Co-authored by Ali Arsanjani, Director of Applied AI Engineering at Google Cloud
1. Introduction: The Dawn of the Agentic Era
We have entered into a new era of AI innovation and adoption, driven by three transformative trends: Multimodality, Multi-agent Systems,and Agentic Workflows. These trends have converged to make AI agents a game-changing technology for organizations across industries. From customer service to data analysis, content creation to process automation, AI agents are increasingly taking on more and more complex tasks with remarkable effectiveness.
However, as organizations move from experimentation to production, they face significant challenges:
- Complexity: Building sophisticated agents requires orchestrating multiple components, including LLMs, tools, and complex workflows
- Deployment: Taking agents to production introduces infrastructure, scaling, and reliability challenges
- Observability: The “black box” nature of LLMs makes it difficult to understand agent behavior and diagnose issues
- Performance: Without proper evaluation, it’s nearly impossible to identify optimization opportunities and measure improvements
Google’s Agent Developer Kit and Arize together provide a comprehensive solution to these challenges. Google’s framework offers a flexible, production-ready foundation for building and deploying agents at scale, regardless of the orchestration framework used. Arize complements this with specialized observability tools designed specifically for modern AI systems, providing unprecedented visibility into agent behavior and performance and the tools to fix issues.
In this blog, we’ll explore how these powerful platforms work together to enable robust, observable AI agents.
2. Google Agent Developer Kit and Arize: A Powerful Combination
As AI agents move from experimental projects to business-critical applications, organizations need solutions that address both development and operational concerns. Google’s Agent Developer Kit and Arize together provide a comprehensive platform for the entire agent lifecycle, from initial development through production deployment and continuous improvement.
Google Agent: Build Once, Deploy Anywhere
The Google Agent Developer Kit provides a flexible foundation for agent development and deployment:
- Framework Agnostic: Supports LangGraph, LangChain, CrewAI, AG2, or custom implementations
- Multi-Agent Support: Design systems with specialized agents working together
- Vertex AI Agent Engine: Managed runtime for deployment, scaling, and operations
- Tool Integration: Connect to APIs, databases, and enterprise systems
- Enterprise-Grade Infrastructure: Google Cloud security, compliance, and reliability
This approach lets developers focus on agent logic while simplifying deployment and iteration. The clean separation between agent definition and deployment infrastructure also makes it easier to iterate on agent designs without disrupting production systems.
Arize: Unified AI Observability & Evaluation Platform
Arize helps accelerate AI app and agent development, then perfect them in production:
- OpenTelemetry Tracing: End-to-end visibility with seamless OpenTelemetry instrumentation, providing detailed insights into prompts, variables, tool calls, and agent interactions
- Evaluation: Automated assessments throughout development, with LLM-as-Judge insights and production-scale evaluation
- Annotations: Combine human expertise only when needed with automation to generate quality labels and identify edge cases
- Prompt Playground IDE: Developer tools for designing, testing, and evaluating prompts with built-in feedback loops
- Datasets and Experiments: Create curated datasets of failure patterns or golden datasets from production data. Run experiments to deliver reliable improvements.
- Monitoring: Real-time monitoring, visualizations, anomaly detection, customizable metrics, and integrated alerts
Together, these platforms address the complete AI agent lifecycle:
- Development: Framework flexibility, evaluation driven development
- Deployment: Managed infrastructure handling scaling and security
- Observability: Full visibility, understanding of every stage of agent flow
- Improvement: Data-driven optimization through systematic evaluation
- Enterprise Readiness: Security and compliance plus production monitoring
This combination empowers organizations to confidently deploy AI agents for business-critical applications on the Vertex AI Agent Engine with reliable monitoring, diagnostics, and continuous improvement capabilities. In the next section, we’ll set up our development environment to start building with these powerful tools.
3. Tutorial: Set Up Your Environment
The rest of this blog is based on the accompanying tutorial notebook.
Before building and deploying an observable AI agent, you’ll need to configure your development environment. This section walks through the essential setup process for Google Cloud.
Arize AX Account Sign up and Google Project Setup
Before using this tutorial, you will need:
A Google Cloud project with Vertex AI enabled and a storage bucket created.
An Arize AX account (sign up for free).
Note: Arize also offers a lighter-weight, open-source option called Phoenix. If you’d prefer to use that option instead, you can follow this tutorial.
