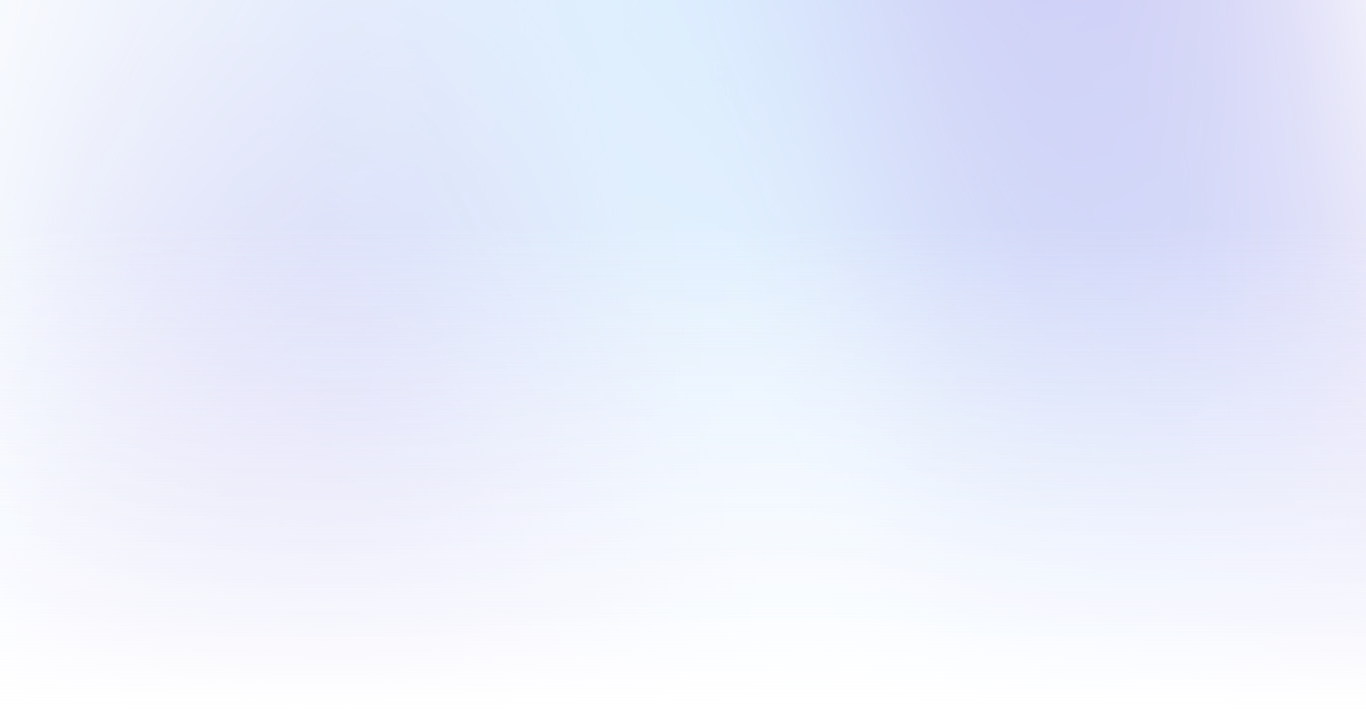
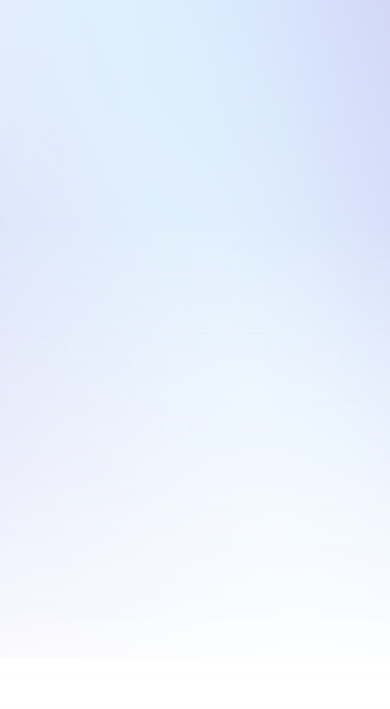
Actively Improve and Fine-Tune Model Outcomes
Uncover opportunities to proactively improve the performance of your generative, NLP, CV, and tabular ML models with tools to help focus your retraining and labeling efforts.
Automated Model Retraining Workflows
Avoid models going stale with workflows that automatically trigger retraining when your model drifts.
