With the accelerated development of GenAI, there is a particular focus on its testing and evaluation, resulting in the release of several LLM benchmarks. Each of these benchmarks tests the LLM’s different capabilities–but are they sufficient for a complete real-world performance evaluation?
This blog will discuss some of the most popular LLM benchmarks for evaluating top models like GPT 4o, Gemma 3, or Claude. Further, we will discuss the LLMs’ use in practical scenarios and whether these benchmarks are sufficient for complex implementations like agentic systems.
Evaluation Criteria: Traditional AI vs GenAI
Traditional AI algorithms, such as those used for classification, regression, and time-series forecasting, are typically deterministic systems. This means that for a specific set of inputs, the model is expected to produce a consistent output. While the model’s predictions might deviate from the expected ground truth depending on its training, the output will remain stable when provided with the same input combination.
Standard evaluation metrics, such as accuracy, precision, and root mean square error, quantify the model’s deviation from the ground truth labels to assess its performance. These metrics offer a simple, structured, and objective measure of the AI’s effectiveness.
However, this is not the case for GenAI models. These generative models are non-deterministic, i.e., they produce a sequential output, and each element in the sequence is determined probabilistically. There are no concrete ground truths to compare the model output, making their evaluation tricky.
GenAI models are used in various scenarios, such as general conversation, logical problem-solving, and informative chatbots. Their performance is evaluated based on their ability to process the input and any available context and generate a response relevant to the scenario. Several standardized benchmarks have been established for this purpose. Each of these targets a unique aspect of the model and provides an evaluation score, which is used to judge the model’s performance.
Let’s discuss these benchmarks in detail.
40 LLM Benchmarks
Here are 40 common LLM benchmarks and the purpose of each.
Benchmark | Summary | Category |
MMLU (Massive Multitask Language Understanding) | A 57-subject multiple-choice QA test evaluating broad world knowledge and reasoning, covering topics from math and history to law. | Reasoning (Knowledge) |
ARC-AGI (Abstraction & Reasoning Corpus) | A set of abstract visual puzzles (François Chollet’s ARC) intended to measure progress toward general AI by testing pattern recognition and analogical reasoning beyond brute-force learning. | Reasoning (Analogy) |
Thematic Generalization | Tests whether an LLM can infer a hidden “theme” or rule from a few example and counterexample prompts, requiring generalization from limited data. | Reasoning (Concept Learning) |
Misguided Attention | A collection of reasoning problems designed with misdirective or irrelevant information to challenge an LLM’s ability to stay focused on the correct cues and avoid being tricked. | Reasoning (Robustness) |
WeirdML | Presents unconventional machine-learning style tasks (e.g. identifying shuffled images, odd ML problems) that demand careful, truly understanding-based reasoning from LLMs. | Reasoning (Unconventional) |
GPQA-Diamond | A graduate-level “Google-Proof” Q&A benchmark of ~200 expert-written questions in physics, biology, and chemistry – extremely challenging science problems intended to resist memorization. | Reasoning (Scientific) |
SimpleQA | A factual QA benchmark from OpenAI with short, fact-seeking questions, testing an LLM’s ability to provide accurate, concise answers to straightforward queries. | Reasoning (Factual) |
TrackingAI – IQ Bench | An IQ test for AIs using verbalized human IQ questions (e.g. Mensa puzzles) to estimate models’ cognitive ability; measures how models handle logic, pattern-matching, and problem-solving typical of IQ exams. | Reasoning (Cognitive) |
Humanity’s Last Exam (HLE) | A new ultra-hard benchmark curated by domain experts (“last exam” for AI) with rigorous questions across math, science, etc., where current top models score <10% – intended as a final obstacle indicating near-AGI when surpassed. | Reasoning (Advanced) |
MathArena | A platform using fresh math competition and Olympiad problems to rigorously assess LLMs’ mathematical reasoning; designed to avoid contamination by testing models right after contest problems are released. | Reasoning (Math) |
