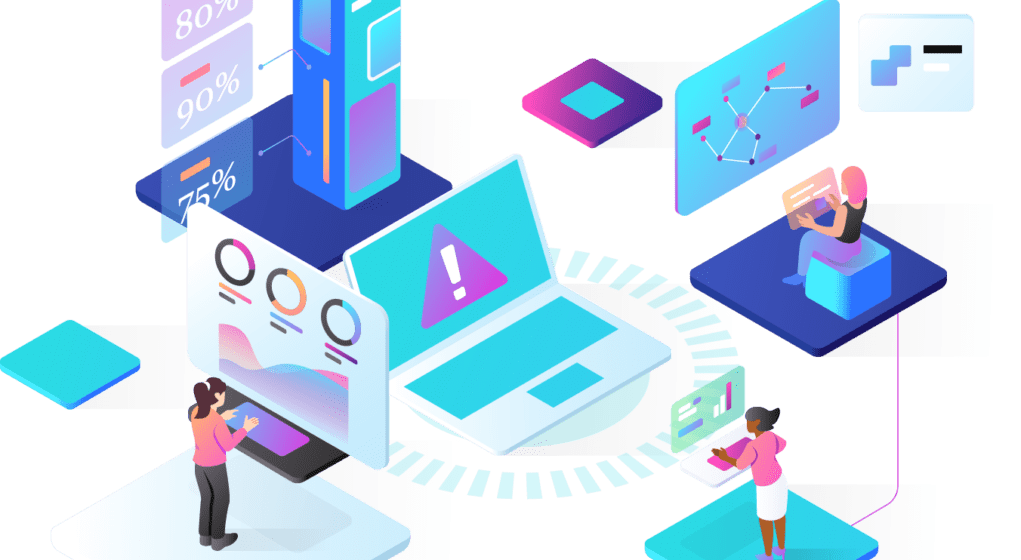
ML Infrastructure Tools — ML Observability
The ML Observability platform allows teams to analyze model degradation and to root cause any issues that arise. This ability to diagnose the root cause of a model’s issues, by connecting points across validation and production, is what differentiates model observability from traditional model monitoring. While model monitoring consists of setting up alerts on key model performance metrics such as accuracy, or drift, model observability implies a higher objective of getting to the bottom of any regressions in performance or anomalous behavior. We are interested in the why. Monitoring is interested in only aggregates and alerts. Observability is interested in what we can infer from the model’s predictions, explainability insights, the production feature data, and the training data, to understand the cause behind model actions and build workflows to improve.
ML Observability backed by an Evaluation Store:
- Move seamlessly between production, training, and validation dataset environments
- Natively support model evaluation analysis by environment
- Designed to analyze performance Facets/Slices of predictions
- Explainability attribution designed for troubleshooting and regulatory analysis
- Performance analysis with ground truth — Accuracy, F1, MAE
- Proxy performance without ground truth — prediction drift
- Distribution drift analysis between data sets and environments
- Designed to answer the why behind performance changes
- Integrated validation
- Architected to iterate and improve
Why ML Observability matters
Our team has personally worked on models deployed in pricing, forecasting, marketing, credit and delivery time estimates, to name a few. In all cases there is a common story line; we would build a model, deploy it and it would work great in one city and not in another. It would work great across a set of neighborhoods/customers/types of products and poorly across others. It would be great in the average case but horrible on the tail end of predictions. Or it would work great on initial launch and then the model would slowly degrade. Other times you would have an instantaneous change caused by an upstream data pipeline mistake, that would slowly poison the training data. In all these cases it was clear there was a missing foundational piece of ML infrastructure to help make models work as teams deployed them to production.
In another common use of Machine Learning, such as fraud model analysis, understanding the why behind performance changes is extremely important. In fraud there is a constant landscape of changing behavior based on adversarial actions of bad actors. These bad actors create pockets of performance degradation that might throw a red or green light in a monitoring system. But the causal connection, “the why”, is typically the insight for stopping the fraud scheme.
In companies that build a model per customer, trained on customer specific data, every model is trained differently. Teams can be inundated with models showing data/model drift but it can be hard to show if that drift is a problem. How do you bottom out issues at scale and how does your ML team scale as customers grow? Teams want to quickly know where issues are occurring at scale, compare production swiftly to validation and clear the issues quickly with confidence.
Without the tools to reason about mistakes a model is making in the wild, teams are investing a massive amount of money in the data science laboratory but essentially flying blind in the real world.
The following blogs will highlight how teams can deliver models with confidence, continually improve and gain a competitive ML advantage. We will take a deeper dive into ML Observability and ML monitoring.
We hope you enjoyed the ML Infrastructure tools series. Up next, we will be diving deeper into ML Observability. There are so many under discussed and extremely important topics on operationalizing AI that we will be diving into!
If this blog post caught your attention and you’re eager to learn more, follow us on Twitter and Medium! If you’d like to hear more about what we’re doing at Arize AI, reach out to us at contacts@arize.com. If you’re interested in joining a fun, rockstar engineering crew to help make models successful in production, reach out to us at jobs@arize.com!