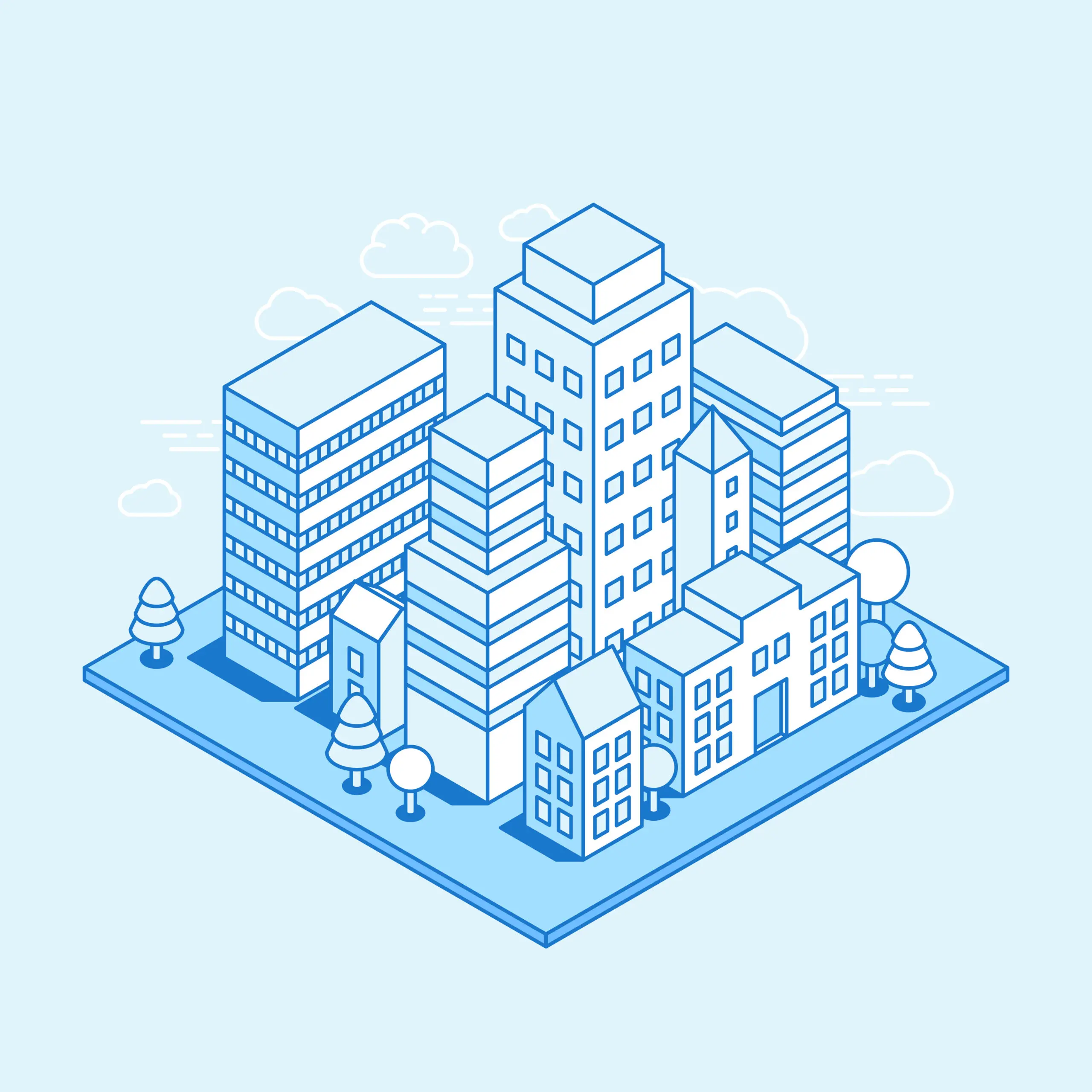
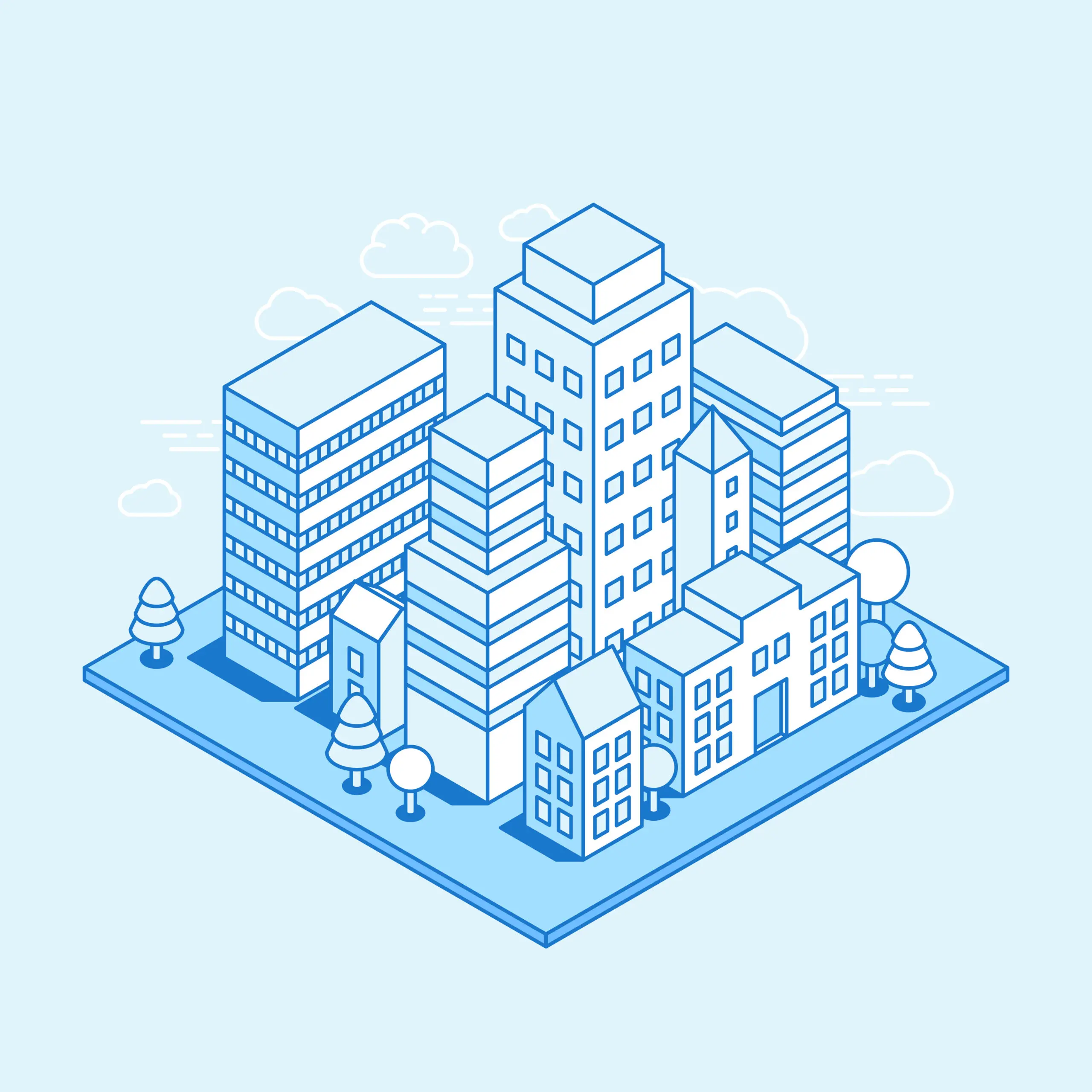
The AI Ecosystem is a MESS
The State of AI in Businesses
Over the last several years, there’s been a rush to find out how to integrate AI into businesses, and it’s no secret that doing so could offer huge comparative advantages. But for all the hype, AI in businesses is still very much in the early phase.
Our team hails from Uber, Google, Facebook and Adobe where we’ve seen both the positives and challenges of deploying AI across business lines. Most companies don’t have the same resources to build in-house tools, deeply measure results and fund extensive research. Our goal with this blog is to use our in-depth knowledge of the AI space to make sense of the ecosystem, cut through the hype, and provide insights that can help you with your AI investment decisions across the pipeline.
In-House Data Science versus External Partner
Many companies are leveraging AI to upend areas of business by applying models to common business problems in various business verticals. We consider these companies as vertical business solutions — they sell software that is “powered” by AI. They target various teams such as customer service, marketing, finance and ops with a SAAS solution to solve their problems. The other set of companies are those selling software solutions to empower your Data Science, Data Engineering and ML engineering teams.