MGSM (Multilingual Grade School Math) | A multilingual version of grade-school math word problems (GSM8K translated into 10 languages) to test math reasoning across languages. (NOTE: this is considered saturated as many models already achieve high chain-of-thought performance) | Reasoning (Math) |
BBH (Big-Bench Hard) | A subset of 23 especially challenging tasks from the BIG-Bench suite that earlier models (like GPT-3) failed at, used to evaluate advanced compositional reasoning and out-of-distribution generalization. (Considered saturated.) | Reasoning (Mixed Hard Tasks) |
DROP (Discrete Reasoning Over Paragraphs) | A reading comprehension benchmark of 96K adversarial questions requiring discrete reasoning (e.g. arithmetic, date sorting) over text passages – models must combine reading with symbolic reasoning. | Reasoning (Reading & Math) |
Context-Arena | A leaderboard focusing on long-context understanding: it visualizes LLM performance on tasks like long document question answering and multi-turn reference resolution (such as OpenAI’s MRCR test for long-context recall). | Reasoning (Long Context) |
Fiction-Live Bench (Short Story Creative Writing) | A creative writing benchmark that asks models to write short stories incorporating ~10 specific required elements (characters, objects, themes, etc.), evaluating how well an LLM maintains narrative coherence while obeying content constraints. | Longform Writing (Creative) |
AidanBench | An open-ended idea generation benchmark where models answer creative questions with as many unique, coherent ideas as possible – penalizing mode collapse and repetitive answers, with effectively no ceiling on the score for truly novel outputs. | Creative Thinking |
EQ-Bench (Emotional Intelligence Benchmark) | Evaluates an LLM’s grasp of emotional and social reasoning through tasks like empathetic dialogue or creative writing with emotional nuance, scored by an LLM judge on facets of emotional intelligence. | Longform Writing (Emotional IQ) |
HumanEval | OpenAI’s coding benchmark of 164 hand-written Python problems where the model must produce correct code for a given specification, used to measure functional correctness in basic programming. | Coding |
Aider Polyglot Coding | A code-editing benchmark from Aider: tasks 225 coding challenges from Exercism across C++, Go, Java, JavaScript, Python, and Rust, measuring an LLM’s ability to follow instructions to modify or write code in multiple languages. | Coding (Multilingual) |
BigCodeBench | A large benchmark of 1,140 diverse, realistic programming tasks (with complex function calls and specs) for evaluating true coding capabilities beyond simple algorithmic problems. | Coding |
WebDev Arena | An arena-style coding challenge where two LLMs compete head-to-head to build a functional web application from the same prompt, allowing evaluation of practical web development skills via human pairwise comparisons. | Coding (Web Dev) |
SciCode | A research-oriented coding benchmark with 338 code problems drawn from scientific domains (math, physics, chemistry, biology) – it tests if models can write code to solve challenging science problems at a PhD level. | Coding (Scientific) |
METR (Long Tasks) | An evaluation framework proposing to measure AI performance by task length: it examines the longest, most complex tasks an AI agent can complete autonomously, as a proxy for general capability growth. | Agentic Behavior (Long-Horizon) |
RE-Bench (Research Engineering) | A benchmark from METR that pits frontier AI agents against human ML engineers on complex ML research & engineering tasks (like reproducing experiments), gauging how close AI is to automating ML R&D work. | Agentic Behavior (R&D Tasks) |
PaperBench | OpenAI’s benchmark evaluating if AI agents can replicate SOTA AI research – agents are given recent ML papers (e.g. ICML 2024) and tasked to reimplement and reproduce the results from scratch, testing planning, coding, and experiment execution. |
Agentic Behavior (Research Automation)
|
SWE-Lancer | A benchmark of 1,403 real freelance software engineering tasks (from Upwork, worth $1M total) to assess whether advanced LLMs can complete end-to-end coding jobs – mapping AI performance directly to potential earnings. |
Agentic Behavior (Real-World Coding)
|
MLE-Bench | OpenAI’s Machine Learning Engineer benchmark with 75 real-world ML tasks (e.g. Kaggle competitions) – it evaluates how well an AI agent can handle the end-to-end workflow of ML problems, including data handling, training, and analysis. | Agentic Behavior (AutoML) |
