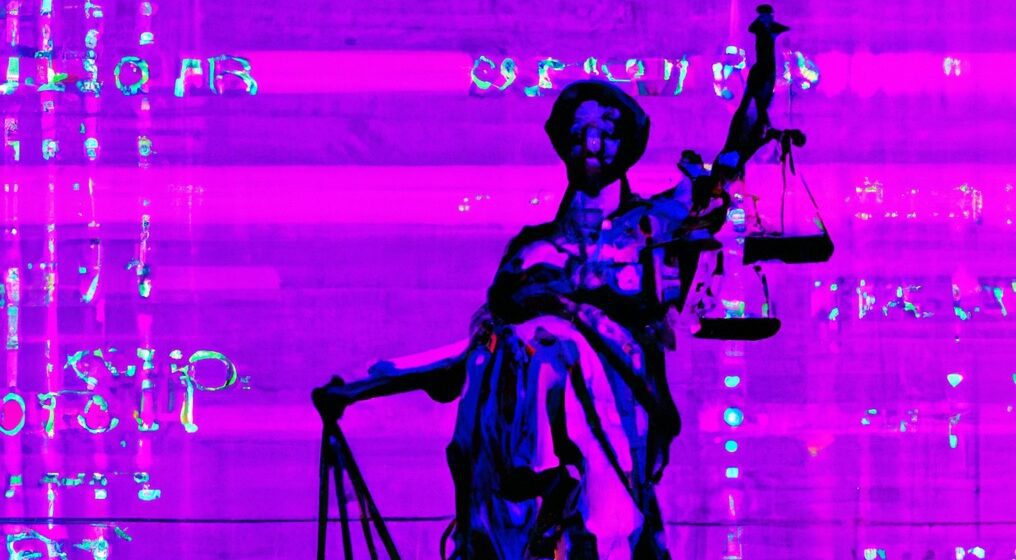
AI Ethical Issues Unraveled: Building a Fair, Transparent, and Responsible Future
In recent years, AI has permeated nearly every aspect of our lives, from healthcare and finance to education and entertainment. As AI technologies continue to advance, so do the ethical challenges associated with their development and implementation.
What are AI Ethics?
AI ethics refers to the moral principles guiding the design, development, and deployment of artificial intelligence systems. These principles are typically centered around transparency, fairness, accountability, and privacy. By adhering to a set of ethical guidelines, data practitioners can ensure that AI systems respect human rights, protect users’ privacy, and promote social good. Key areas of focus within AI ethics include bias and fairness, transparency, accountability, privacy, and security.
Bias and Fairness in Machine Learning
Bias and fairness are central to AI ethics, as they directly impact the equitable treatment of different groups by AI systems. Model fairness refers to the equitable treatment of different groups by an AI system. Ensuring fairness is essential for creating AI solutions that are not only effective but also ethical. Biased AI can lead to discriminatory outcomes, perpetuating or exacerbating existing inequalities. Ensuring fairness in AI involves addressing bias at various stages of the AI development process, from diverse data collection and preprocessing to the implementation of fairness-aware algorithms and ongoing evaluation. By focusing on fairness, AI developers can create more inclusive and equitable systems that better serve the needs of all users.
Transparency
Transparency is another key area of AI ethics, as it pertains to the openness and clarity with which AI systems operate. Ensuring that AI models are transparent helps to maintain trust, facilitate understanding, and promote accountability. However, the complexity of many AI systems, particularly deep learning models, presents challenges to achieving full transparency. Strategies for increasing transparency include creating interpretable models, using explainable AI techniques, and providing clear documentation of the development process and decision-making criteria. By prioritizing transparency, AI developers can foster greater trust and understanding of AI systems among users and stakeholders.
Accountability
Accountability in AI ethics involves establishing clear lines of responsibility for the development, deployment, and consequences of AI systems. This includes holding AI developers, users, and organizations accountable for the ethical implications of their AI solutions. Ensuring accountability in AI requires the creation of robust governance structures, the development of guidelines and standards, and the establishment of mechanisms for auditing and monitoring AI systems. Accountability is crucial for maintaining trust in AI technologies and ensuring that potential harms are identified and addressed in a timely manner.
Privacy and Security
Privacy and security are central concerns in AI ethics, as AI systems often process vast amounts of personal and sensitive data. Balancing the need for data-driven insights with the protection of individual privacy is a critical challenge for AI developers. Strategies for addressing privacy and security concerns in AI include the use of privacy-preserving techniques such as differential privacy, encryption, and federated learning, as well as the development of robust data governance policies and practices. By prioritizing privacy and security, AI developers can help to ensure that users’ personal information is protected and that their privacy rights are respected.