Install Dependencies
%pip install --upgrade --user --quiet \
"google-cloud-aiplatform[agent_engines,langchain]==1.87.0" \
cloudpickle==3.0.0 \
pydantic==2.11.2 \
langgraph==0.2.76 \
httpx \
"arize-otel>=0.7.0" \
"openinference-instrumentation-langchain>=0.1.4"
Google Cloud Configuration
PROJECT_ID = "your-project-id" # Replace with your GCP project ID
LOCATION = "us-central1" # Choose a supported region
STAGING_BUCKET = "gs://your-storage-bucket" # For storing agent artifacts
import vertexai
vertexai.init(project=PROJECT_ID, location=LOCATION, staging_bucket=STAGING_BUCKET)
These settings establish the connection to your Google Cloud resources and specify where deployment artifacts will be stored.
With our environment configured, we’re ready to build our agent in the next section. We’ll create a simple but practical example that demonstrates the key capabilities of both Google’s Agent Framework and Arize’s observability platform.
4. Build and Test a Simple Agent
In this section, we’ll build a straightforward but functional agent using LangGraph and prepare it for deployment on Vertex AI Agent Engine. Remember that while we’re using LangGraph for this example, Google’s Agent Framework supports multiple orchestration frameworks and custom setup.
Defining Agent Tools
First, let’s define a simple tool for our agent to use. For this example, we’ll create a product lookup tool that returns details about different products:
def get_product_details(product_name: str):
"""Gathers basic details about a product."""
details = {
"smartphone": "A cutting-edge smartphone with advanced camera features and lightning-fast processing.",
"coffee": "A rich, aromatic blend of ethically sourced coffee beans.",
"shoes": "High-performance running shoes designed for comfort, support, and speed.",
"headphones": "Wireless headphones with advanced noise cancellation technology for immersive audio.",
"speaker": "A voice-controlled smart speaker that plays music, sets alarms, and controls smart home devices.",
}
return details.get(product_name, "Product details not found.")
This simple function serves as an API that our agent can call to retrieve product information. In a real-world scenario, this might connect to a product database, e-commerce system, or other data source.
Creating a Router
Next, we’ll define a router that controls the flow of the conversation, determining when to use our product information tool:
from typing import Literal
def router(state: list):
"""Initiates product details retrieval if the user asks for a product."""
# Get the tool_calls from the last message in the conversation history
tool_calls = state[-1].tool_calls
# If there are any tool_calls
if len(tool_calls):
# Return the name of the tool to be called
return "get_product_details"
else:
# End the conversation flow
return "__end__"
This router examines each message to determine if the agent should use the product details tool or simply end the current processing flow.
Build the Agent Application
Now we’ll create our agent application class that brings everything together. Agent templates in Vertex AI Agent Engine are defined as Python classes.
Set your Arize space id, api key and project name variables accordingly in the tracer provider which will send traces to your Arize account.
from langchain_core.messages import BaseMessage, HumanMessage
from langchain_google_vertexai import ChatVertexAI
from langgraph.graph import END, MessageGraph
from langgraph.prebuilt import ToolNode
class SimpleLangGraphApp:
def __init__(self, project: str, location: str) -> None:
self.project_id = project
self.location = location
# The set_up method is used to define application initialization logic
def set_up(self) -> None:
# Set up Arize instrumentation with OpenTelemetry
from arize.otel import register
tracer_provider = register(
space_id = "", # in Arize settings page
api_key = "", # in Arize settings page
project_name = "", # name this to whatever you wish
)
from openinference.instrumentation.langchain import LangChainInstrumentor
LangChainInstrumentor().instrument(tracer_provider=tracer_provider)
# Create the Vertex AI model
model = ChatVertexAI(model="gemini-2.0-flash")
# Build the agent graph
builder = MessageGraph()
# Add the model with tool binding
model_with_tools = model.bind_tools([get_product_details])
builder.add_node("tools", model_with_tools)
# Add the tool node
tool_node = ToolNode([get_product_details])
builder.add_node("get_product_details", tool_node)
builder.add_edge("get_product_details", END)
# Set entry point and routing
builder.set_entry_point("tools")
builder.add_conditional_edges("tools", router)
# Compile the runnable graph
self.runnable = builder.compile()
# The query method will be used to send inputs to the agent
def query(self, message: str):
"""Query the application.
Args:
message: The user message.
Returns:
str: The LLM response.
