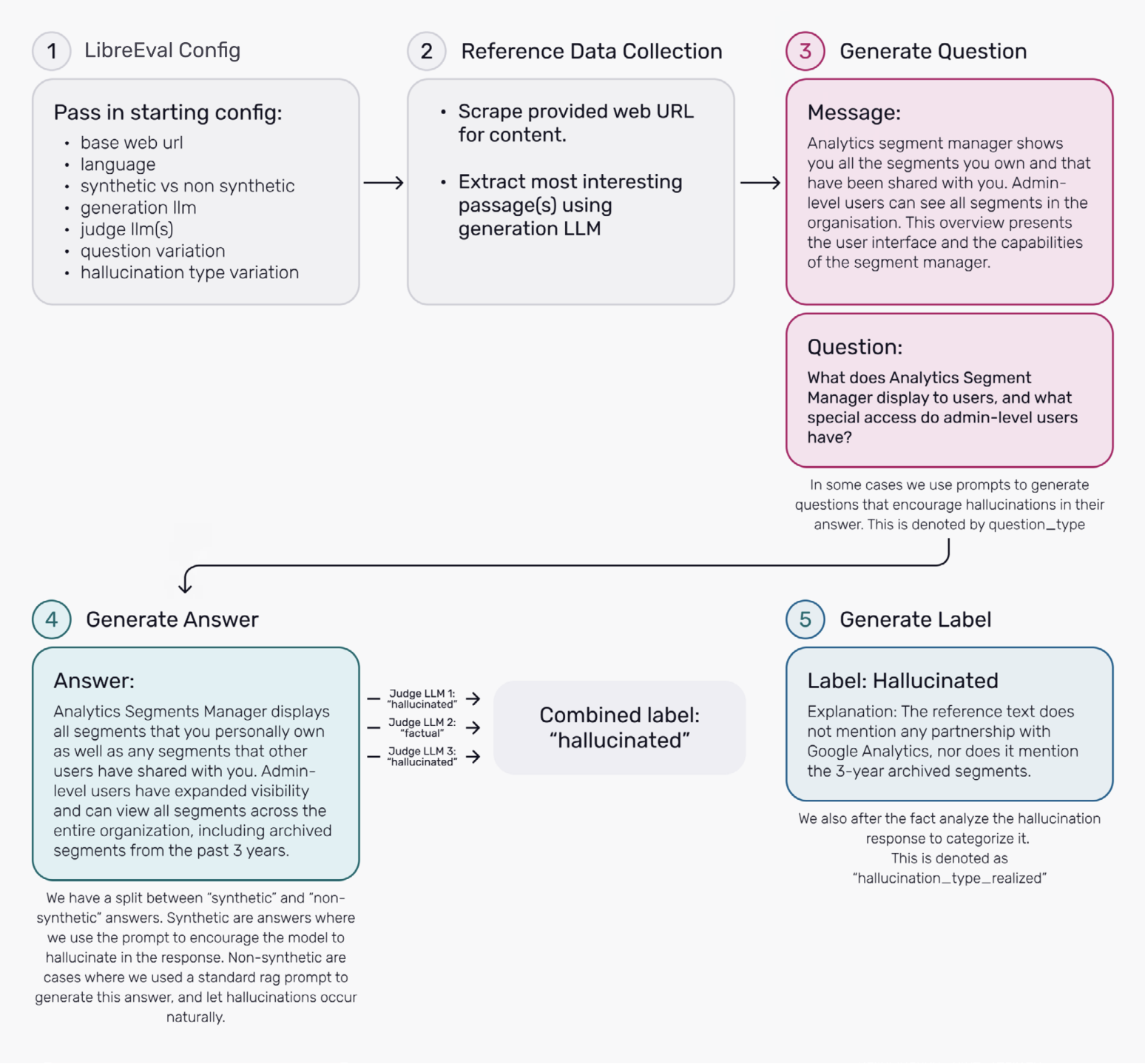
Why It Matters: LibreEval1.0 provides an unparalleled benchmark for evaluating hallucinations in RAG applications, with both synthetic and naturally occurring hallucinations.
Fine-Tuned Models: Closing the Gap with Proprietary LLMs
LibreEval includes fine-tuned hallucination detection models that outperform their base counterparts in recall and F1 score, providing a cost-effective alternative to proprietary solutions.
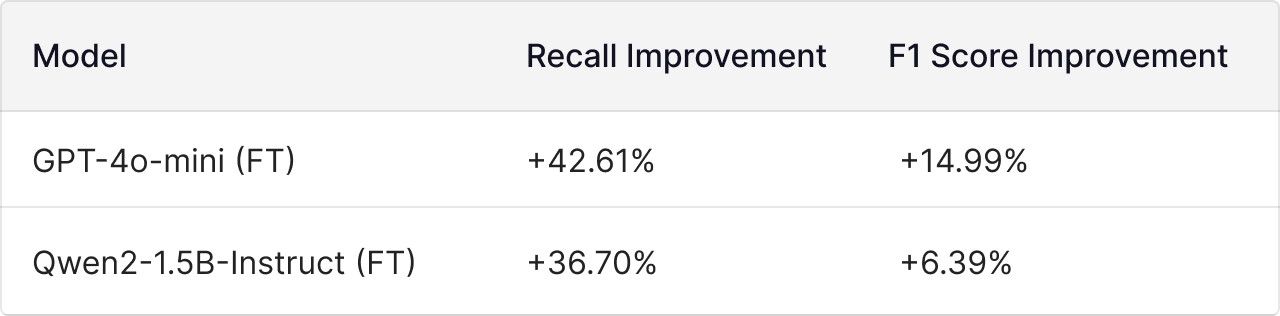
Key Insight: Fine-tuned models on LibreEval 1.0 rival proprietary SLMs like GPT-4o-mini while reducing operational costs.
Key findings
Advanced Hallucination Analysis
LibreEval provides in-depth insights into hallucination types and how well models detect them. Our findings include:
- Requests requiring advanced logical reasoning are the most hallucination-prone.