SWE-Bench | A Princeton/OpenAI benchmark of 2,294 GitHub issues (with associated codebases) – models must act as software agents that read a repo, then write patches to resolve the issue, passing all tests; it closely mirrors real developer workflows. |
Agentic Behavior (Code Maintenance)
|
Tau-Bench (Tool-Agent-User) | Sierra AI’s benchmark of interactive agents in realistic scenarios – the agent converses with a simulated user and uses tools to accomplish tasks in domains like retail (cancel orders, etc.) or airlines, testing multi-turn tool use and dynamic planning. | Agentic Behavior (Tool Use) |
XLANG Agent | An open framework and leaderboard (HKU) for multi-lingual agents – it evaluates agents’ ability to perform tasks involving multiple languages, reflecting an agent’s versatility and reasoning across language barriers. |
Agentic Behavior (Multilingual Agents)
|
Balrog-AI | A benchmark for agentic reasoning in games: tasks LLMs (and VLMs) with playing a text-based adventure or completing long-horizon game objectives, evaluating planning, memory, and decision-making in an interactive environment. | Agentic Behavior (Game) |
Snake-Bench | An LLM-as-Snake-Player challenge where models control a snake in a simulated Snake game; multiple LLM “snakes” compete, testing the model’s ability to strategize and react in a turn-based environment with long-term consequences. | Agentic Behavior (Game) |
SmolAgents LLM | A HuggingFace leaderboard that evaluates small-scale autonomous agent tasks (a mini subset of the GAIA agent benchmark and some math tasks) – ranking how both open-source and closed models perform when deployed as minimalistic agents. | Agentic Behavior (Agents) |
MMMU (Massive Multi-discipline Multimodal Understanding) | A comprehensive multimodal benchmark with college-level problems that include both text and images (diagrams, charts, etc.), requiring models to integrate visual information with advanced subject knowledge and reasoning. | Multimodal (Reasoning) |
MC-Bench (Minecraft Benchmark) | An interactive benchmark where LLMs generate Minecraft builds or solutions that are evaluated via human comparisons (like a Minecraft Arena); it tests spatial reasoning and creativity in a visual sandbox, making evaluation more dynamic and open-ended. | Multimodal (Interactive) |
SEAL by Scale (Multi-Challenge Leaderboard) | Scale AI’s multi-challenge evaluation leaderboard that aggregates a wide range of tasks into one ranking – providing a holistic comparison of models across diverse challenges (the “MultiChallenge” track showcases overall capability). | Meta-Benchmarking (Multi-Task) |
LMArena (Chatbot Arena) | A crowd-sourced Elo-style leaderboard where models duel in pairwise chat conversations (judged by users); it reveals general quality/preferences by having models compete in open-ended dialogue. | Meta-Benchmarking (Human Pref) |
LiveBench | An evergreen evaluation suite updated monthly with fresh, contamination-free test data across 18 tasks (math, coding, reasoning, language, instruction following, data analysis). It provides an up-to-date benchmark to track model progress over time. | Meta-Benchmarking (Multi-Task) |
OpenCompass | An open-source LLM evaluation platform supporting 100+ datasets. It serves as a unified framework and leaderboard to benchmark a wide range of models (GPT-4, Llama, Mistral, etc.) on many tasks, enabling apples-to-apples model comparisons. | Meta-Benchmarking (Platform) |
Dubesor LLM | A personal but extensive benchmark aggregator (named “rosebud” backwards): one individual’s ongoing comparison of various models across dozens of custom tasks, combined into a single weighted score for each model. | Meta-Benchmarking (Aggregator) |
Understanding LLM Benchmarks
To better understand some of these benchmarks, below is more detail on several of the most popular ones used for LLM evaluation.
General Knowledge & Language Understanding Benchmarks
Common benchmarks designed to test a model’s natural language understanding include:
1. MMLU Benchmark
The Massive Multi-task Language Understanding (MMLU) benchmark is a general-purpose benchmark designed to evaluate the model against diverse subjects. It contains multiple-choice questions covering 57 subjects, including STEM, social sciences, humanities, and more. The difficulty of the question ranges from elementary to advanced professional.