Keeping your models relevant takes less operational overhead with Arize.
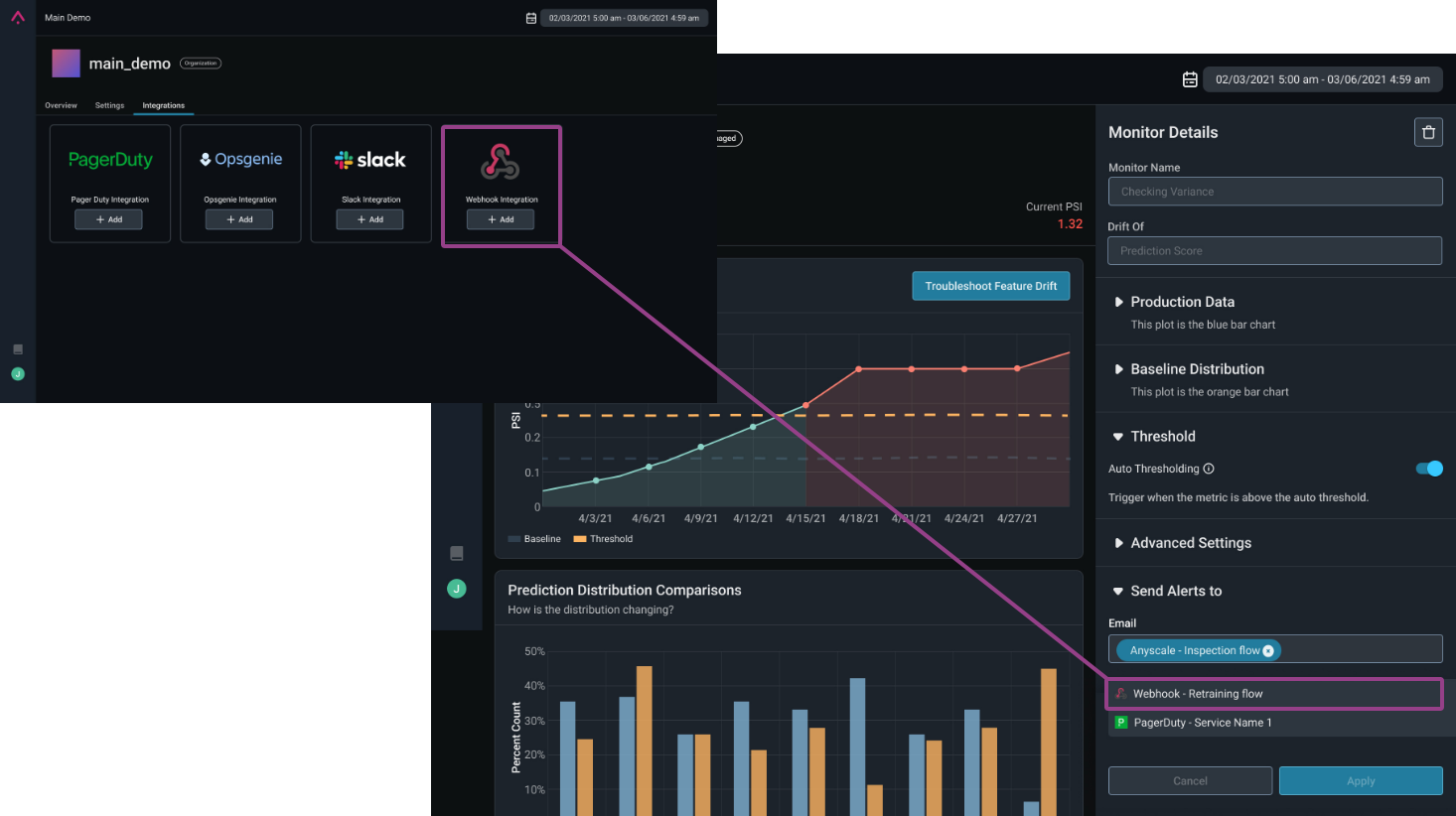
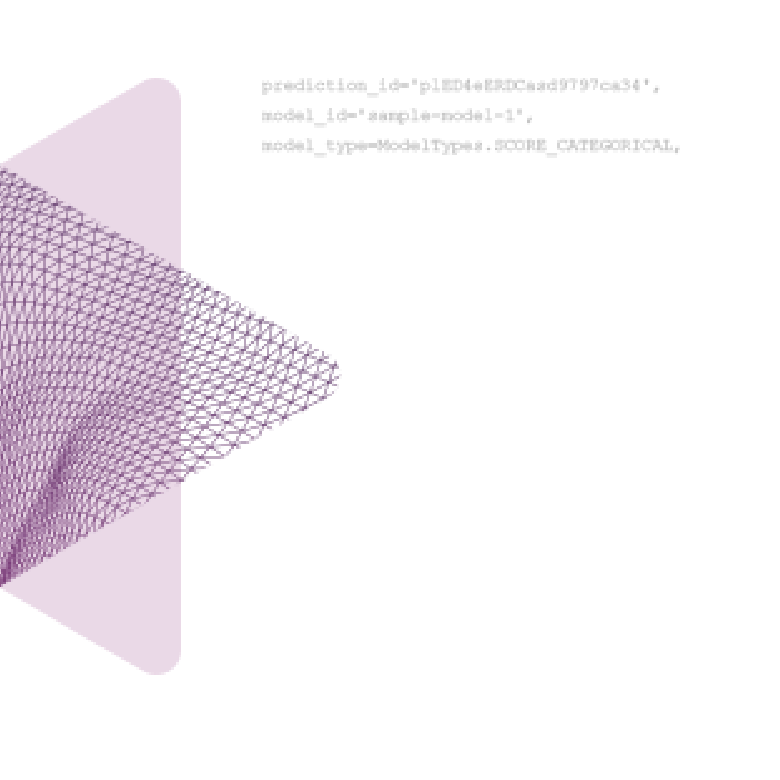
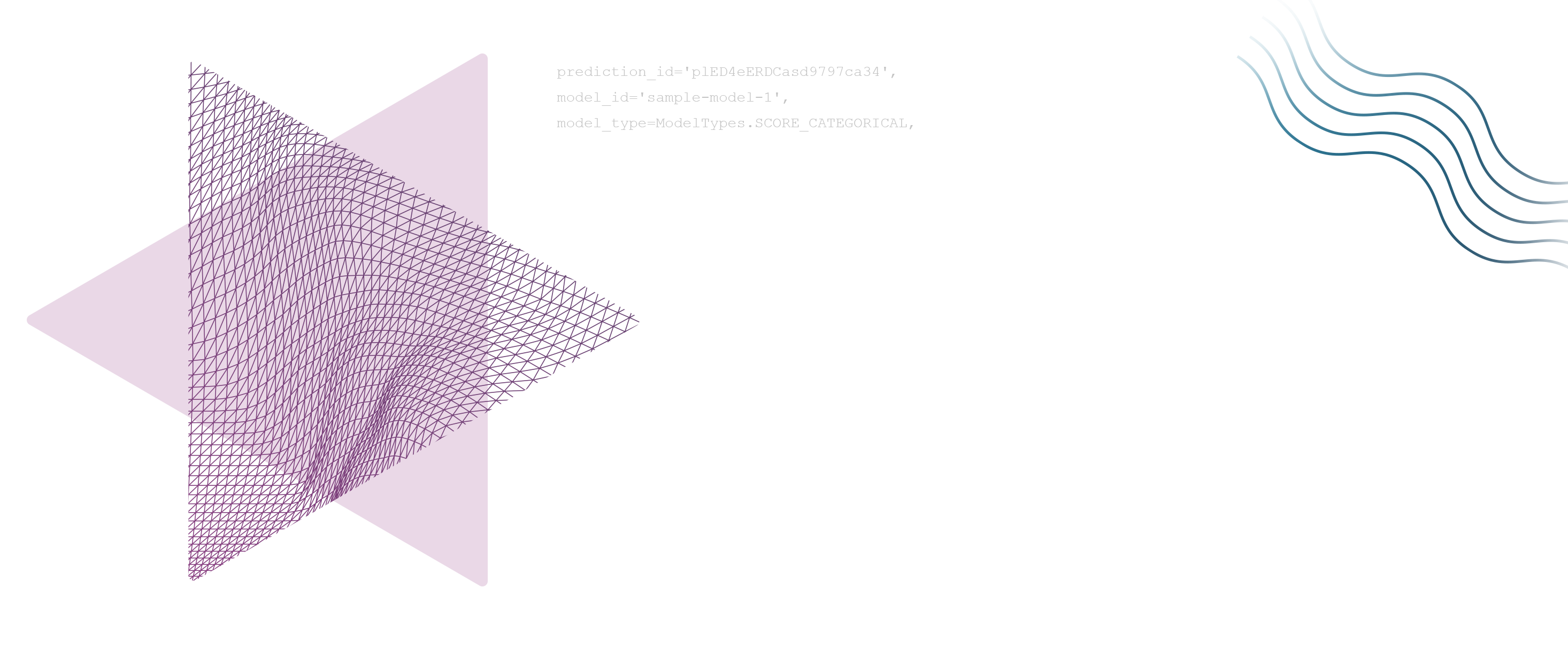
Export Back to Data Science Notebooks
Easily share data when you discover interesting insights so your data science team can perform further investigation or kickoff retraining workflows.