- Relation-error and incompleteness hallucinations are the most difficult types of hallucinations for models to detect.
- Synthetic hallucinations are far more challenging for models to detect than naturally occurring ones.
Agreement Between Human and AI Judges:
- LLM-based ensemble evaluation outperformed human annotators in hallucination detection (92% vs. 85% accuracy).
Dataset Composition
Hallucination Type Distribution
LibreEval1.0 includes 72,155 samples across seven languages and six key domains:
Relation-error hallucinations (32.0%) are the most common, followed by incompleteness (22.2%) and overclaims (17.7%). Unverifiable (10.2%), outdated (9.8%), and entity-error (8.1%) hallucinations pose risks in high-stakes domains like law, medicine, and finance, making them critical for model training.
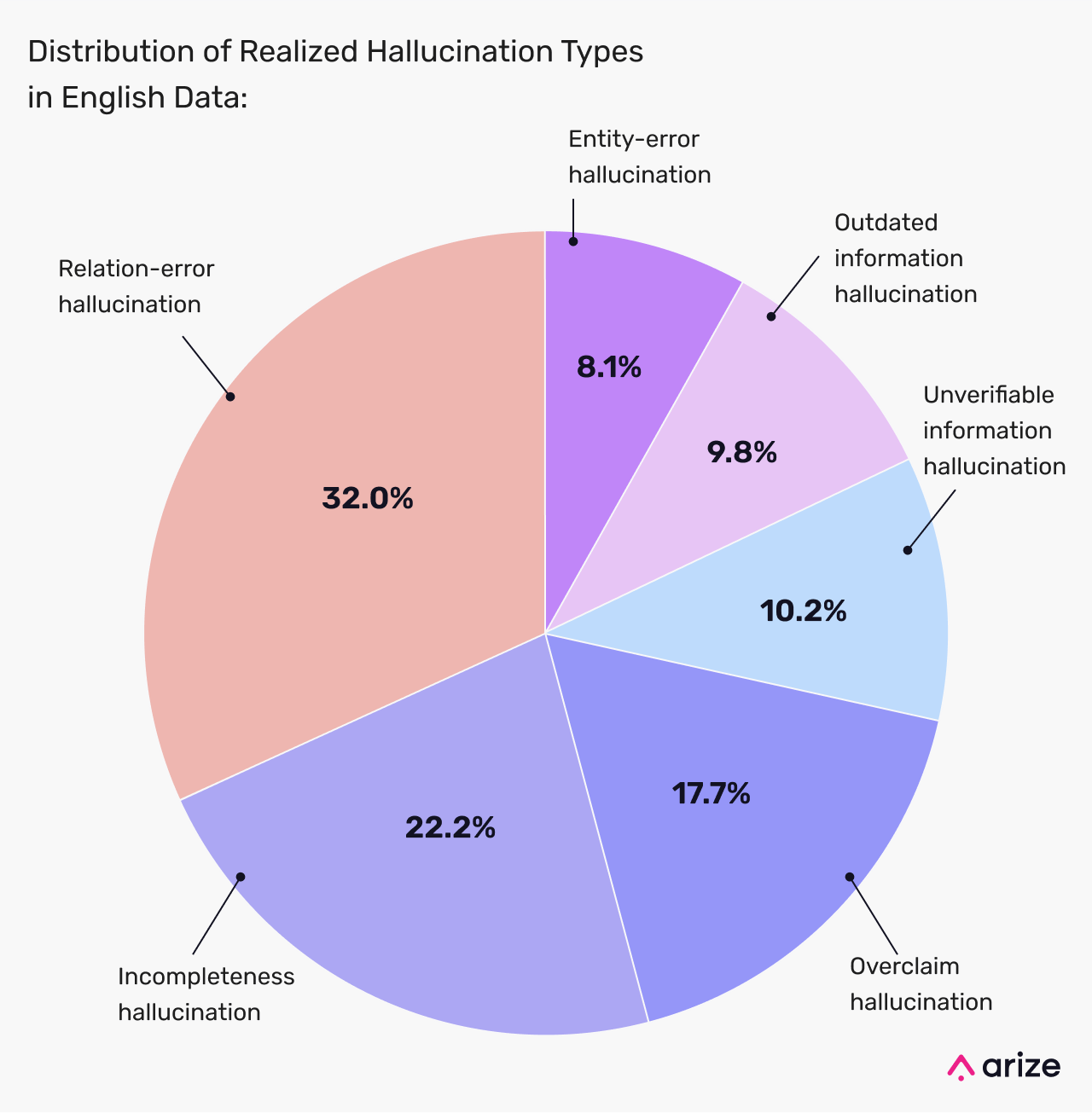
Hallucination Categories: Synthetic vs. Non-Synthetic
In non-synthetic data, Incompleteness and Relation-Error Hallucinations dominate, while Outdated, Unverifiable, and Overclaim Hallucinations are rare. Synthetic data amplifies all hallucination types, especially Relation-Error and Incompleteness, making them the most frequent overall. The scarcity of Outdated Information Hallucinations in non-synthetic data suggests overlap in training data between response-generating and hallucination-judging models.