Here is an example question from the dataset related to business ethics:
_______ such as bitcoin are becoming increasingly mainstream and have a whole host of associated ethical implications, for example, they are______ and more ______. However, they have also been used to engage in _______.
A. Cryptocurrencies, Expensive, Secure, Financial Crime
B. Traditional currency, Cheap, Unsecure, Charitable giving
C. Cryptocurrencies, Cheap, Secure, Financial crime
D. Traditional currency, Expensive, Unsecure, Charitable giving
NOTE: MMLU is widely considered saturated and is not usually an appropriate benchmark for comparisons of today’s models. However it is still it is still worth being familiar with given its common and historical usage.
2. AI2 Reasoning Challenge
The AI2 Reasoning Challenge (ARC) is a collection of 7787 grade-school science questions. The dataset is divided into an easy set and a challenge set, where the challenge set contains questions answered incorrectly by both a retrieval-based algorithm and a word occurrence algorithm.
Here is an example question from the dataset:
Q: George wants to warm his hands quickly by rubbing them. Which skin surface will produce the most heat?
A. Dry palms
B. Wet palms
C. Palms covered with oil
D. Palms covered with lotion
3. SuperGLUE
SuperGLUE is an advanced version of the original General Language Understanding (GLU) benchmark. It consists of 8 language understanding tasks. SuperGLUE includes various tasks like reading comprehension, textual entailment, question answering, and pronoun resolution, making it a more comprehensive benchmark than the original GLUE.
A sample task from the dataset:
Premise: | The dog chased the cat. |
Hypothesis: | The cat was running from the dog. |
Label: | Entailment |
Coding Benchmarks
While there are a variety of code benchmarks, several stand out for their popularity.
4. HumanEval
The hand-written evaluation benchmark is a set of programming challenges designed to test a model’s coding capabilities. It was first introduced in “Evaluating Large Language Model Trained on Code” and comprises 164 hand-written programming challenges.
The challenges are hand-written since most LLMs are already trained on data sourced from GitHub repositories. Each problem includes a function signature, docstring, body, and several unit tests, averaging 7.7 tests per problem.
Here is a sample problem from the dataset:
def solution(lst):
"""Given a non-empty list of integers, return the sum of all of the odd elements
that are in even positions.
Examples
solution([5, 8, 7, 1])=12
solution([3, 3, 3,3, 3]) =9
solution([30, 13, 24, 321]) =0
"""
LLMs output: return sum(lst[i] for i in range(0,len(lst)) if i % 2 == 0 and lst[i] % 2 == 1)
5. CodeXGLUE
The CodeXGLUE benchmark dataset is built to test LLMs’ code understanding and generation. It includes a collection of 10 tasks across 14 datasets and a platform for model evaluation and comparison. The tasks can be divided into 4 higher categories:
- Code-code: This includes code translation, completion, debugging, and repair.
- Text-code: This includes code generation from natural language descriptions and analyzing the semantics between code and a text description.
- Code-text: This includes code summarization and explanation.
- Text-text: This includes translating code documentation from one natural language to another.