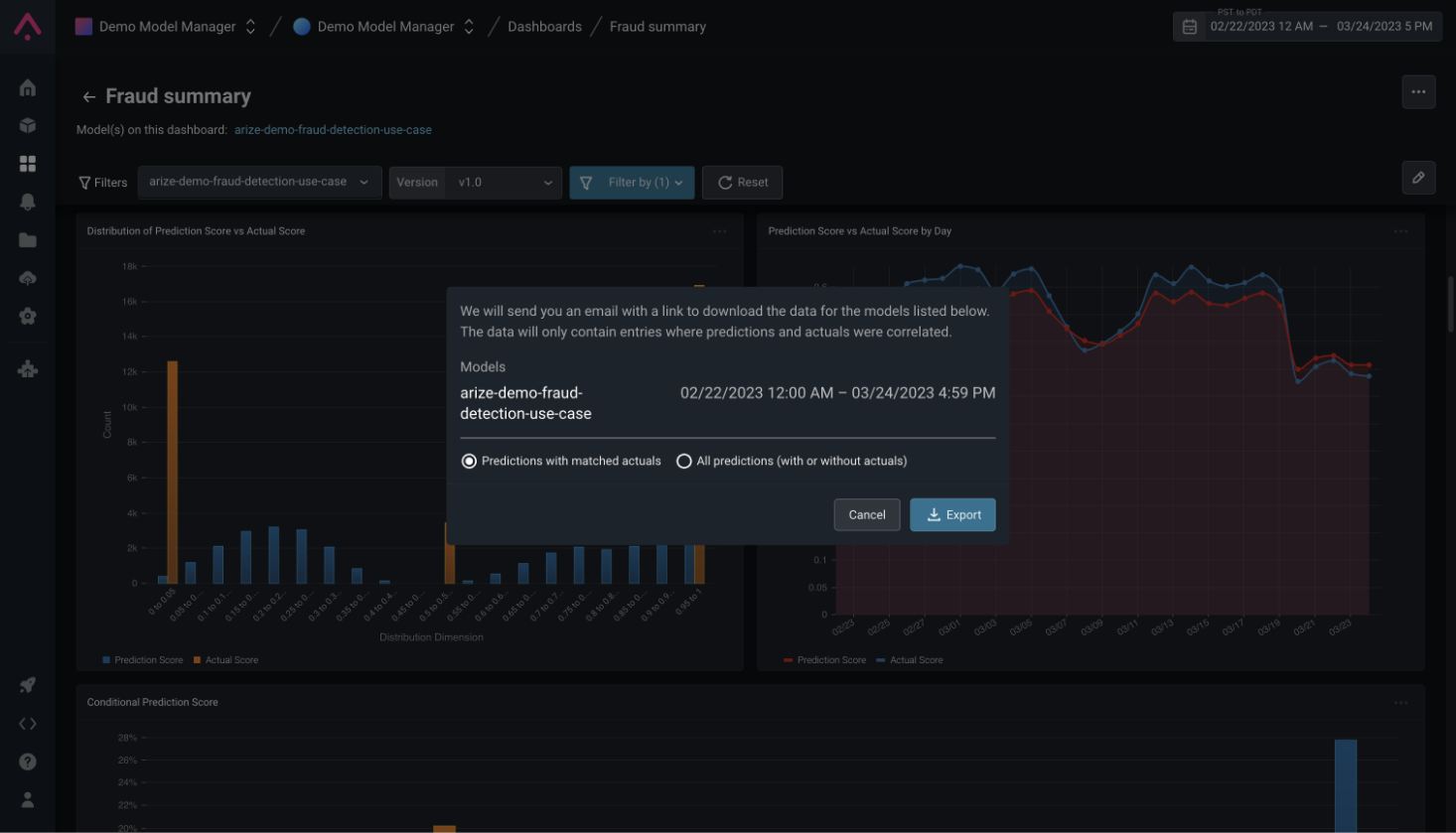
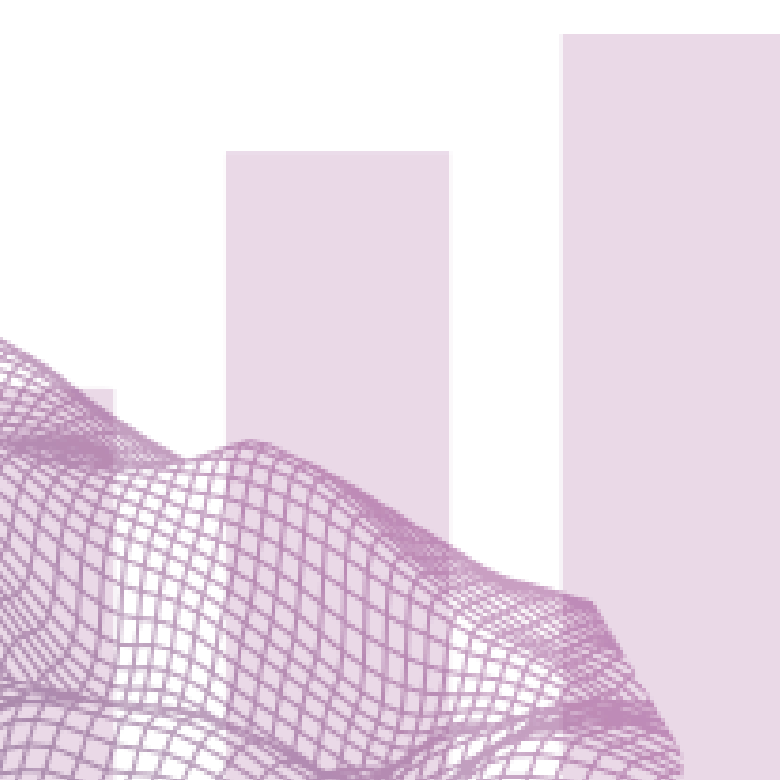
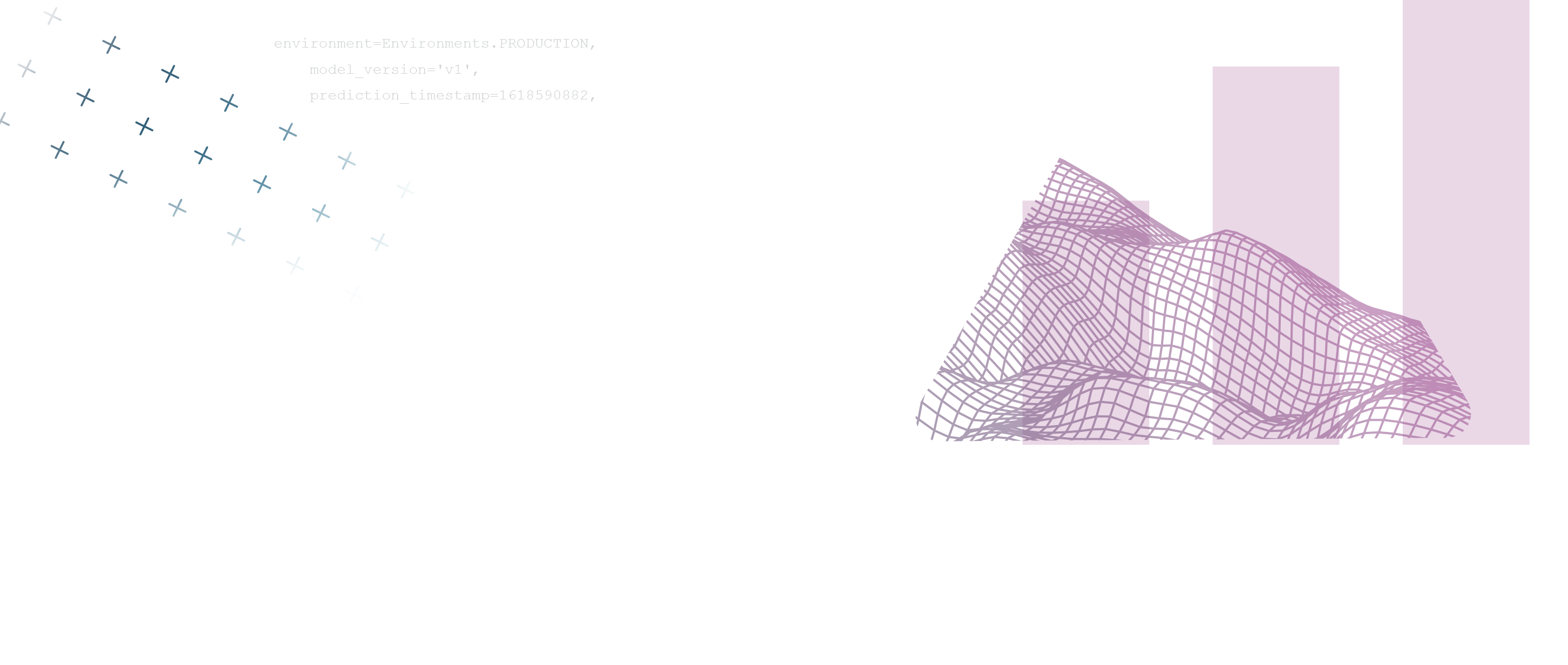
Export Embeddings Clusters for Fine-Tuning
Arize exposes clusters of data points that have drifted between your primary and baseline datasets using a combination of UMAP and clustering algorithms.
