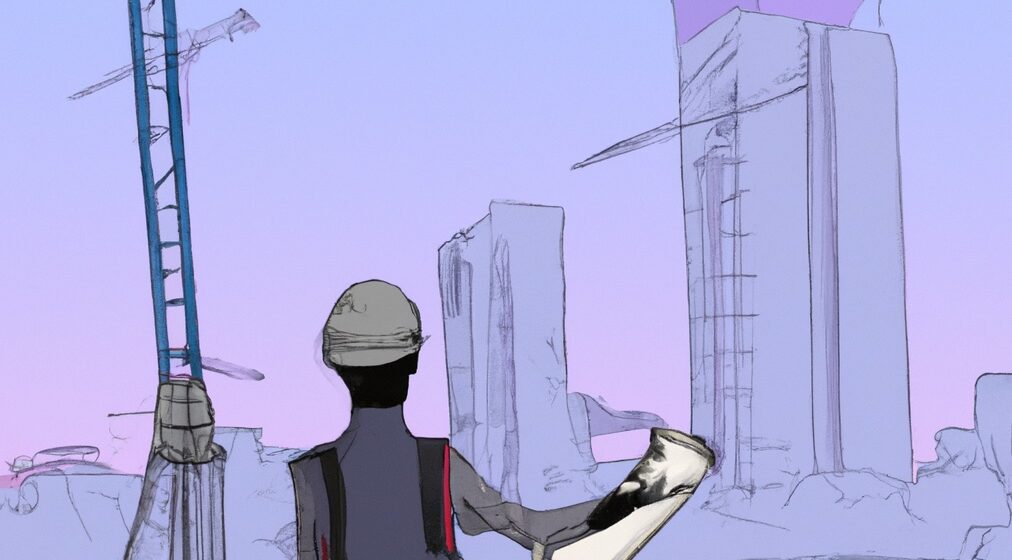
Survey: Massive Retooling Around Large Language Models Underway
Over half (53.3%) of say they plan to deploy large language model (LLM) applications into production in the next 12 months or “as soon as possible”
To paraphrase Shakespeare (the original bard), it is the best of times and the worst of times for AI. On the one hand, generative AI is fueling a technical renaissance. Models like OpenAI’s GPT-4 show sparks of artificial general intelligence and new breakthroughs and use cases emerge daily on everything from coding to generating functional protein sequences. On the other hand, the potential for things to go wrong is equally clear. Most leading large language models are black boxes that have known issues around hallucination and problematic biases and a potential for creative destruction likely unseen in generations.
Driven by these and other concerns, some have called for a “pause on giant AI experiments.” Is this likely to happen? No. In fact, according to a six-question flash poll of attendees of Arize:Observe and other ML teams conducted in April of 2023, the opposite is occurring.
Here are four highlights from our survey on the future of LLMOps.
Machine Learning Teams Are Retooling Around Large Foundational Models
The rate of adoption of large language models is astounding. Despite the fact that ChatGPT only launched in November, nearly one in ten (8.3%) of machine learning teams have already deployed an LLM application into production! Nearly half (43.3%) have production deployment plans for LLMs within a year. Only 38.3% of machine learning teams say they have no plans to leverage these models for 12 months or more.
Biggest Barriers To Production LLM Deployment: Data Privacy and Inaccuracy of Responses
Unsurprisingly, data privacy and the need to protect proprietary data are the largest roadblocks for production deployment of LLMs (a lesson that some teams are learning the hard way).
Accuracy of LLM responses and “hallucinations” are the second-largest barrier, highlighting the need for better LLM observability tools to fine-tune models and troubleshoot issues.
Of the “other” responses, cost is the most-listed concern.
OpenAI: Early Bird Takes the Lead
OpenAI dominates the field, with 83.0% of ML teams reporting that they are considering or already working with one of the company’s models as its early-mover advantage in several areas materializes. LLaMa ranks second, with nearly one in four (24.5%) saying they plan to use the model.
The Rise of Prompt Engineering
Of those using LLMs today, most (58.3%) say they are prompt engineering. Emerging techniques also appear to be graduating from the early-adoption phase. Nearly one in three (31.6%) are using a vector database, and over one in five (23.3%) are using an agent like LangChain.
Conclusion
Although this survey cannot claim to represent the entire field, its findings underscore the swift emergence and significance of LLMOps. As machine learning teams pivot towards LLMs and novel use cases surpass conventional methods, innovative strategies are essential to ensure the readiness of LLM applications for deployment and to swiftly detect issues post-deployment. Fortunately, new tools are being introduced to fulfill these requirements.