The rest of this blog is focused on companies in the latter space, AI/ML Infrastructure companies that are building software to help your teams build and deploy models themselves.
Complexity of the AI Space
One of the first problems we hear from enterprise teams is that it’s extremely hard to figure out the right problems to solve with AI. Even when they find the right problems, we commonly hear that most of the models that they develop never make it to production. It’s such a common problem that we know of a company building a model to predict whether other models will actually make it to production. You have to wonder if that model itself will make it into production.
At various Data Science team sizes and scaling points, you find that there are new unique complexities that arise out of the Data Science organization. Some of the complexities are really software engineering problems that have taken on a new form and others are unique to this space. All of this is compounded by the confusing suite of software tools and solutions that are built to “help” Data Science. Enter stage left, where you have a 100+ companies who are pitching confusing software solutions to “Empower your Data Science teams”.
How to Evaluate ML/AI Software Companies
AI/ML Infrastructure tools is an extremely crowded space. I can point to 100+ companies offering services in hyper-parameter tuning, deploying, MLOps, governance, control, auditing, regulation, explainability, performance tuning, you name it. Even as a technical person, I’ve been pitched by some ML tools companies, where after an hour pitch, I still don’t know what they do.
The nebulous quotes abound include “Achieve AI scale”, “Optimize AI”, “Empower AI”, “Take control of your AI” — but what do these software solutions actually do?
There is a simple mental model we use to section the space, place the solutions, and cut through the hype.
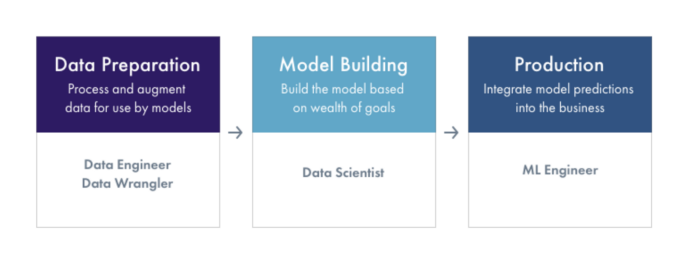
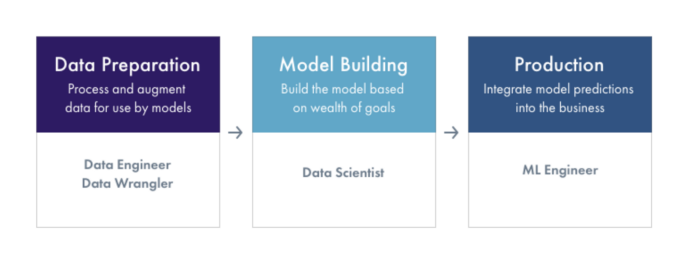
Production or Pre-Production?
As you look at the ML/AI Infrastructure space, the first segmentation of products to make is pre-production or production.
Pre-Production
- Is the Software helping you build a better model, auto-choose the right model, make the model build process reproducible, audit model builds, produce better data for model builds, or track training runs?
Production
- Is the Software helping you integrate your model into your business, scale your model into your product, troubleshoot problems in production, explain results for the rest of your team, or provide A/B effective analysis of models?
The reason this segmentation matters is that as a Data Science team grows, different people in the organization are eventually responsible for production versus pre-production. Software solutions built for one function/organization don’t serve all people well across the organization.
Model Workflow Stages
The next segmentation of products to make is to where in the ML workflow does the product fit. Broadly, we see three main stages — Data Prep, Model Building, and Production.
Data Preparation involves preparing, labeling, and cleaning the data to be used for models. It’s where the lion’s share of time and energy goes in terms of using AI models, and it’s primarily under the domain of data engineers and data wranglers.
Model building involves feature selection, training pipelines, hyper-parameter tuning, explainability analysis, pre-production audits and evaluation. It is usually taken on by data scientists.
Finally, there’s production, which involves deploying models for inference (generating predictions), performance monitoring, troubleshooting and production based explainability. It’s usually in the machine learning engineer wheelhouse.
Each of these broad categories of the ML Workflow (Data Prep, Model Building, and Production) has a deeper set of features. Here’s a more detailed overview of the ML Workflow stages with product capabilities needed in each stage and companies offering features in those verticals today.