Here is an example from the code translation task:
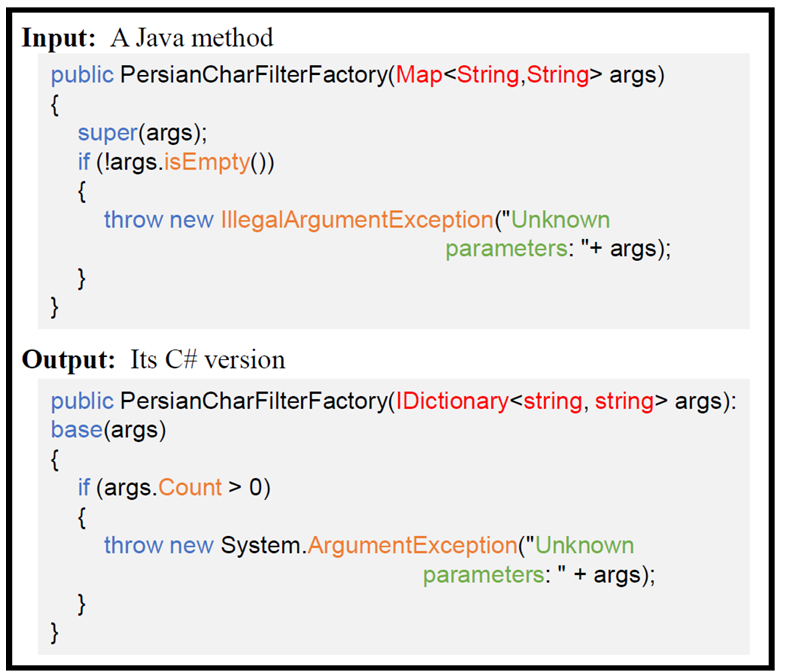
Code translation task from CodeXGLUE benchmark – Source
6. SWE-Bench
The SWE-Bench benchmark consists of 2294 real-world software engineering problems pulled from GitHub. The tasks involve understanding comments from GitHub pull requests and making relevant changes to the codebase. The LLM is tasked with identifying and solving the issue and running tests to ensure everything runs fine.
Reasoning Benchmarks
Here are some benchmarks that test the model’s ability to conduct logical reasoning to reach a conclusion.
7. GSM8k
GSM8k consists of 8.5k grade-school-level and linguistically diverse mathematics problems. These problems are laid out in natural language, making them challenging for AI models to understand. It tests the LLMs’ ability to break down the natural language problem, form a chain-of-thought, and reach the solution.
Here is an example problem from the dataset:
Problem: | Beth bakes 4, 2 dozen batches of cookies in a week. If these cookies are shared amongst 16 people equally, how many cookies does each person consume? |
Solution: | Beth bakes 4 * 2 = 8 dozen cookies. There are 12 cookies in a dozen, so she makes 12 * 8 = 96 cookies. She splits the 96 cookies equally amongst 16 people, so each person eats 96 / 16 = 6 cookies. |
Final Answer: | 6 |
8. Counterfactual Reasoning Assessment (CRASS)
CRASS provides a novel test scheme utilizing so-called counterfactual conditionals and, more precisely, questionized counterfactual conditionals. A counterfactual is a statement that presents a scenario that might have happened but did not. These are also commonly known as what-if scenarios. The CRASS benchmarks contain multiple such scenarios with alternative realities and test the model’s understanding against these.
A sample scenario from the dataset is:
A woman sees a fire. What would have happened if the woman had fed the fire?
- The fire would have become larger.
- The fire would have become smaller.
- That is not possible.
9. Big-Bench Hard (BBH)
The original Big-Bench benchmark consists of 200 tasks covering domains like arithmetic and logical reasoning, commonsense knowledge, and coding. However, most modern LLMs outperform human raters for many of these tasks. The Big-Bench Hard is a subset of the original, containing 23 challenging tasks for which no LLM outperformed human raters. These tasks challenge the LLMs’ reasoning capabilities and the development of a chain-of-thought.
A sample task from the benchmark is:
Question: | Today, Hannah went to the soccer field. Between what times could they have gone? We know that: Hannah woke up at 5 am. [ … ] The soccer field was closed after 6 pm. [ … ] |
Options: | A. 3 pm to 5 pm B. 5 pm to 6 pm C. 11 am to 1 pm D. 1 pm to 3 pm |
Are LLM Benchmarks Sufficient?
LLM benchmarks are a great way to evaluate these models’ performance in real-world scenarios, but the real question remains: Are they sufficient for a generic evaluation? The benchmarks we have discussed above are just a small subset, and there are several more frameworks for numerous other tasks.
Moreover, no single LLM excels in each evaluation since each model is trained for a different purpose. For example, the recently released GPT-4.5 surpasses the older o3-mini in basic language understanding but loses in complex reasoning tasks as it is not a Chain-of-Thought (CoT) reasoning model.