"""
chat_history = self.runnable.invoke(HumanMessage(message))
return chat_history[-1].content
This class:
- Initializes with Google Cloud project information
- Sets up Arize instrumentation for observability
- Creates a LangGraph agent with our product tool
- Defines the flow using our router
- Provides a simple query interface for interacting with the agent
Testing Locally
Before deploying to Vertex AI Agent Engine, let’s test our agent locally:
agent = SimpleLangGraphApp(project=PROJECT_ID, location=LOCATION)
agent.set_up()
# Test with a product query
response = agent.query(message="Get product details for headphones")
print(response)
This should return information about the headphones from our product database. We can try a few more queries to ensure the agent is functioning correctly:
print(agent.query(message="Get product details for coffee"))
print(agent.query(message="Get product details for smartphone"))
We can also test how the agent handles requests outside its capabilities:
print(agent.query(message="Tell me about the weather"))
This should result in a message indicating that the agent cannot fulfill this request using its available tools.
Key Takeaways from the Agent Design
This simple agent demonstrates several important principles:
- Separation of Concerns: The tool (product details), routing logic, and agent framework are cleanly separated.
- Declarative Flow Definition: The agent’s behavior is defined as a graph of nodes and edges rather than complex if/else logic.
- Tool Integration: External functions are seamlessly integrated as tools that the agent can use when appropriate.
- Observability by Design: The LangChain auto-instrumentator from Arize (LangChainInstrumentor().instrument()) makes it incredibly easy to add comprehensive observability with just a few lines of code, automatically capturing all interactions between components without requiring manual instrumentation throughout the codebase.
While this example is intentionally simple, the same patterns can be applied to more complex agents with multiple tools, sophisticated routing logic, and advanced capabilities.
In the next section, we’ll deploy this agent to Vertex AI Agent Engine for production use.
5. Deploy Agent to Vertex AI Agent Engine
Once you’ve built and tested your agent locally, the next step is deploying it to Vertex AI Agent Engine for production use. This managed service handles all the infrastructure complexity, allowing you to focus on your agent’s capabilities rather than operational concerns.
Preparing for Deployment
Before deploying, ensure your agent implementation is complete and working as expected in local testing. The deployment process will package your code, dependencies, and configuration into a format that can be executed on Google Cloud.
Deploying the Agent
Deploying your agent to Vertex AI Agent Engine is remarkably straightforward. Using the Vertex AI SDK, you can deploy your agent with just a few lines of code:
from vertexai import agent_engines
# Deploy the agent to Vertex AI Agent Engine
remote_agent = agent_engines.create(
SimpleLangGraphApp(project=PROJECT_ID, location=LOCATION),
requirements=[
"google-cloud-aiplatform[agent_engines,langchain]==1.87.0",
"cloudpickle==3.0.0",
"pydantic==2.11.2",
"langgraph==0.2.76",
"httpx",
"arize-otel>=0.7.0",
"openinference-instrumentation-langchain>=0.1.4"
],
display_name="Agent Engine with LangGraph",
description="This is a sample custom application in Agent Engine that uses LangGraph",
extra_packages=[],
)
print(f"Agent deployed: {remote_agent.resource_name}")
This deployment process:
- Packages your agent class (SimpleLangGraphApp)
- Specifies all required Python dependencies
- Configures a display name and description
- Creates a deployable instance on Vertex AI Agent Engine
The deployment process may take a few minutes as Vertex AI provisions the necessary resources and configures your agent for serving.
6. Test the Deployed Agent
Once deployed, you can interact with your agent using the same interface as in local testing:
# Test the deployed agent
response = remote_agent.query(message="Get product details for headphones")
print(response)
# Try another query
response = remote_agent.query(message="Get product details for coffee")
print(response)
# Test how it handles requests outside its capabilities
response = remote_agent.query(message="Tell me about the weather")
print(response)
The deployed agent should respond just as it did during local testing, but now it’s running on Google’s managed infrastructure.
7. View Traces in Arize AX
With the Arize instrumentation we configured during agent development, trace data will automatically flow to your Arize space. To access this data:
- Log in to your Arize account
- Navigate to the space configured in your agent’s setup method
- Select the “Tracing” tab to view agent interactions
The dashboard provides a centralized view of all agent activities, enabling you to view traces, run evaluations, monitor performance, detect and fix issues, and identify improvement opportunities.