Analyze clusters by performance and drift metrics. Save and export problem clusters, such as pixelated images your model wasn’t trained on, for high-value relabeling.
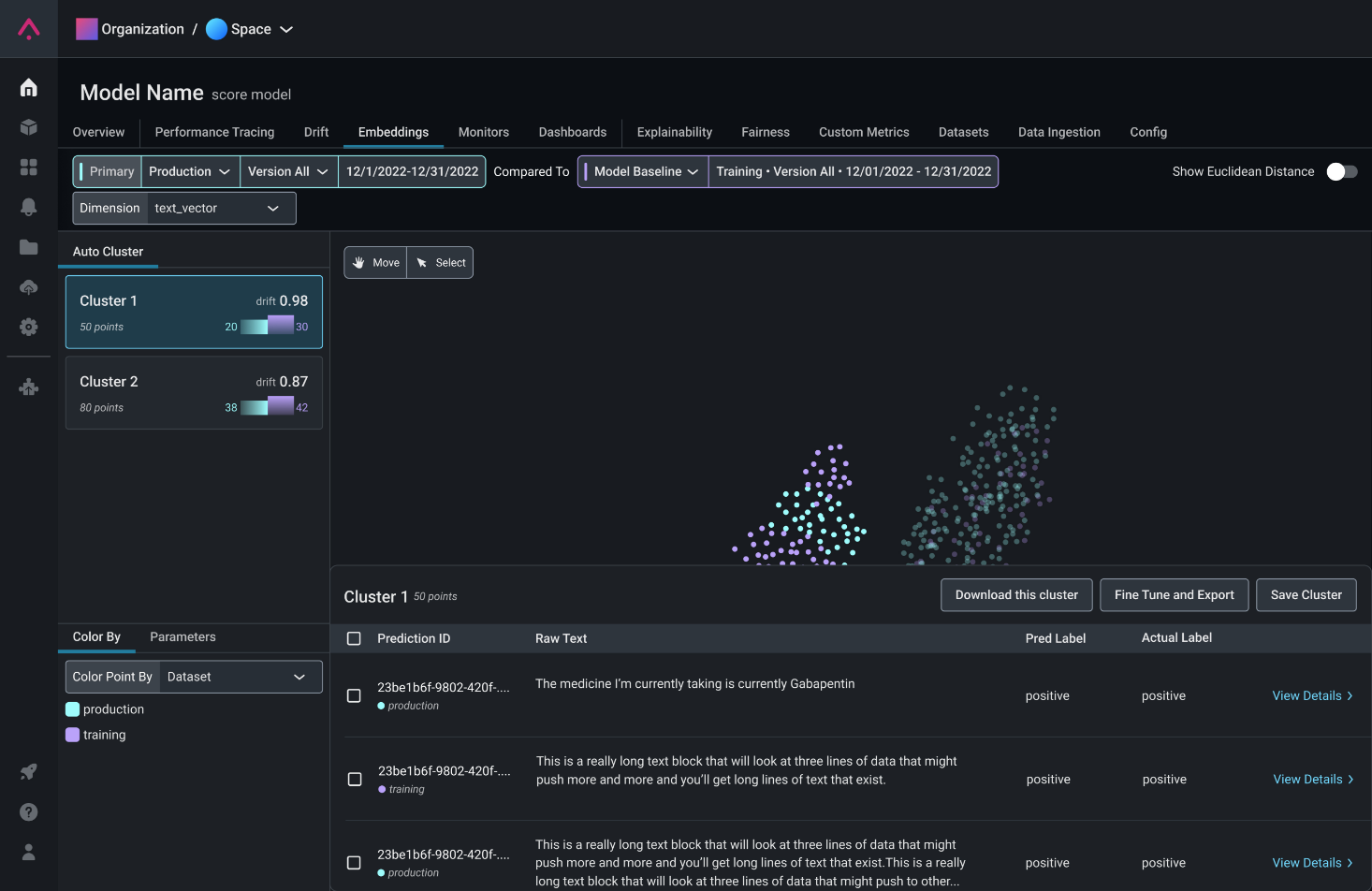
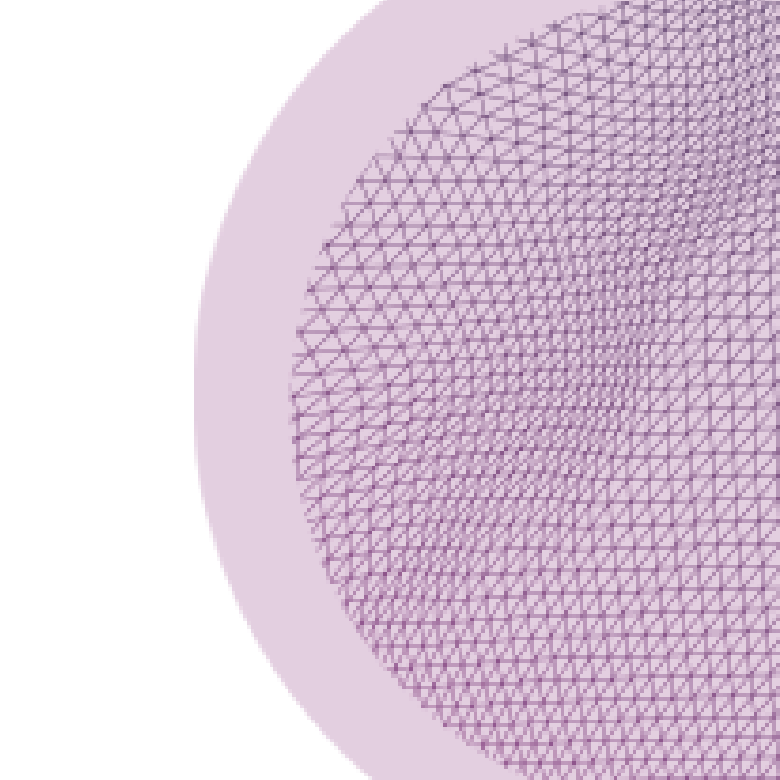
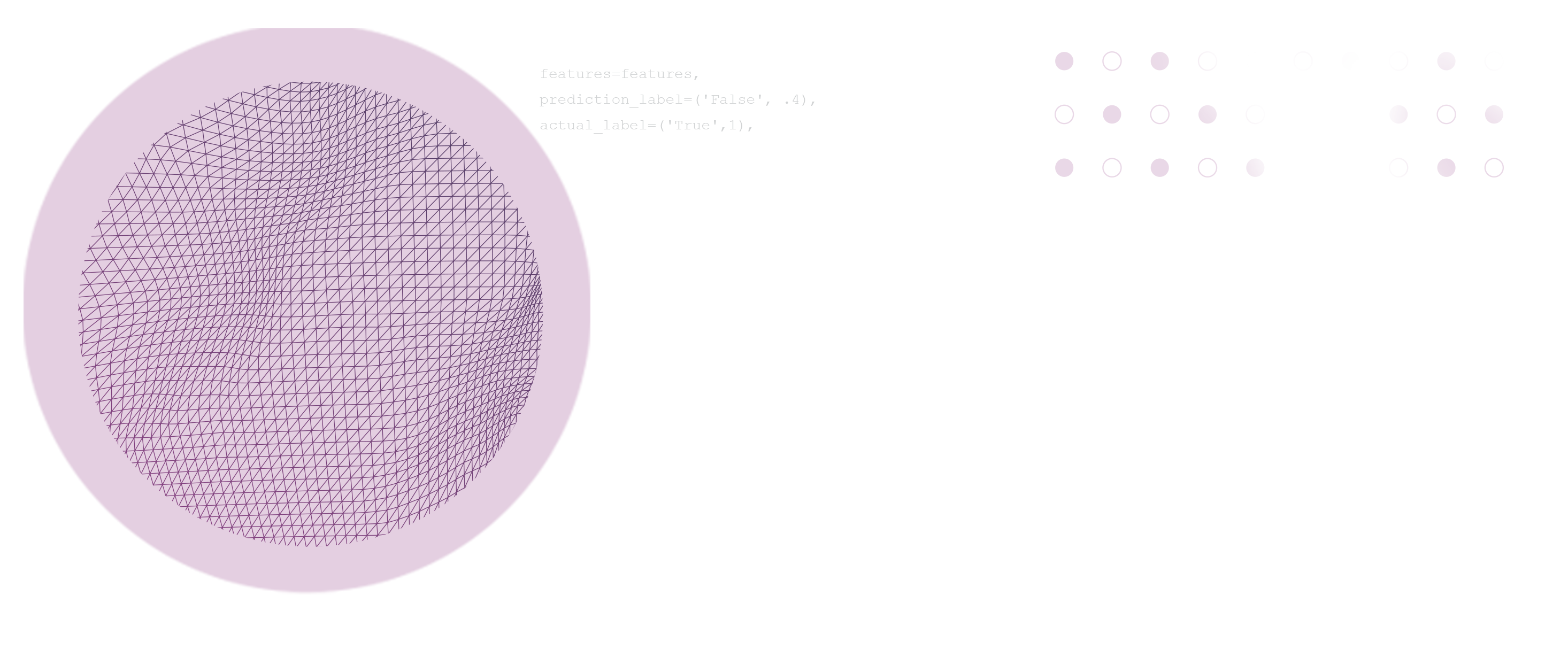
Augment Data to Improve Training