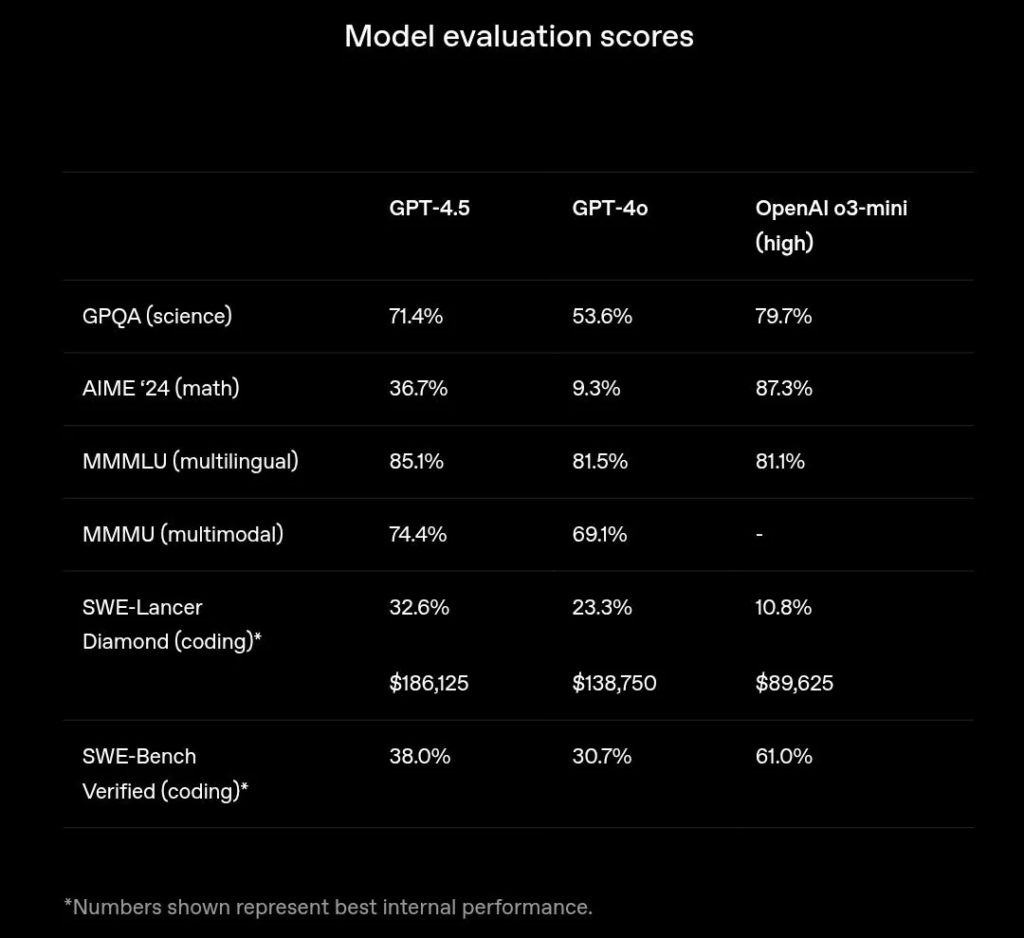
Evaluation scores for GPT-4.5 compared with GPT-4o and o3-mini – Source
So, while each benchmark quantifies the LLM’s performance for a few particular scenarios, these numbers do not portray an overall picture. A single LLM may perform differently on different benchmarks, even within a single domain, since each has slightly different tasks.
It proves that most benchmarks are designed for a specific and rather lenient evaluation. A great example is the Humanity’s Last Exam (HLE) benchmark, which is one of the rare evaluation frameworks designed to be a single unit of measure of the model’s performance. It consists of 2700 extremely challenging and multi-modal tasks across several academic domains. The results for HLE against state-of-the-art models prove how much current LLMs are still lacking and how other benchmarks are insufficient for modern-day evaluation.