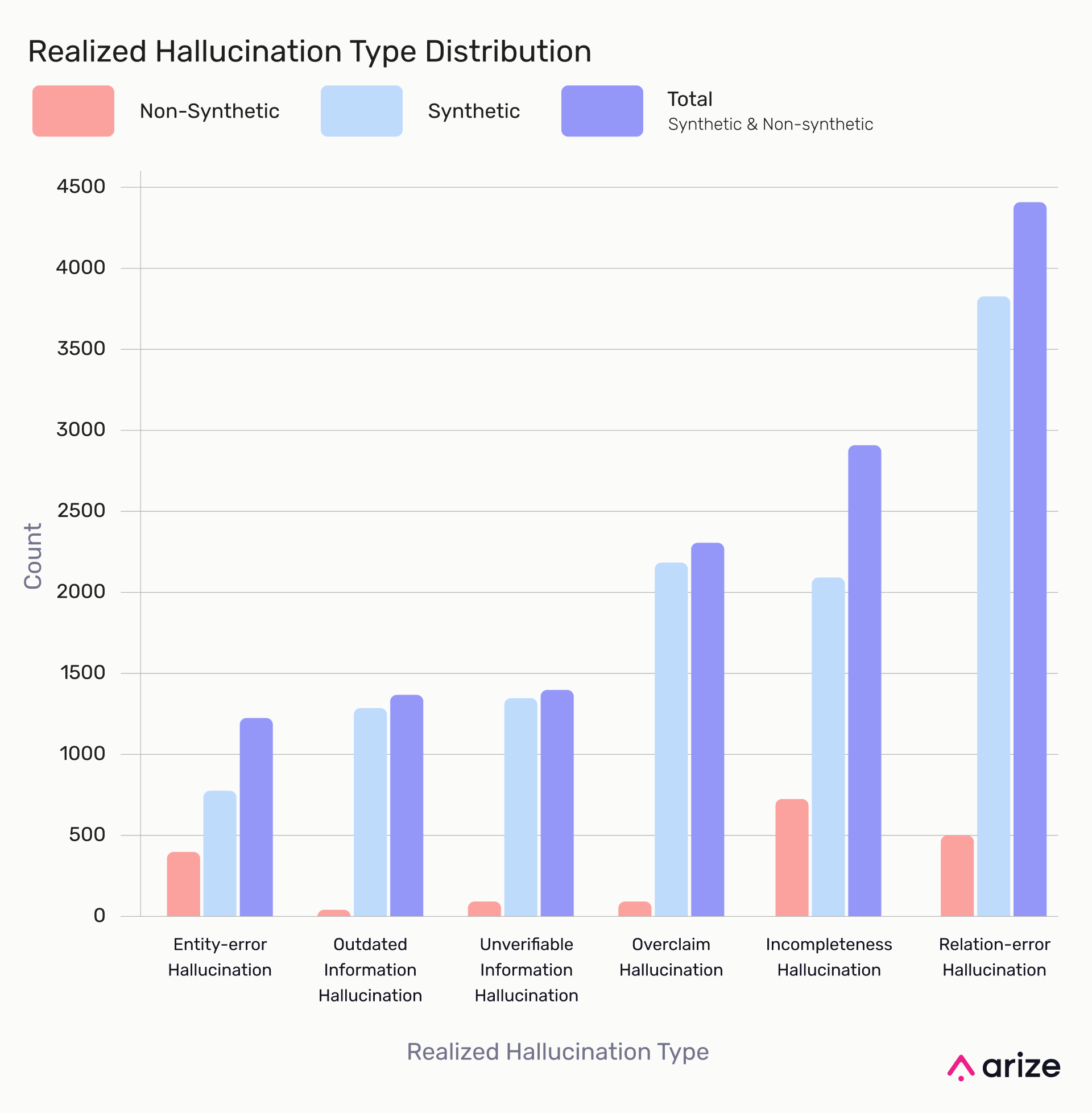
Hallucination Detection Rates by Model
Relation-Error and Incompleteness Hallucinations have the highest detection rates, indicating models struggle with factual consistency and completeness. Entity-Error and Outdated Information Hallucinations are least detected, suggesting models rarely fabricate named entities or outdated facts. Synthetic data consistently shows higher hallucination rates, reinforcing its greater challenge for models due to adversarial prompts and increased complexity.
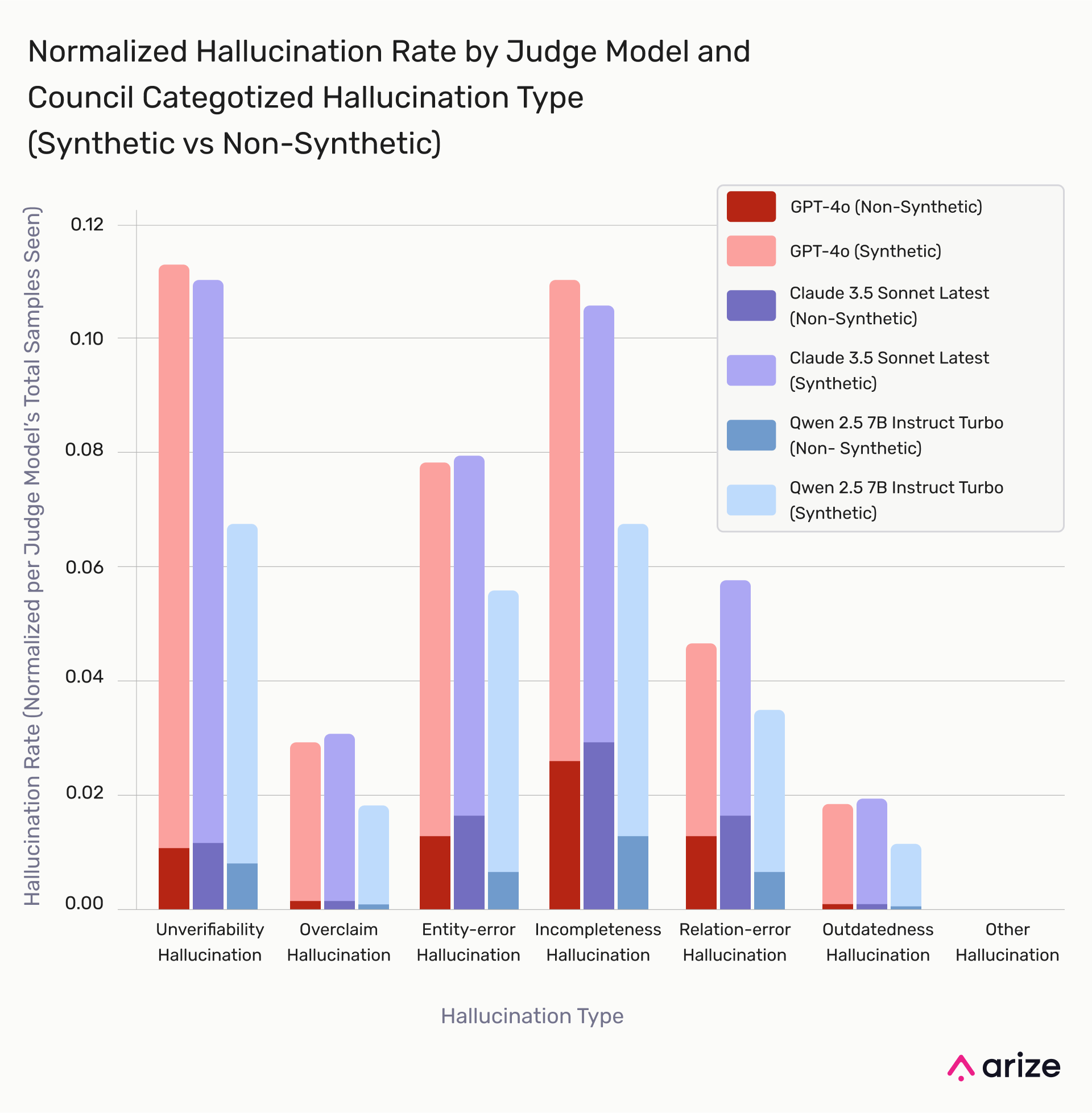
Model Performance
Model Performance on LibreEval1.0 (English Dataset)
Claude-3.5-Sonnet and GPT-4o performed well, while Qwen2 struggled across all metrics. Surprisingly, fine-tuning on multilingual data improved F1 scores more than English-only training. Some test questions may be semantically similar to training data, so results primarily highlight post-training improvements rather than absolute performance.