Once a problem cluster is detected for a generative model, leverage workflows to find look-a-like data.
Augment with correct responses or export for manual labeling.
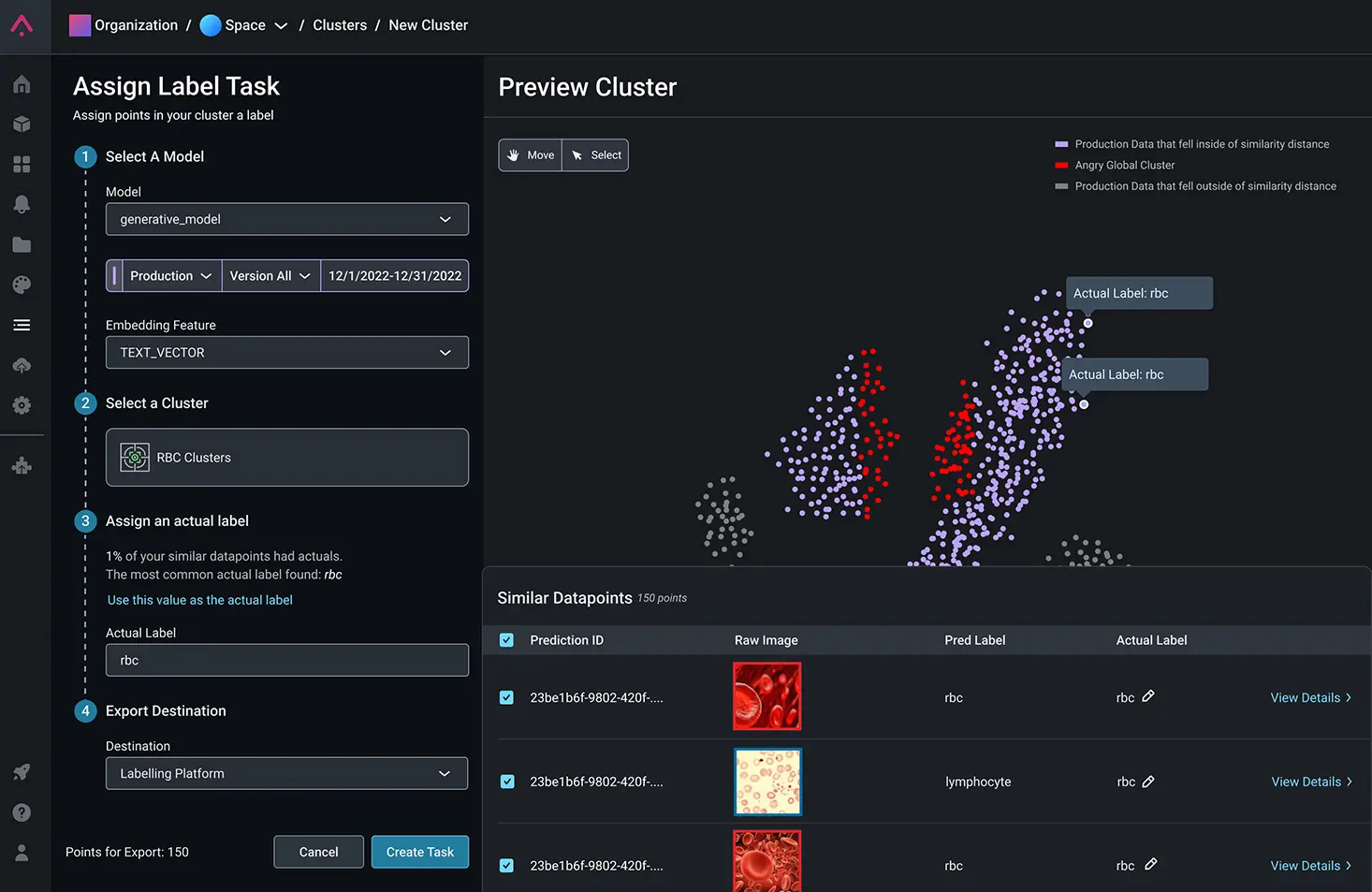
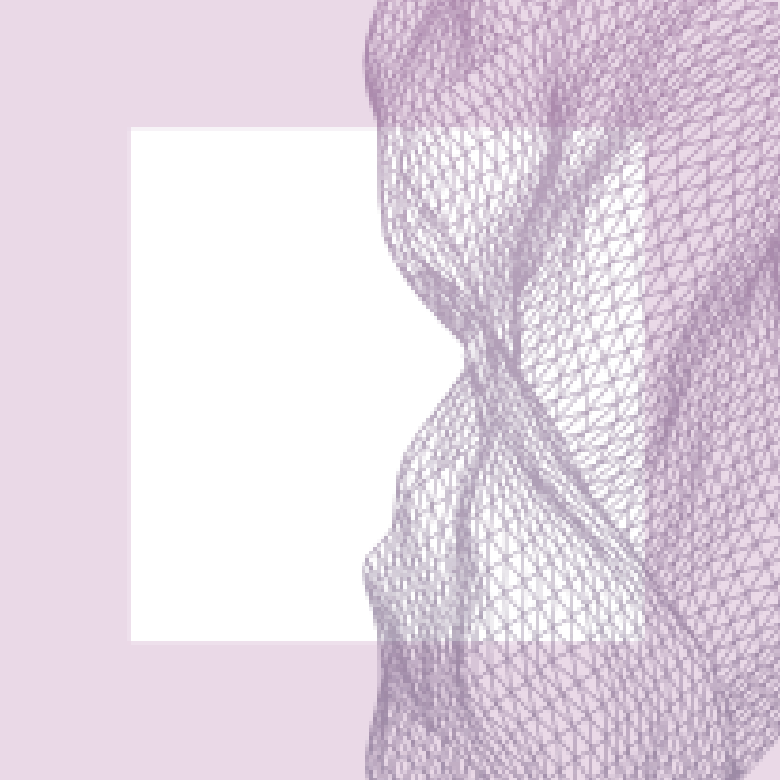
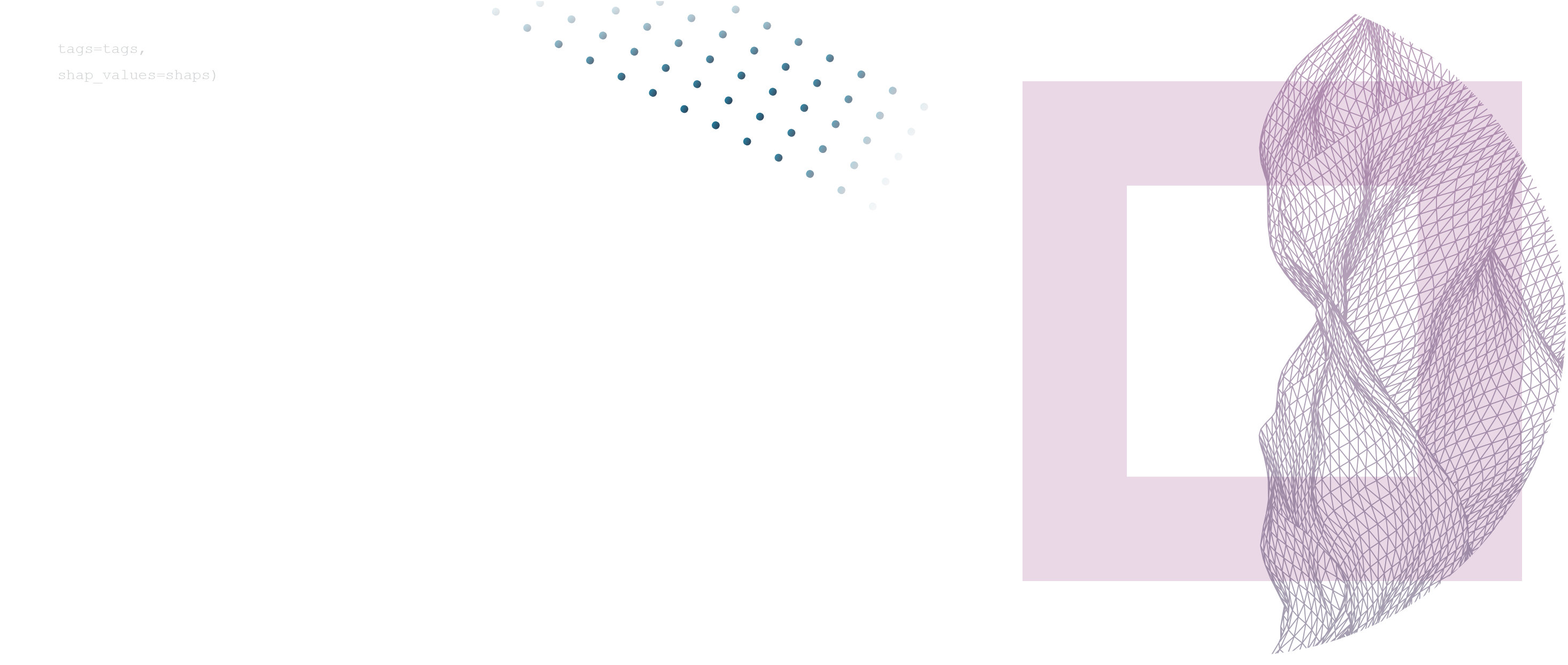
Cohort Analysis