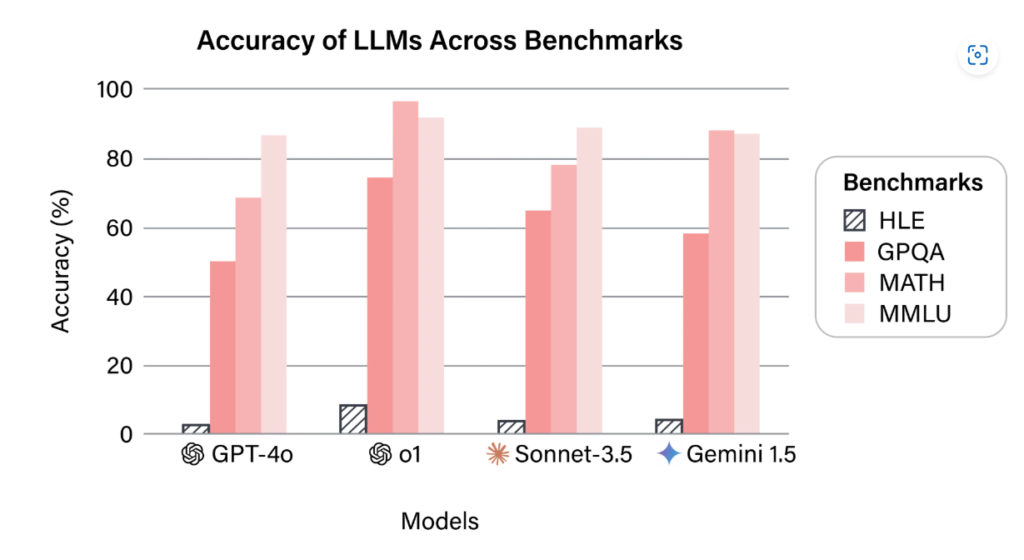
Benchmarks demonstrating LLMs poor performance on the HLE benchmark – Source
Another important factor to consider here is that modern systems are now moving towards agentic implementations. Conventional benchmarks may evaluate the model’s generative response but do not assess its performance with an automated agentic system.
Agentic Evaluation: Going Beyond LLMs
An agentic system goes beyond language understanding and data generation. It involves reading real-time data streams, interacting with the environment, and breaking down tasks to complete a set objective. AI agents are gaining popularity, and several interesting and practical use cases have been found in industries like customer support, e-commerce, and finance. They have also been deployed in some unusual but fun situations, such as Anthropics Claude Sonnet 3.7 Sonnet playing Pokemon Red on the original Game Boy.
Conventional benchmark scores do not represent performance in real-world actionable scenarios. These practical systems require specialized benchmarks like AgentBench and t-bench to better judge the agent’s capabilities. These benchmarks test the LLMs’ interaction with modules like databases and knowledge graphs and further evaluate them on multiple platforms and operating systems. Moreover, agentic systems also need to be judged on the time it takes them to complete a certain task compared to humans. Studies suggest that while the task completion time horizon is growing exponentially, agentic systems are presently behind human workers and may take some time to fully automate everyday tasks.
Final Thoughts
The age of GenAI is here, and it’s here to stay. Generative models are being integrated into everyday workflows, automating mundane tasks and improving work efficiency. However, as this adoption increases, it is vital to evaluate these LLM-based systems and how they fare against challenging real-world scenarios.
Several benchmarks have been created for LLM evaluation, and each tests the model in different scenarios. Some evaluate the model’s performance on logical reasoning, while others judge it on its ability to solve programming problems and generate code. However, as newer and smarter models are released, even the most popular benchmarks are proving insufficient in providing a complete evaluation. Benchmarks like the HLE prove that even state-of-the-art models can be found lacking in challenging scenarios.
Moreover, as agentic AI gains traction, we need newer, more robust ways to evaluate end-to-end systems. Conventional benchmarks do not evaluate a model’s understanding of its surroundings or its ability to complete a set objective.
As GenAI progresses, evaluation metrics must evolve to meet the demanding practical requirements. Newer standards must be set to ensure the safe and smooth adoption of AI.