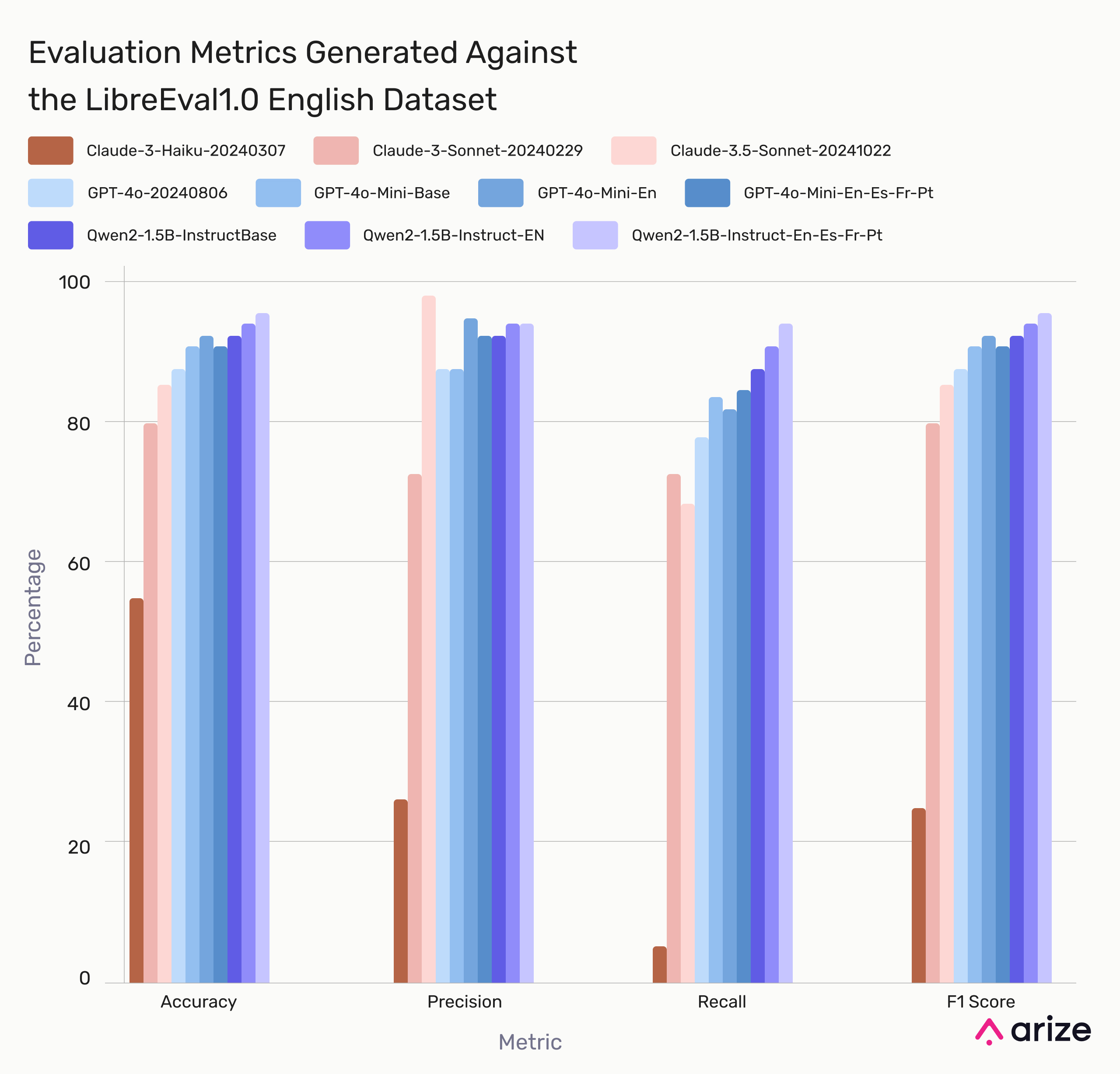
Model Performance by Web Domain Category
LibreEval1.0 evaluations show GPT-4o-mini had the lowest initial performance but outperformed other base models after fine-tuning. Results serve as a benchmark for domain-specific performance, though some test questions may overlap with training data.