Closely track cohorts of interest across datasets, environments, and model versions for more informed monitoring and troubleshooting.
Add or exclude cohorts of features or slices to validate the impact, and discover opportunities to improve model performance. Export insights to aid your data teams in retraining.
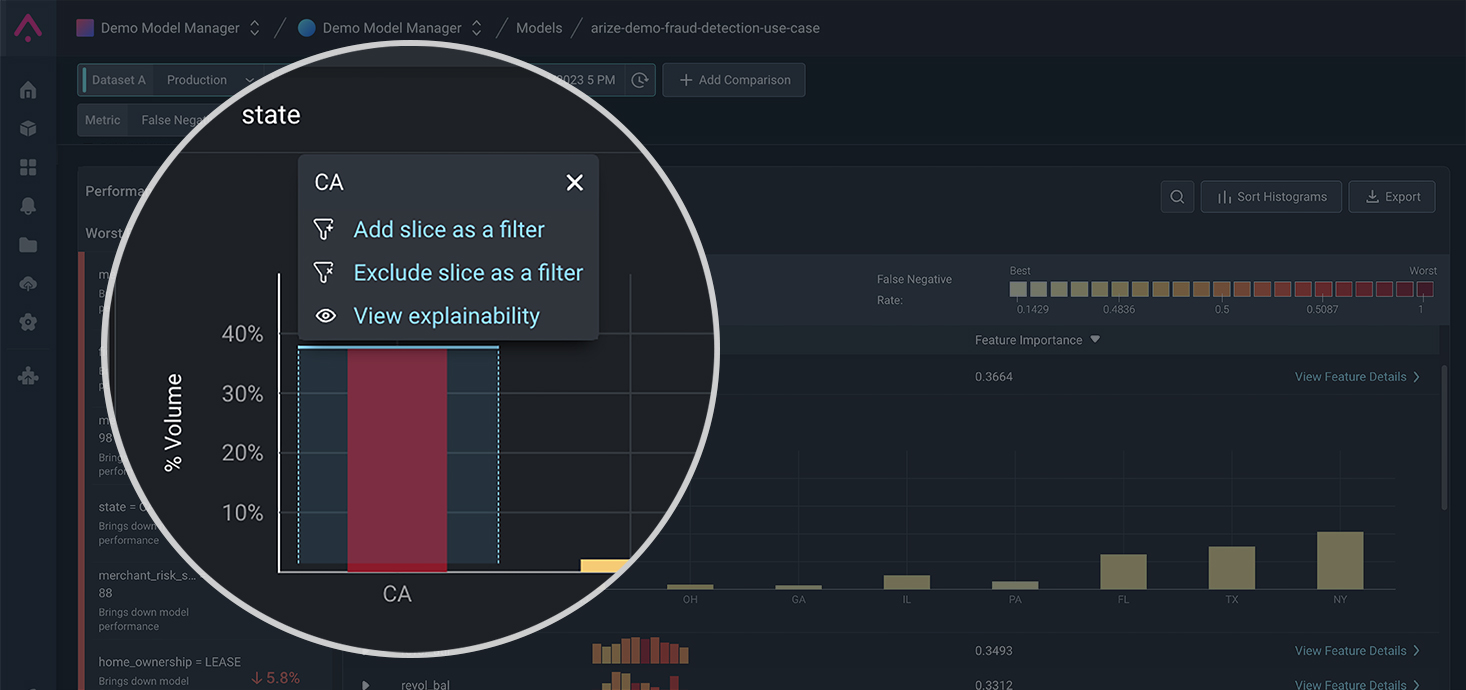
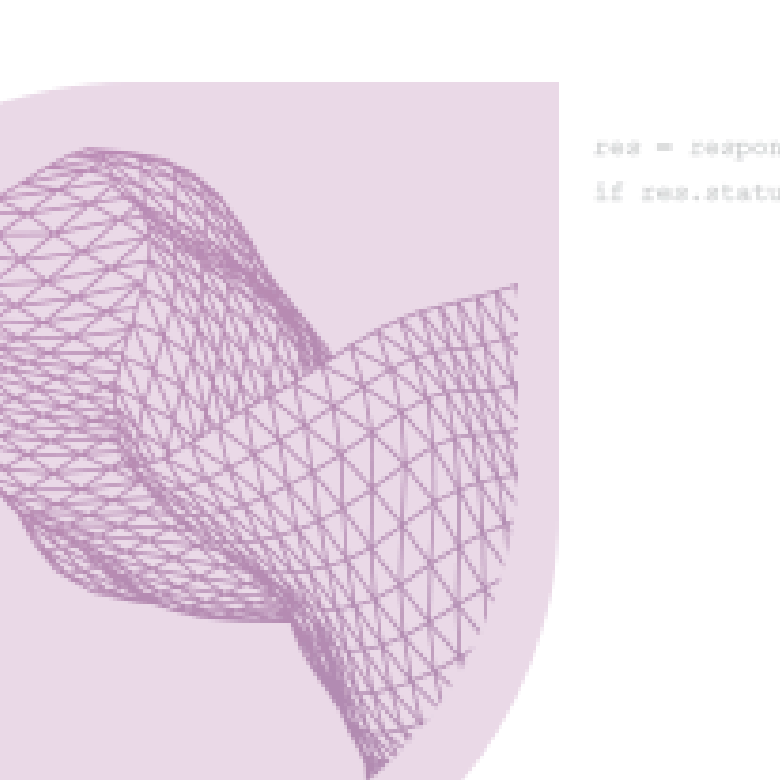
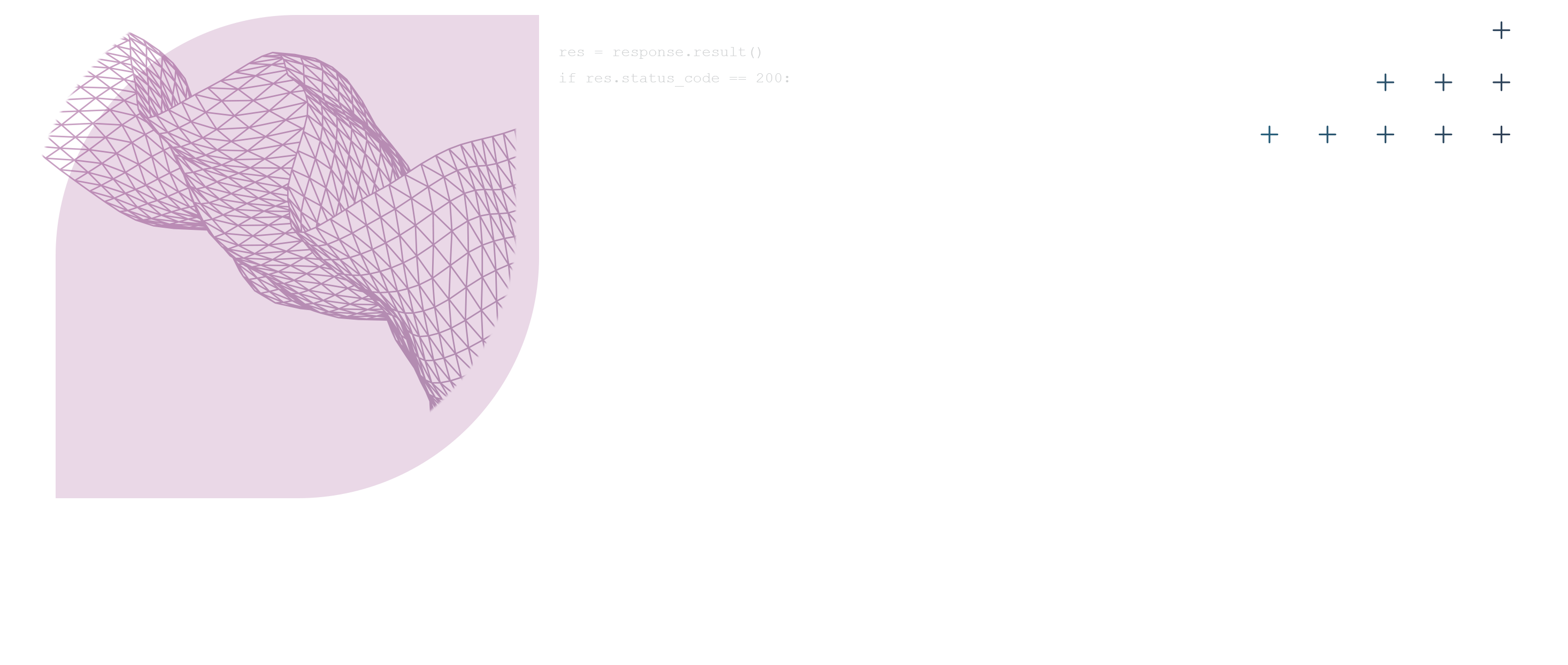
Understand a Feature’s True Impact
Prediction drift impact scores help you easily determine if feature issues actually matter to your model’s overall performance – so you can focus on the dimensions that matter most.
