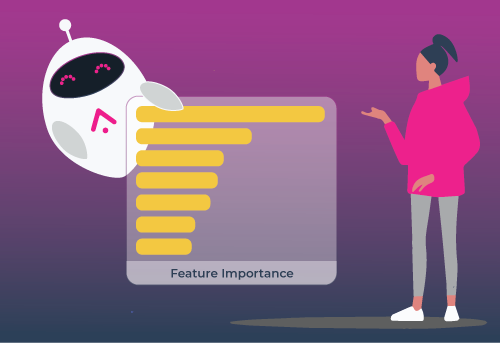
What Are the Prevailing Explainability Methods?
Welcome to “The Slice,” a new blog series from Arize that explains the essence of ML concepts in a digestible question-and-answer format.
Learn more about how Arize can help you tackle explainability or request a trial.
What Is Explainability in Machine Learning?
The adoption of machine learning (ML) has resulted in an array of artificial intelligence (AI) applications in the growing areas of language processing, computer vision, unsupervised learning and even autonomous systems.
As models increase in complexity, the ability to introspect and understand why a model made a particular prediction has become more and more difficult. It has also become more important, as ML models make predictions that increasingly influence important aspects of our lives — from the outcome of home loan applications to job interviews, medical treatment or even incarceration decisions.
Explainability is a technique designed to determine which model feature or combination of features led to a specific model decision.
For example, did a model decide that an animal was specifically a labrador because of the eyes? Nose? Ears? Or was it a combination of sorts?
A machine learning model might deliver an answer based on a seemingly unjustified interpretation. To understand why an inference is given, explainability approaches are used. This allows model builders to improve the models in more intentional and programmatic ways to produce desired results or to regulate standards. It’s worth noting, however, that explainability does not explain how the model works — rather, it offers a rationale to interpret human-understandable responses.
The goal of this piece is to highlight different explainability methods and demonstrate how to incorporate them into popular ML use cases.