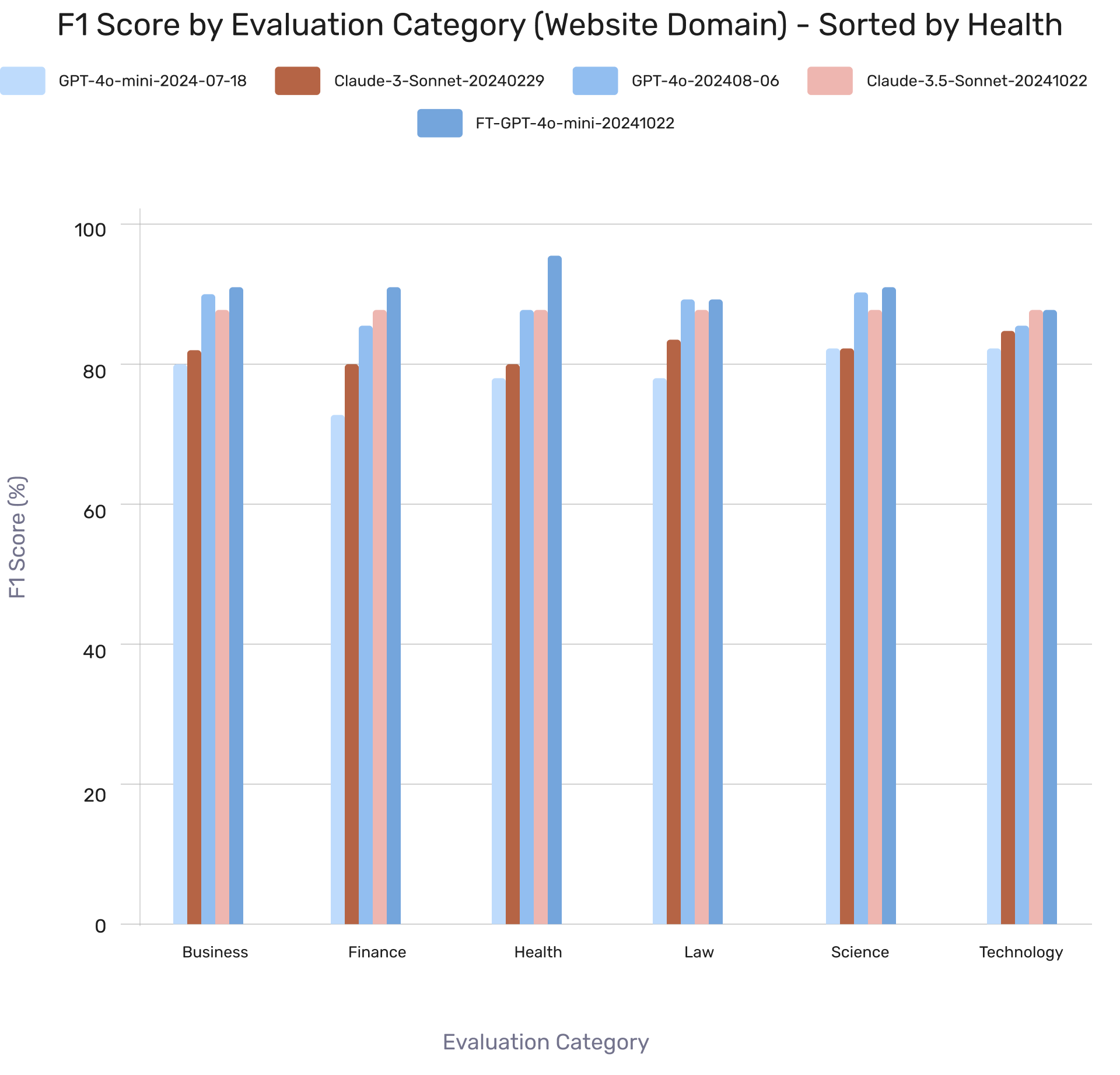
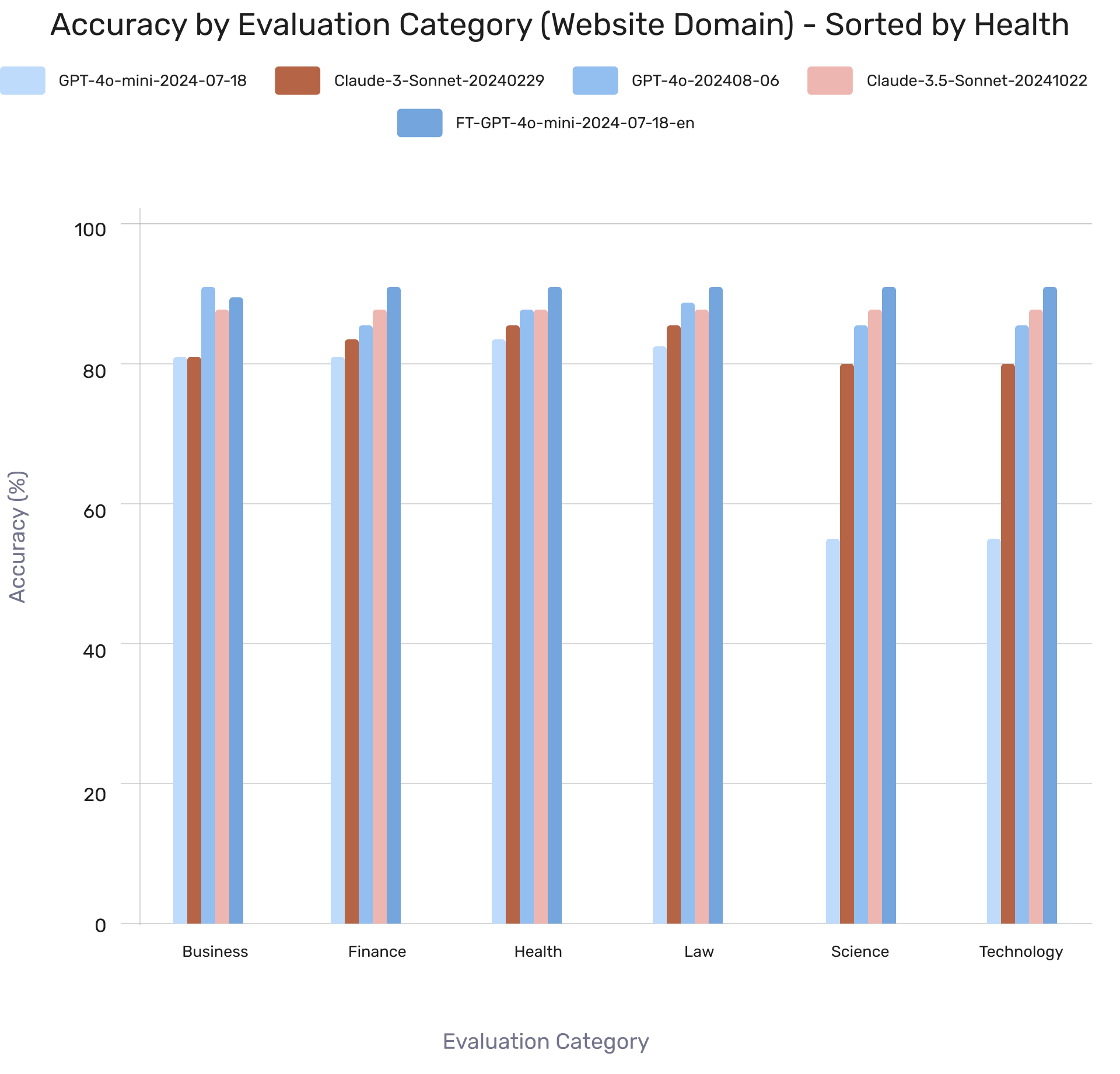
Model Performance: Synthetic vs. Non-Synthetic
Base models struggle more with non-synthetic hallucinations, showing ~35% lower recall compared to synthetic cases. This suggests that datasets relying on synthetic hallucinations