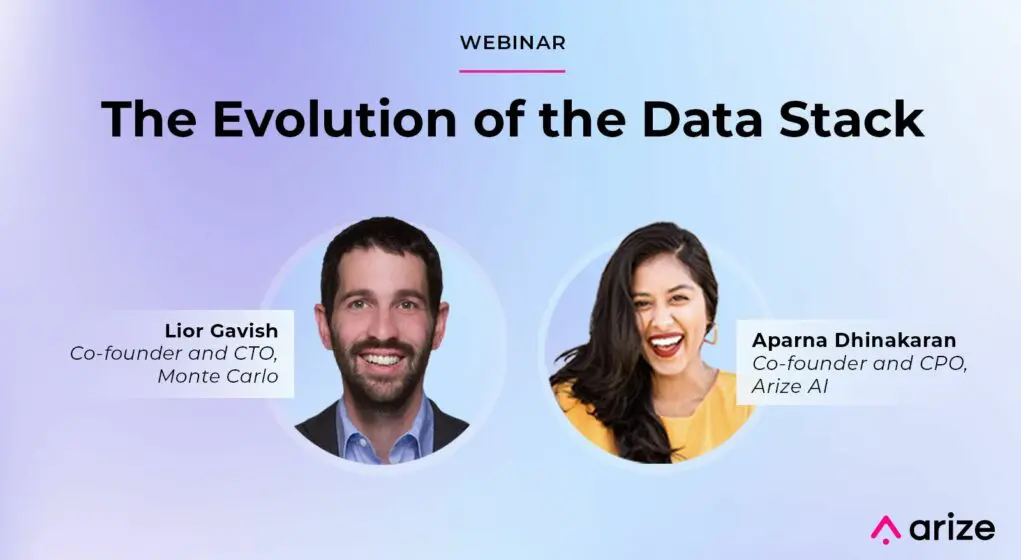
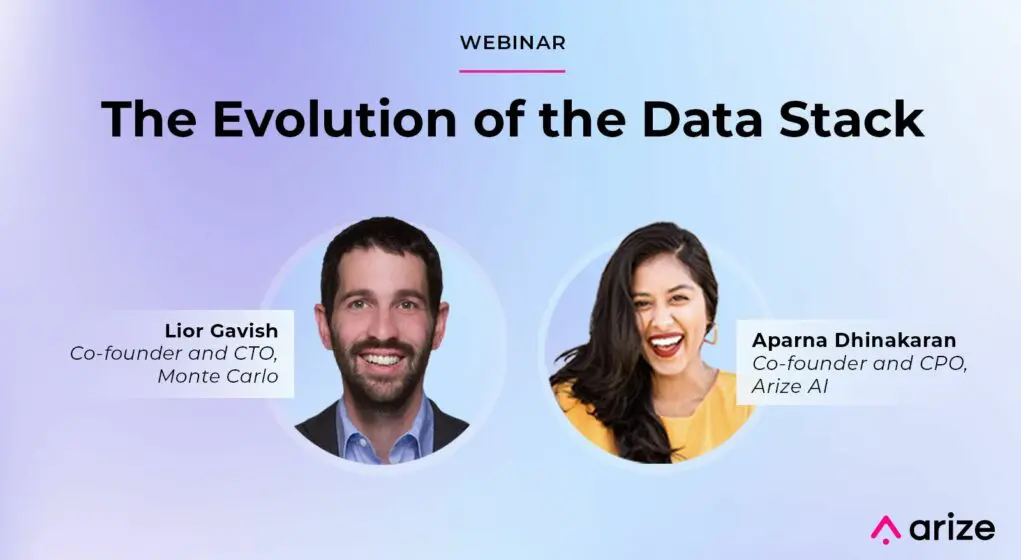
Six Takeaways From Our Event On the Evolution of the Data Stack
Recently, Arize AI co-founder and Chief Product Officer Aparna Dhinakaran sat down with Lior Gavish, co-founder and CTO at data observability platform Monte Carlo, to discuss the evolving and intertwined worlds of data and machine learning (ML) infrastructure.
Miss the event? Here are six top takeaways from the discussion.
There Are Important Differences Between Data And ML Observability (And You Need Both)
While related, there are key differences between data and ML observability. Data engineering and machine learning use separate stacks. They have different personas, use cases, and units of focus. They have distinct workflows to troubleshoot problems. But, as Lior Gavish notes, you need both data observability and ML observability to create a modern data practice.
