Agent Workflow Memory is a technique for teaching an LLM-based agent to remember and reuse multi-step task solutions (“workflows”) from its past experience. As the agent interacts with an environment (i.e. a web browser), AWM monitors its action sequences and induces common subroutines that led to success. These extracted workflows are stored and later injected as guiding plans when a similar task or goal is encountered. By leveraging previously discovered action sequences, AWM seems to improve long-horizon task success rates (by 51% on the WebArena benchmark) while reducing steps needed. It works both offline (mining training trajectories) and online (during live use) to continually refine the agent’s competence (paper.
What is agent workflow memory (AWM)?
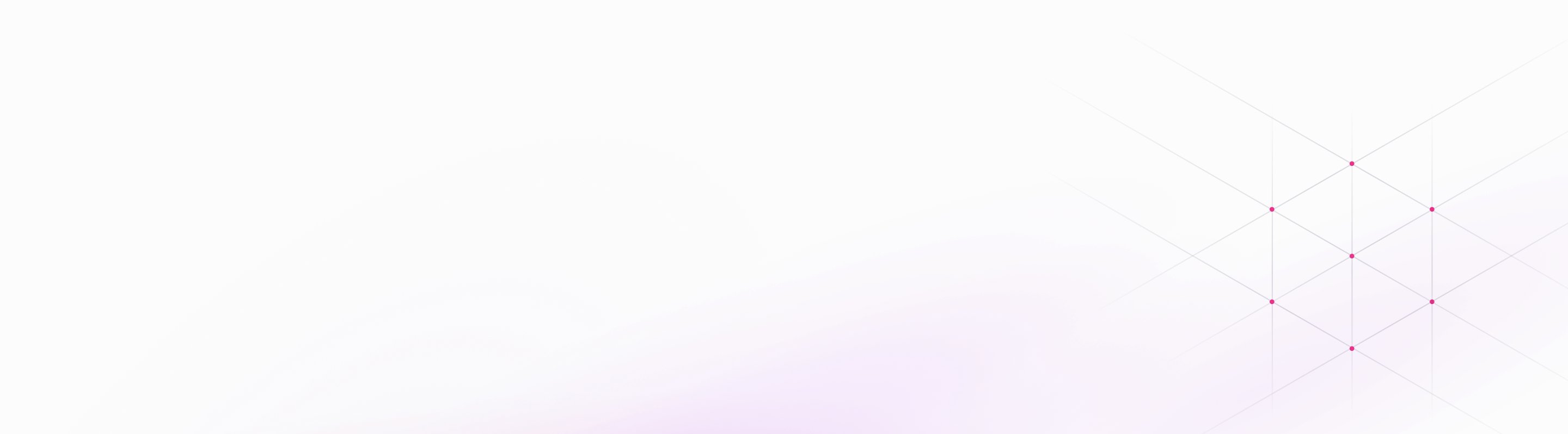
Agent Workflow Memory (AWM)
Bi-weekly AI Research Paper Readings
Stay on top of emerging trends and frameworks.