may overestimate model performance in real-world scenarios.
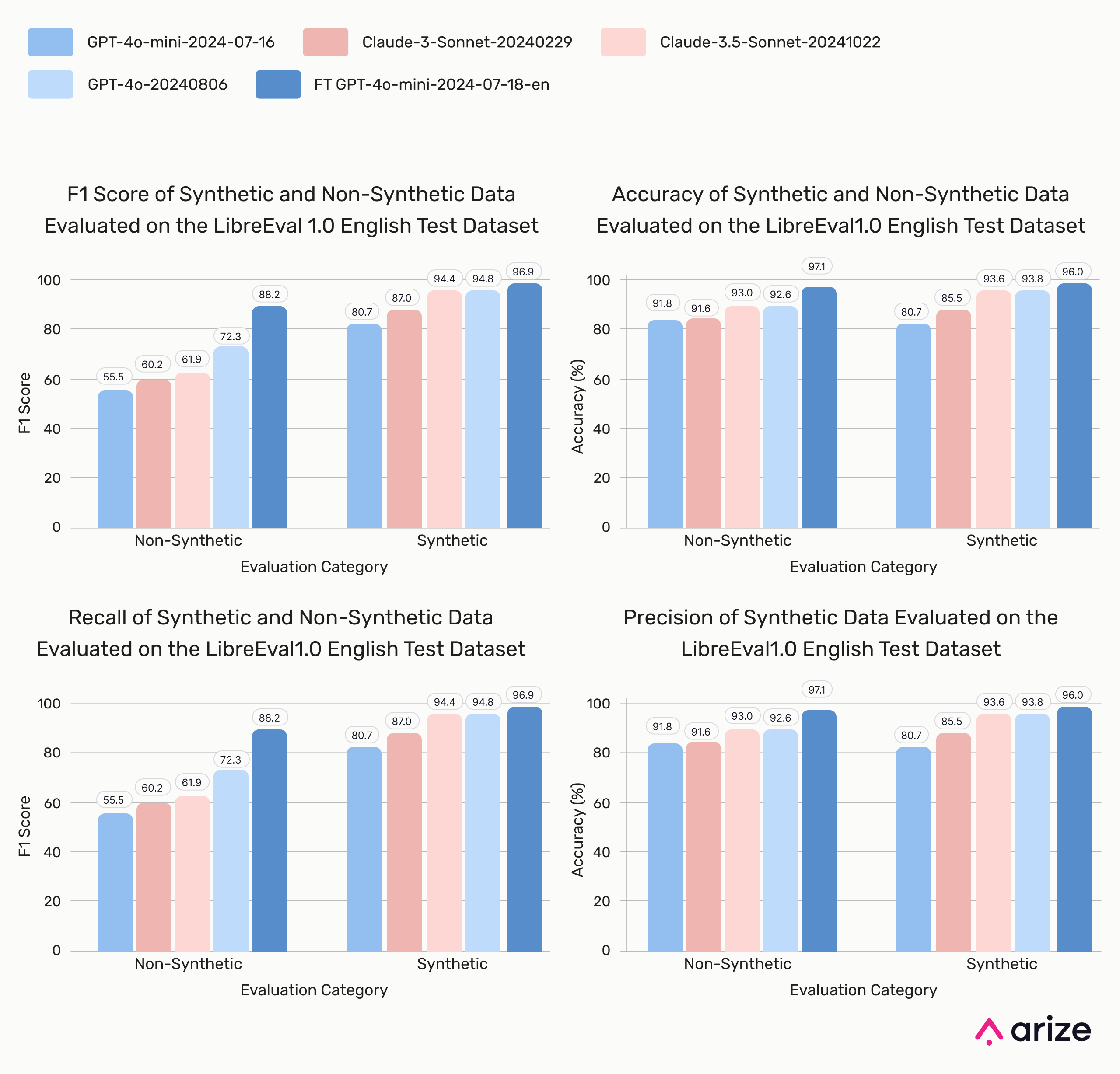
Fine-Tuned Model Performance on HaluEval1.0
Qwen2 and GPT-4o-mini fine-tunes outperformed their base models, with Qwen2 closing a 38% F1 gap to just 7-8% after fine-tuning on English and multilingual data.