We can inspect a trace from the testing we ran earlier. Here’s what to look for:
1. Trace Explorer
- Visualize the execution path of each query
- See the timing of each step in the process
- Identify bottlenecks or slow operations
2. LLM Call Analysis
- Review actual prompts and completions from the LLM calls
- Understand how the model interprets user requests
- Identify patterns in successful vs. problematic interactions
3. Tool Usage Patterns
- Monitor tool calling and selection activity
- Identify cases where incorrect tool was called or incorrect params were passed
- Detect when tools fail to provide useful information
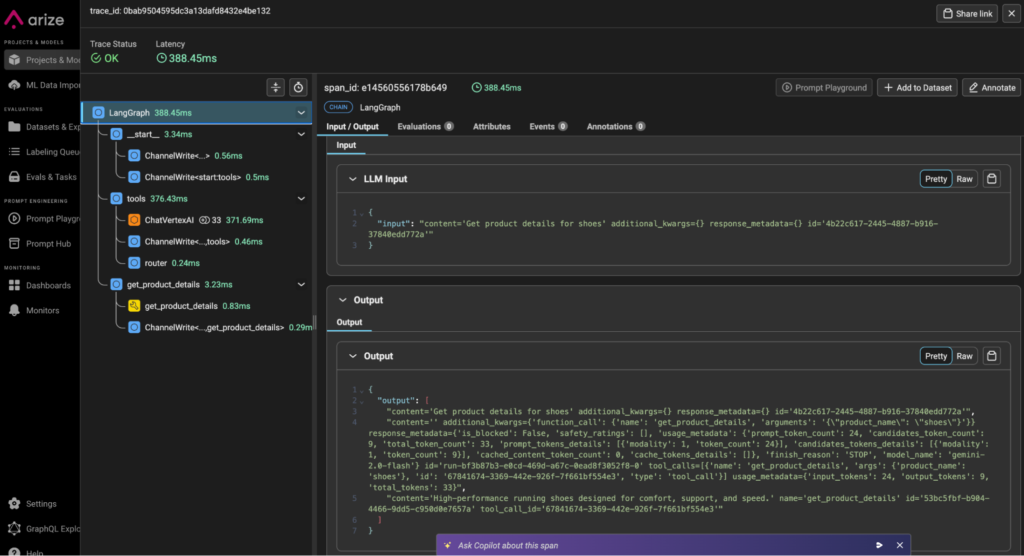
Continuing Your Journey with Arize AX
After mastering the basics of tracing and monitoring, you can leverage Arize’s comprehensive suite of AI development and optimization tools to take your agents to the next level. The platform offers a complete ecosystem for the AI application development lifecycle, enabling you to not only observe but also actively improve your agents through structured evaluation and experimentation.
- Setting up automated evaluations: Configure comprehensive evaluation suites that assess your agent against multiple dimensions including accuracy, adherence to guidelines, and response quality
- Testing and optimizing prompts: Use Arize’s prompt playground to systematically test prompt variations, measure their performance, and identify optimal configurations
- Generating curated datasets: Build high-quality datasets based on real user interactions for targeted testing and evaluation
- Running controlled experiments: Design and execute A/B tests to measure the impact of agent changes in a structured way
- Implementing custom evaluation metrics: Create domain-specific metrics that align with your business objectives and user experience goals
- Leveraging Arize’s AI Copilot: Use AI-powered assistance to analyze complex patterns in your agent’s behavior and recommend specific improvements
- Setting up continuous evaluation pipelines: Automate the evaluation process to continuously assess agent performance as you deploy new versions
8. Conclusion and Final Thoughts
Throughout this blog, we’ve explored how Google’s Agent Framework and Arize’s observability platform combine to create a powerful solution for building, deploying, and improving AI agents.
Key Takeaways
The Power of Framework-Agnostic Deployment: Google’s Agent Framework offers unprecedented flexibility, allowing you to use your preferred orchestration framework while benefiting from a consistent, enterprise-grade deployment platform.
Observability as a Superpower: Arize’s specialized AI observability platform transforms agent development from guesswork to data-driven improvement, providing deep visibility into agent behavior and performance.
The Continuous Improvement Cycle: This combination creates a powerful improvement cycle where you can deploy new agent versions, observe their behavior, identify enhancement opportunities, and implement targeted improvements with confidence.
Final Thoughts
AI agents represent a significant evolution in how we interact with technology, combining the power of large language models with the ability to take actions in the real world. As these agents become more capable and widespread, the need for robust development, deployment, and monitoring solutions becomes increasingly critical.
The combination of Google’s Agent Framework and Arize provides a comprehensive answer to this need, enabling teams to build sophisticated agents, deploy them with confidence, and continuously improve them based on data-driven insights.
By adopting this approach, you can move beyond experimental AI agents to create production-ready systems that deliver real value to users while maintaining the reliability, observability, and continuous improvement capabilities that enterprise applications demand.
We hope this guide helps you on your journey to building exceptional AI agents.
Happy building!
Further Resources
Google Cloud Resources
Arize Resources