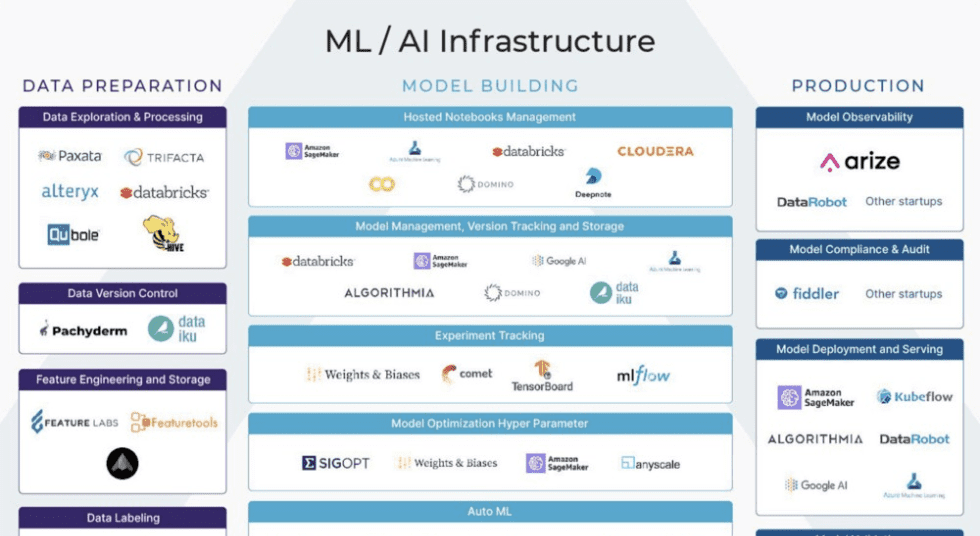
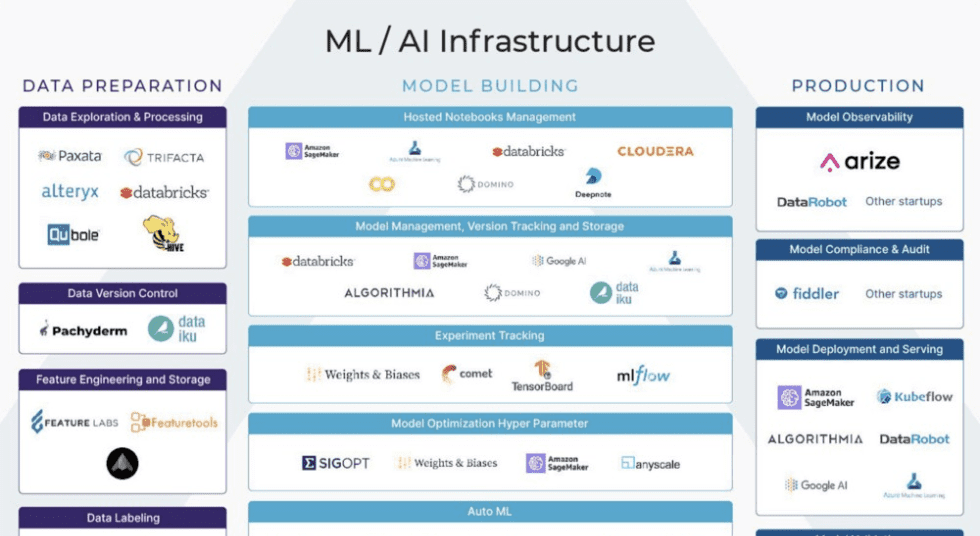
There is a strong debate whether each platform should be tailor made for going deep in the vertical or a horizontal solution makes sense. We will dive deeper into the various verticals and products in the space in upcoming blog posts.
Notes on AI Company Pitches
- If a small company pitches a product that does many things across many stages, be skeptical they are just trying to figure out their business model.
- If a product requires you to upend everything in your model build & deploy process to generate value, the value created is probably not worth the disruption & risk of lock-in.
- Product pitches to improve model performance should be considered against the effort of implementation to generate that value.
Up Next
We’ll be doing a deeper dive into the production segment of the ML Workflow. Arize AI is focused on making AI successful in production and we’re excited to share more tidbits about how to get the most value of your models.
Contact Us
If this blog post caught your attention and you’re eager to learn more, follow us on Twitter and Medium! If you’d like to hear more about what we’re doing at Arize AI, reach out to us at contacts@arize.com. If you’re interested in joining a fun, rockstar engineering crew to help make models successful in production, reach out to us at jobs@arize.com!