“If you think about the way machine learning systems work in most companies, there’s a data platform that brings in data from different sources, transforms it in various ways, and then makes it available for downstream applications,” says Lior Gavish. “And one of the most powerful downstream applications is ML. People want to predict things or build features that are smart or run business processes that use ML models. Those two components are actually quite different. They’re dependent on each other, but they’re separate. Data platforms are mostly built by data engineers or analytics engineers who tend to focus on batch systems, creating data sets, and sometimes reporting on top of it. The ML platform is focused on training models using those data sets. And this is where the worlds of data and ML overlap. ML is a separate stack that draws data from the data platform that’s oftentimes built on top of data sets, but there’s a lot more complexity there. ML models could break because the underlying data used for training is wrong, but they could also break because the world changed and you’re getting new data. Or they could even break because of operational issues in machine learning.”
Observability > Monitoring
Both data and ML observability empower teams to go a step further than monitoring.
According to Aparna Dhinakaran, “Observability is about understanding why a problem occurred and knowing what actions to take to resolve the issue. When an alert goes off, it’s about knowing exactly what to do and how to fix the problem.”
Gavish adds: “On the prevention side, it’s about gaining an understanding of how platforms are operating as a whole and making foundational improvements to reduce the number of problems to track progress over time, instead of just reacting to every incident as it happens. These are critical aspects of operationalizing a system that can’t be solved with alerting alone, and it probably means taking a page from industries that have done this for a long time to find the right applications for the data stack.
Building Trust In Data and Machine Learning Starts With Investing In Systems That Help Resolve Issues Faster
According to a recent survey, 84.3% of ML teams cite the time it takes to detect and diagnose issues with their models as a pain point.
“To date, tracing model problems back to your data or input has been a very manual process,” notes Dhinakaran. “That’s what got me to launch Arize and focus on ML observability. By providing an end-to-end platform with ML performance tracing to pinpoint the data source of model problems before they impact customers or the bottom line, we’re helping teams accelerate model velocity.”
Gavish adds: “At Monte Carlo, we’re trying to make sure that, when things break, the data team knows about it first and has all the tools to resolve it quickly, communicate about it effectively to the rest of the organization, and then minimize the business impact and the softer kind of trust issues that happen when things break. This resonates with anyone who’s ever built a data system. It’s fundamental to minimize and better manage the resolution process, because these incidents are exactly the problems that diminish trust in the data platform and the data initiatives as a whole.”
Treating Data And ML As a (Real-Time) Product Can Make a Big Difference In Extracting Value from Both
“There’s a lot we can pull from product development lifecycles and bring into data and ML product development lifecycles to start treating ML as a product,” says Dhinakaran. “It’s about solving for things like data quality and performance in the real world outside of the research phase to iterate and improve over time.”
“The way we used to share data was very slow and manual,” Gavish argues. “Now we’re shifting to real-time analytics for people to make decisions in their jobs every day, ML models that make decisions on our behalf sometimes billions of times a day, and applications that use data and analytics as their underpinnings. There are other teams working on making data available to production applications, whether it’s the feature stores that make it easy to consume features for ML in a production environment in real time or companies that create APIs on top of data that allow you to use your data in a customer-facing, synchronous application.”
Service-Level Agreements (SLAs) And Reliability Benchmarks Are Gaining Ground In The Worlds of Data and ML
As the data and ML infrastructure spaces mature, service guarantees are becoming more commonplace. “I’ve definitely seen our customer base adopting SLA as a concept and implementing it in practice,” notes Gavish. “If you want to take trust and reliability seriously, you need to set SLAs and define what an acceptable reliability level is for your organization. This can change between companies, within companies, and even between different use cases. Some datasets might break more frequently. Going through the process of defining what it means for data to be up and what level of service you’re expecting to see is a critical first step. Some of the most advanced companies measure this and create dashboarding and reporting around it so they can keep themselves and their teams accountable and also track how they’re making progress towards improving the reliability of the system. Not long ago, this was something that wasn’t well understood or accepted, but we’re starting to see the industry go in this direction and that’s exciting.”
Troubleshooting Data and ML Will Get Easier Over the Next Few Years
“Datadog, New Relic, and Splunk have been around for a relatively long time compared to data and ML infrastructure,” observes Dhinakaran. “There’s a whole process of logs, metrics, tracing, and application monitoring that’s become very systemized. There are some initial best practices around these things in the worlds of data and ML observability, but there’s still a long way to go before every ML or data engineer knows what those best practices are and how to use them in their day-to-day. It took a while to make runbooks, playbooks, and best practices in the software world, so it will be interesting to see how adoption of these things happen in the data and ML worlds over the next several years.”