Consequences of Unethical Artificial Intelligence
The consequences of unethical AI practices are far-reaching and can significantly impact individuals, communities, and society as a whole. Understanding these consequences is essential for appreciating the importance of prioritizing ethical considerations in AI development.
Discrimination and inequality are among the most concerning consequences of unethical AI. Biased AI systems can lead to discriminatory outcomes that disproportionately affect certain groups based on factors such as race, gender, or socioeconomic status. This can further exacerbate existing inequalities and hinder social progress. For example, biased hiring algorithms may result in unfair treatment of minority candidates, while biased lending algorithms could deny loans to deserving individuals from underprivileged backgrounds. By perpetuating and reinforcing these inequalities, unethical AI practices can create barriers to social and economic mobility for marginalized groups.
Misinformation and manipulation represent additional risks associated with unethical AI applications. AI-generated deepfakes, for example, can create realistic but false images or videos that can deceive and mislead people. This poses a serious threat to democratic processes and undermines the credibility of information sources. To address this challenge, AI developers must focus on creating systems that promote the integrity and accuracy of information, rather than facilitating the spread of false or misleading content.
Another consequence of unethical AI is the reinforcement of harmful stereotypes. AI systems that are trained on biased data can perpetuate and reinforce harmful stereotypes, potentially entrenching existing inequalities. For example, an AI-driven advertising system may serve ads based on gender stereotypes, reinforcing traditional gender roles and further entrenching inequality. Addressing this issue requires a commitment to fairness and the development of AI systems that challenge, rather than reinforce, harmful stereotypes.
Finally, unethical AI practices can lead to a lack of accountability, making it difficult to pinpoint responsibility for harm caused by AI systems. This can result in legal challenges and a lack of recourse for affected individuals or communities, further exacerbating the negative consequences of unethical AI. To address this issue, clear lines of accountability must be established for AI developers, users, and organizations, ensuring that ethical standards are upheld and potential harms are addressed in a timely manner.
The Responsibility of Practitioners
As AI technologies become increasingly integrated into our daily lives, data practitioners have a unique responsibility to prioritize ethical considerations in their work. By focusing on model fairness, transparency, and accountability, data practitioners can help to ensure that AI systems are developed and deployed in a manner that benefits society as a whole. This includes actively seeking diverse perspectives, engaging with stakeholders, and continuously evaluating AI systems for potential biases and other ethical concerns.
AI for social good is an emerging area of AI ethics that focuses on leveraging AI technologies to address pressing global challenges and promote social and environmental well-being. This involves ensuring that AI systems are developed and deployed in a manner that benefits society as a whole, rather than solely serving the interests of a select few. Key principles within AI for social good include inclusivity, sustainability, and human-centered design. By prioritizing these principles, AI developers can work towards creating technologies that have a positive impact on the world and contribute to a more equitable and sustainable future.
Mitigating Bias in ML Systems
Effectively mitigating bias in ML systems is essential for promoting fairness and ensuring that these technologies serve the best interests of all users. Here are several strategies for reducing bias:
- Diverse Data Collection: Start by gathering data from diverse sources to ensure a more representative sample. This helps to minimize the risk of reinforcing existing biases in AI systems.
- Data Preprocessing: Carefully preprocess your data to identify and address potential biases. Techniques such as re-sampling, re-weighting, or data augmentation can help to balance underrepresented groups and reduce bias in the training data.
- Fairness Metrics and Evaluation: Regularly evaluate your AI models using model fairness metrics to identify and address any potential biases. This process should be iterative and involve ongoing monitoring and adjustment as needed.
- Collaboration and Stakeholder Engagement: Engage with stakeholders, including those from diverse backgrounds and communities, to gain insight into potential biases and develop more inclusive AI solutions. This collaboration can also help to ensure that the AI system aligns with the values and needs of its users.
- AI Ethics Guidelines and Training: Establish clear AI ethics guidelines for your organization and provide training for data practitioners to ensure that they understand their responsibilities in developing ethical AI solutions.
- Transparency and Accountability: Promote transparency by clearly documenting the development process and decision-making criteria for AI systems. Establish accountability mechanisms to ensure that developers, users, and organizations can be held responsible for the ethical implications of their AI solutions.
Summary
As generative AI technology continues to shape our world, it is crucial that we prioritize ethical considerations to create solutions that are fair, transparent, and beneficial to all. Data practitioners have a unique responsibility to develop responsible AI systems, and mitigating bias is a key aspect of this responsibility. By focusing on diverse data collection, data preprocessing, fairness-aware algorithms, and ongoing evaluation using fairness metrics, we can work together to reduce bias in AI systems. Furthermore, collaborating with stakeholders, providing AI ethics training, and establishing transparency and accountability will help to ensure that AI technologies contribute to a more just and equitable future.