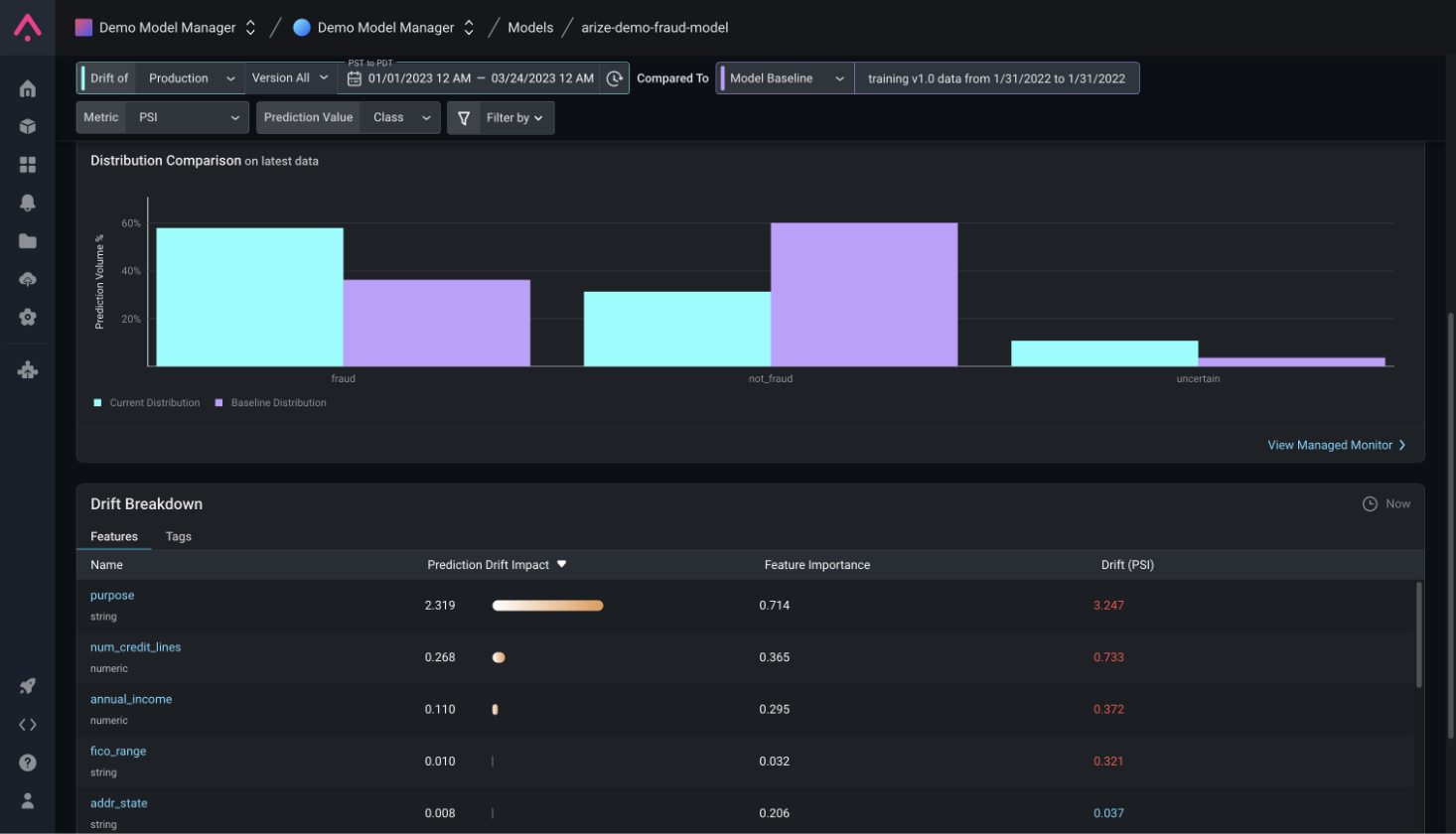
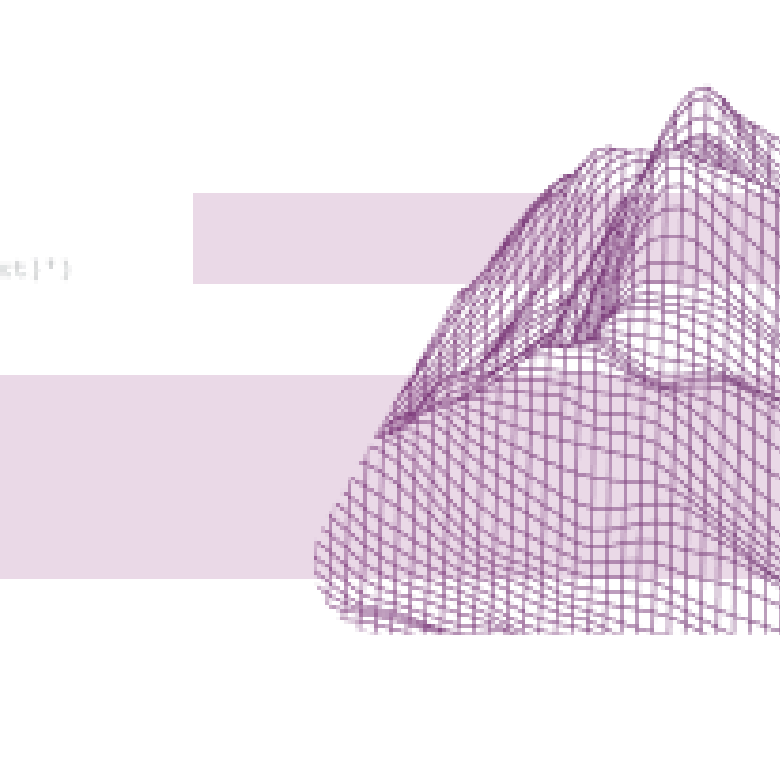
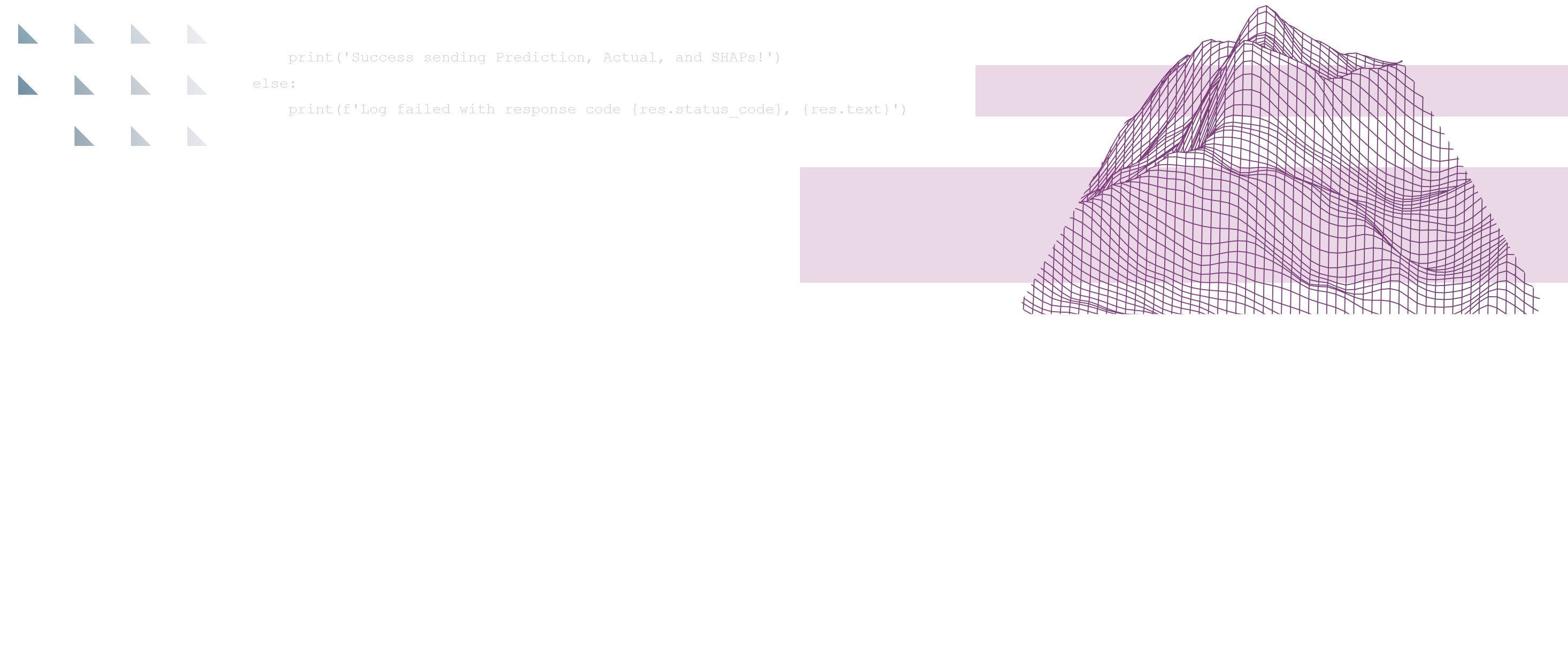