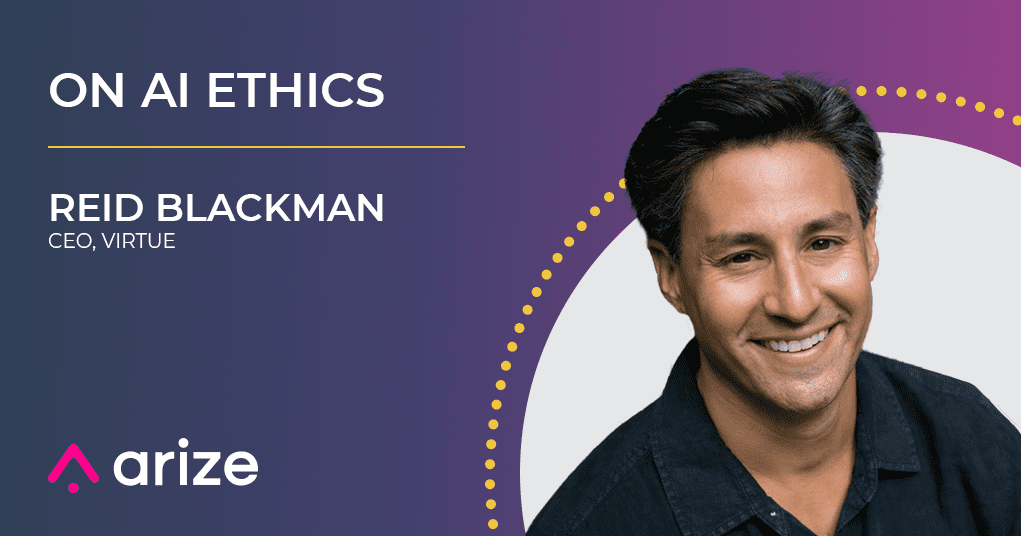
Operationalizing AI Ethics, No Longer An Option But An Imperative
A Virtual Sit Down with Reid Blackman.
As I’ve written in my “On AI Ethics,” series, machine learning models that aim to mirror and predict real-life as closely as possible are not without their challenges. Household name brands like Amazon, Apple, Facebook, Google have been accused of algorithmic bias that has negatively affected society at large.
While some organizations are investing in teams to ensure algorithmic accountability and ethics, Reid Blackman, CEO of Virtue and former professor of philosophy at Colgate University and the University of North Carolina, Chapel Hill, says most are still falling short in ensuring their products perform ethically in the real world.
“Despite reputational, regulatory, and legal risks, it’s surprising how many companies that rely on AI/ML still lack the ability to identify, evaluate, and mitigate the associated ethical risks,” says Blackman. “Teams end up either overlooking risks, scrambling to solve issues as they come up, or crossing their fingers in the hope that the problem will resolve itself.”
When we built an end-to-end ML observability and model monitoring platform–an “on the ground” solution as Blackman calls it–our goal was to help clients address these issues by better surfacing, troubleshooting, and explaining anomalies in their models.
But solutions like ours are merely tools for an organization to wield. We agree, as Blackman suggests, that the answer to using data and developing AI products without falling into ethical pitfalls along the way, must involve implementing systems that identify ethical risks throughout the organization, from IT to HR to marketing to product and beyond. What’s more, as Blackman points out, “these tools can be great for those on the front lines of defense, but they also need the ability to elevate concerns to senior executives and relevant ethics experts. Engineers and data scientists often don’t receive institutional support and the skills, knowledge, and experience to systematically, exhaustively, and efficiently answer ethical questions are dispersed across the organization.”
There is no single right way to operationalize AI ethics given the varying values of companies across dozens of industries. Regardless of the approach a company takes, the best measure of its effectiveness is simple: does it make it more trustworthy?
Here are Blackman’s seven steps to operationalizing ethical AI, as outlined in HBR:
- Identify existing infrastructure that a data and AI ethics program can leverage
- Create a data and AI ethical risk framework that is tailored to your industry
- Change how you think about ethics by taking cues from the successes in health care
- Optimize guidance and tools for product managers
- Build organizational awareness
- Formally and informally incentivize employees to play a role in identifying AI ethical risks
- Monitor impacts and engage stakeholders
Today, machine learning and artificial intelligence systems, trained by data, have become so effective that many of the largest and most well-respected companies in the world use them almost exclusively to make mission-critical business decisions. For those of us working to ensure that these decisions don’t disproportionately harm underrepresented and disadvantaged communities, we’re far from alone in suggesting that ethics in AI should be center stage in the discussion. However, we’d be doing a disservice to the movement if we didn’t acknowledge that a siloed, technology-centric approach alone cannot make AI socially and ethically responsible.
Instead, we can agree with Blackman that an approach worth exploring is one that looks at ethics from an operational perspective, integrating the most appropriate ML infrastructure tools and processes where appropriate.