Precision dropped (Qwen2: 13-16%, GPT-4o-mini: 2-8%), but Qwen2 saw a 29-30% performance boost, while GPT-4o-mini improved by 1-3% across other metrics.
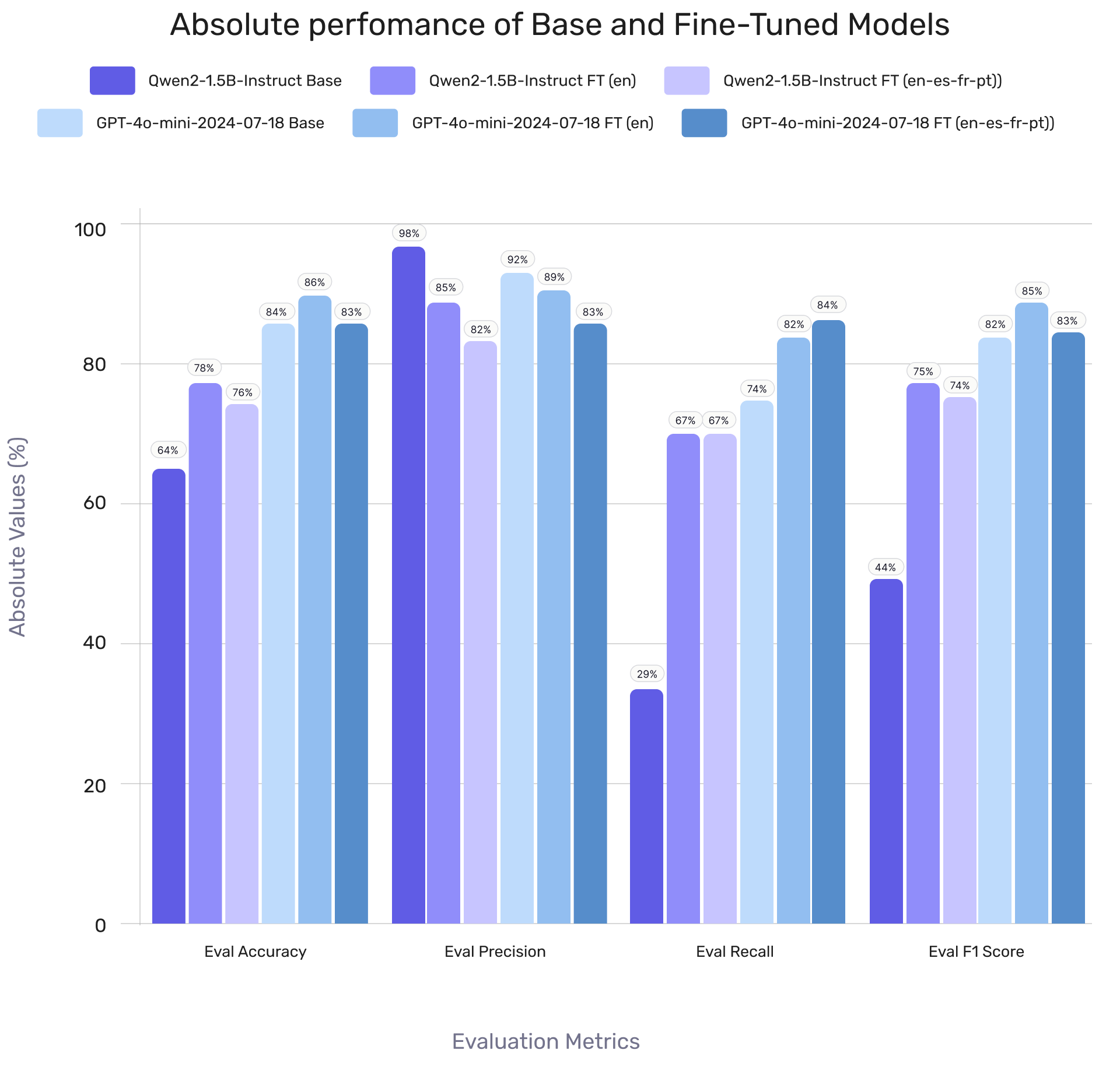
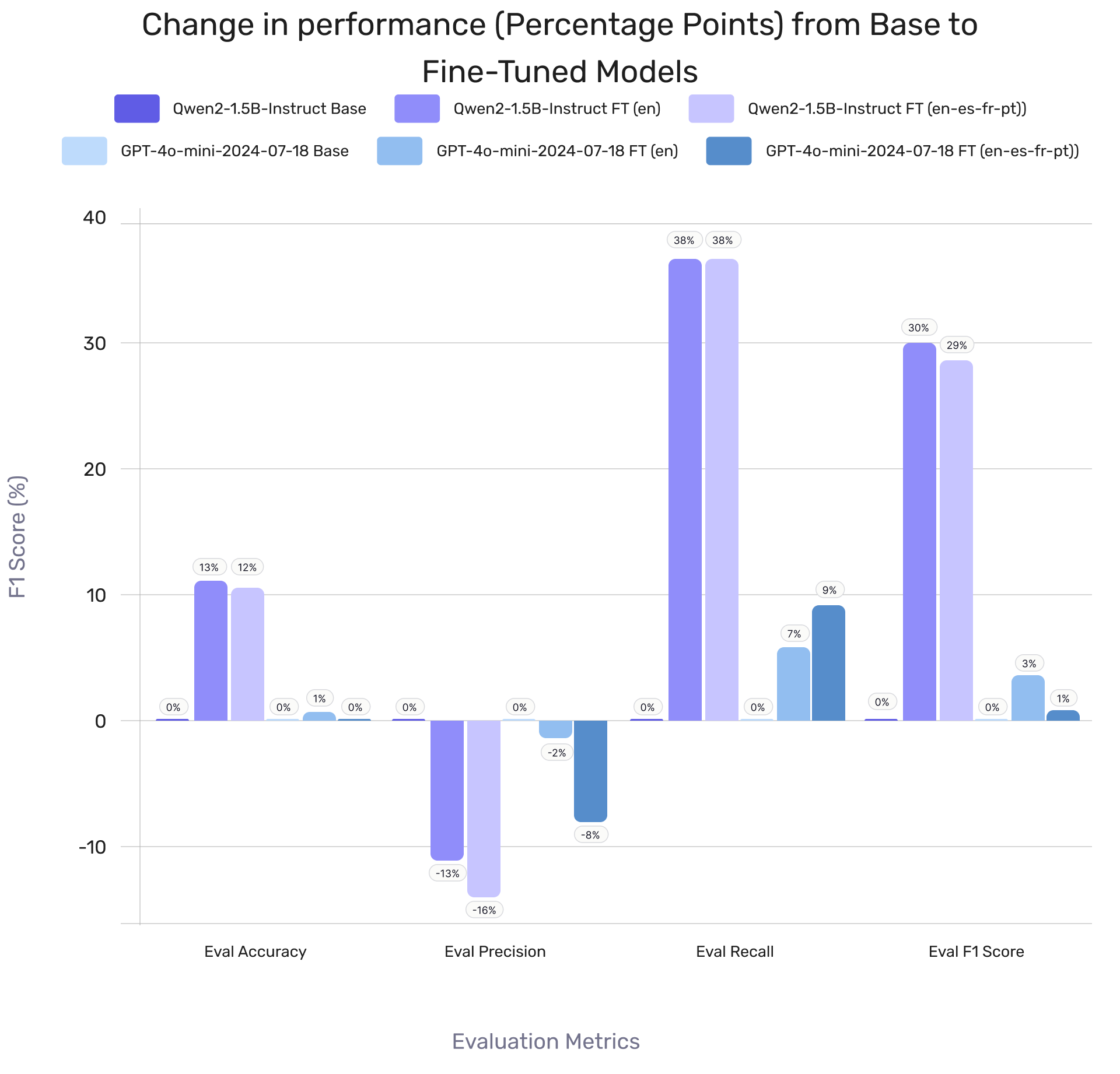
Discussion
Dataset Insights
LibreEval1.0 effectively evaluates hallucinations across domains, question types, and data sources, guiding future dataset improvements. The LLM Council of Judges outperformed human annotators (94% vs. 93%), though disagreements were 4x more common in non-synthetic data, particularly in Incompleteness and Relation-Error Hallucinations.
Model Comparisons
GPT-4o and Claude-3.5-Sonnet excel in precision, but recall varies across hallucination types. Fine-tuned Qwen2-1.5B-Instruct