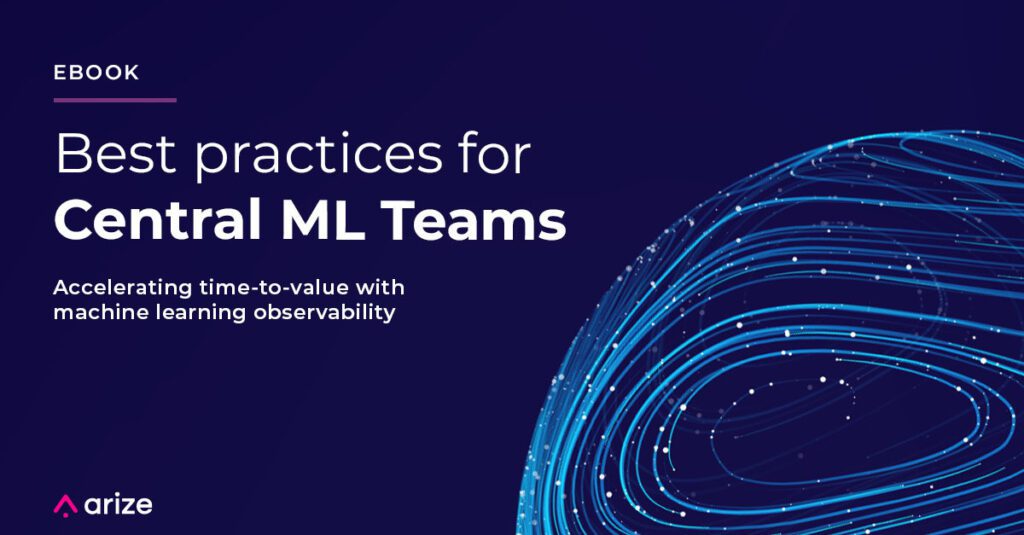
Central machine learning (ML) engineering teams play a pivotal role in managing the critical ML infrastructure for a company to successfully get models from research to production. ML observability is a key part of that toolchain, enabling teams to automatically surface and resolve model performance problems before they negatively impact business results.
In order to see these benefits, however, central ML teams must first integrate ML observability into their existing infrastructure and onboard and train data scientists to use it. While this might sound easy, it is often difficult in practice. This best practices guide is based on Arize’s experience working with a diverse array of central ML teams.
In this paper, you will learn:
- How to integrate and work with an ML observability provider
- How to introduce an ML observability solution to data scientists and business teams, drive adoption, and measure success
- How to navigate security, deployment, and update protocols