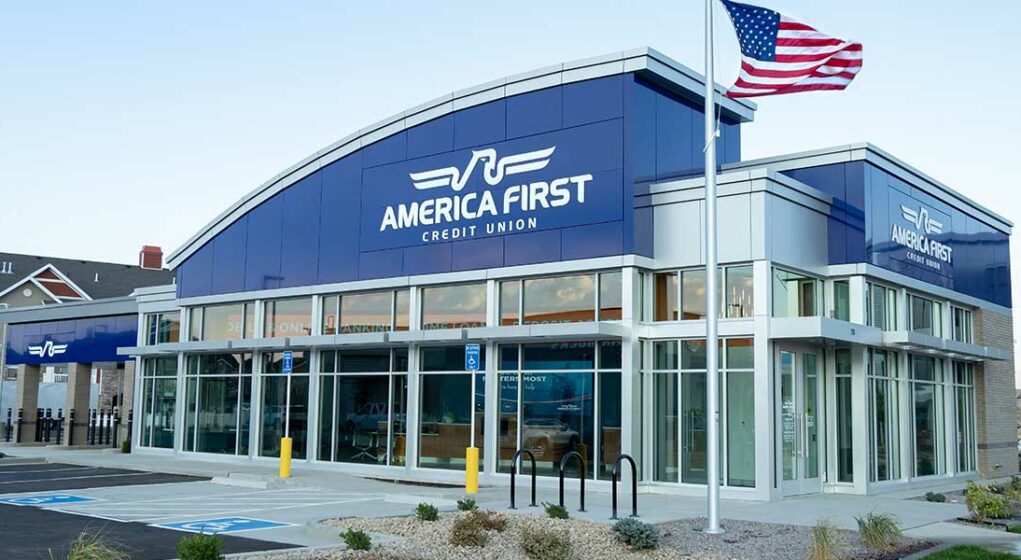
How ML Observability Helps America First Credit Union Stay a Step Ahead
Insights from Arize AI’s fireside chat with client America First Credit Union
Last month, we hosted a webinar on “Best Practices in ML Observability for Lending & Insurance” featuring a fireside chat with America First Credit Union’s Data Science Manager Richard Woolston. In the wide-ranging interview, Woolston shares his thoughts on the evolving financial services industry and how his team approaches model monitoring and ML observability. Here are some of the more pointed questions and Woolston’s answers from that session.
Arize: America First Credit Union is one of the largest credit unions in the country. How do you help the organization continue to stand out in a competitive market?
Speed. Everyone these days wants a quick response. Models are the best way to generate lending decisions faster. Another way our team stands out is in identifying segments that we may not have targeted and finding ways to capture that group. So it’s speed and ensuring we’re analyzing the portfolio as a whole as effectively as possible.
Arize: What are your biggest challenges in terms of model development and keeping an eye on model performance in production?
It’s a couple of things; the first one is delayed actuals. Often, the feedback time for amortizing loans – such as auto loans, RV loans, etc. – can be quite substantial because the tag or the label that you actually care about takes time, often years, to get. For example, did the loan pay off early, did it write off, or is it still active?
One of our main priorities is identifying proxy metrics to act as canaries, such as a high number of delinquencies or whether a member is paying down quicker than scheduled. By working those into our monitoring framework, we don’t have to wait two or three or four or five years to get a good sense of portfolio health.
Another challenge: legacy systems. We have some actuals that people update manually in spreadsheets so we work with the analysts and move those actuals into the database so that way systems can access them in a much friendlier way.
Arize: Let’s talk about proxy metrics. Is drift one that you’re looking at actively and are there other proxy metrics that are top-of-mind?
Drift is the first proxy metric that we use as a canary metric and it actually helped us very early on identify an issue where the source system was actually storing things in an incorrect fashion – and we were able to make some quick adjustments.
As we deploy a model into production, drift is the first thing we look at as predictions start to come across over the hours or days to know that things are stable. If not, we can go ahead and roll back and identify what pieces were missing and fix the offline feature store and iterate.
As I mentioned earlier, delinquency is a huge proxy metric – so did someone make their first payment and are they 45 days behind, 60 days behind and those types of things – and then of course eventually the real metrics like whether they pay it off, write-off, or whether they are still active, which can come substantially longer or later in the life cycle depending on the product.
Arize: How are you accounting for fair lending regulations and bias assessment as it relates to race, gender, sex and other protected groups?
That’s a great question and it’s always at the forefront of our minds. Obviously we don’t use any of these metrics within our models, but the nice thing about Arize is that we can submit gender and age as features for the model even though we didn’t use them in the model itself. That allows us to go ahead and double check to see breakdowns, so we’re able to watch those very specifically, and even tag them with monitors. We are working on adding what we call the ECOA feature group so that it is automatically added to every prediction for tracking and monitoring.
Arize: When monitoring your model’s health and performance, what are the specific metrics you’re looking at? Are there intermittent estimates of performance given delayed actual values?
The metrics depend on the domain, but specifically within lending we tend to focus on three key metrics: recall, precision and mean absolute error (MAE).
As loans come through our system, we withhold five percent of the responses. Those loans are then moved to manual underwriting, where an underwriter goes ahead and performs their natural operation and says this is approved, declined, or a couple of other statuses.
The ones we typically care about are approved and declined loans. We monitor those specifically using recall and precision – ensuring the model hasn’t drift in a direction different from underwriting.
Then, based upon those loans and ones we missed – so things like credit cards – and what does our limit look like compared to underwriting’s limit, which is where MAE and MSE come into play. That’s our first line, and then as we get into delinquency where we once again use precision and recall but don’t use MAE because there’s nothing really to measure against. Then with write-off, it’s just recall and precision.
Arize: Once you’re alerted of an issue, how do you troubleshoot – and how does Arize help?
At a high level we allow Arize to set up drift monitors – we tune them of course, and as mentioned we upload more features than what’s actually in the model so that we can slice it in different ways.
Once setup and an alert happens, Arize will email us which will generate a Jira ticket that says “your credit score average is out of whack in some way” and whoever is on call then goes ahead and works that issue to determine whether we need some sort of longer discussion or if it is some sort of short-term seasonality thing.
We also have great product teams that we will have a back-and-forth where we tell them that we’ve identified this problem – how do we want to work through it? Here’s the impact on the model, here are the actions – we can either retrain or we can say there is drift but it’s not a problem yet.
It’s really this interaction between the product owners and my team that is critical to any major troubleshooting because we don’t actually own any of the models – we act more as an internal consultancy at America First Credit Union. We build and deploy the models and then we work with the product owners, who are even more well-versed in the product and often drift issues require that deeper understanding for the proper context.
Arize: How do you see the future of lending continue to be shaped by ML models?
I think that access to credit becomes easier, especially for groups that are traditionally excluded – and especially as we bring in more and more and more data. I also think regulation becomes easier over time, as systems needed to support these ecosystems naturally become more self documenting.