approaches GPT-4o-mini performance (7-8% gap), making it a viable cost-effective alternative for large-scale use.
Challenges & Future Work
Hallucination classification remains ambiguous, requiring refined LLM judge prompts and expanded multilingual datasets. Future efforts should improve robustness against adversarial inputs by incorporating user-generated challenges beyond structured web data.
Conclusion
LibreEval1.0 advances hallucination evaluation research by systematically generating diverse datasets and benchmarking models. Fine-tuned open-source models offer strong alternatives to proprietary solutions, making AI-driven retrieval systems more reliable and cost-effective.
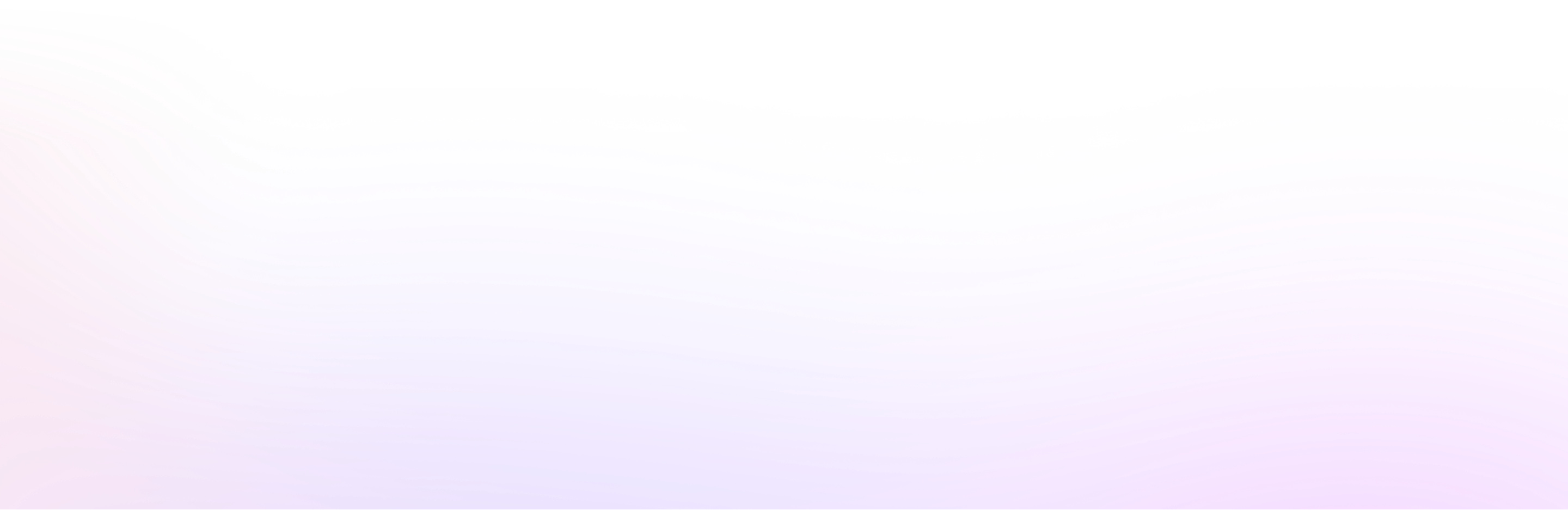