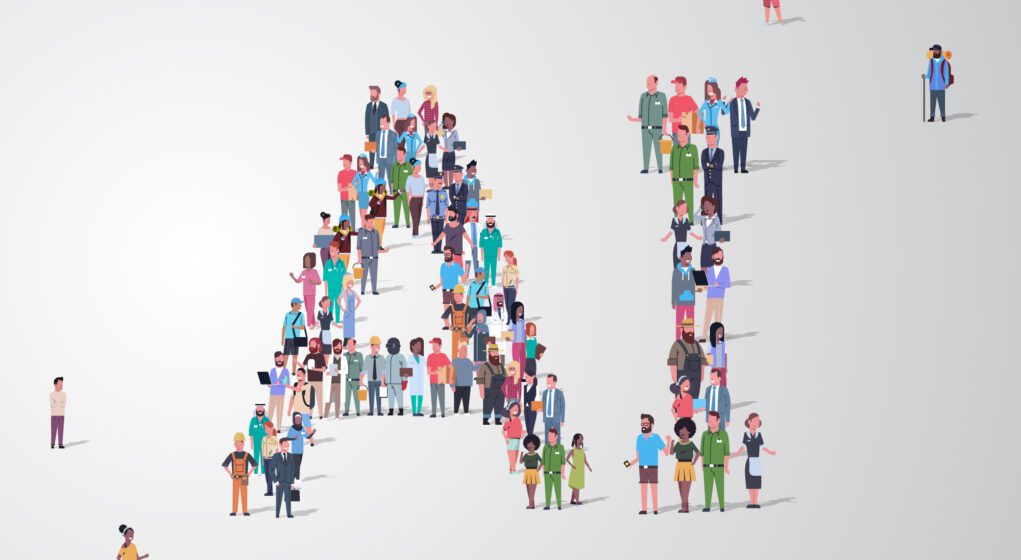
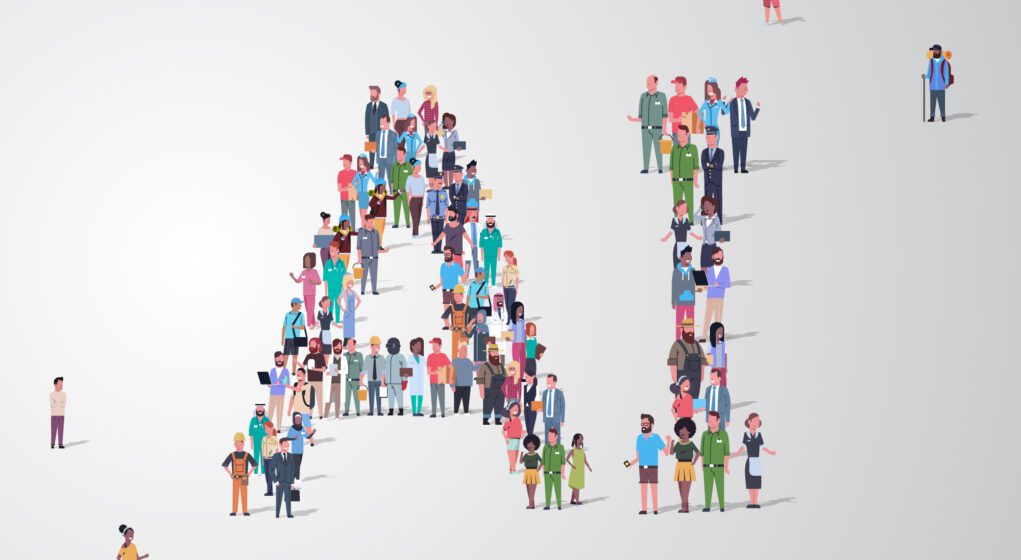
What Every Enterprise Can Do To Ensure The Long-Term Success and Sustainability of AI Initiatives
To see how Arize can help your organization accomplish the goals set out in this piece, request a trial.
Today, nearly any company – even enterprises with large teams of sophisticated data scientists and engineers – can be brought to heel by machine learning (ML) models that fail spectacularly in the real world. Whether it’s demand forecasting models upended by COVID economics or models that power HR software inadvertently discriminating against potential job seekers, problems with models are as common as they are dangerous when not monitored and caught early.
In recognition of this fact, an increasing number of companies – from Alphabet and Amazon to Bank of America, Intel, Meta and Microsoft – quietly disclose their use of AI (or its potential regulation) as a risk factor in their most recent annual financial reports.
Despite the risks, most enterprises are confidently (and rightly) plowing ahead. Today, ML-powered systems are relied on by nearly every industry to increase profitability, productivity and even save lives. In all, IDC forecasts that global enterprise spending on AI will top $204 billion by 2025.
So how can enterprises balance the tremendous power and potential peril of AI, maximizing positive outcomes for customers and society at large?
Here are five things every company can address to ensure sustainability when scaling AI initiatives.
1) The teams building and deploying AI – and the datasets used to train models – must be representative of the diversity of customers and society at large. Explicit hiring goals and ongoing data fairness audits are table stakes.
In the world of AI and machine learning, data and models can sometimes obscure the hard truths of a person’s lived experience. Since ML models are trained on historical data, they can amplify any discrimination or unequal power structures present in that historical data. Models trained on the past few years worth of housing data, for example, might reflect the continued legacy of redlining.
While most teams believe this is a problem and want to solve it, even sophisticated data scientists can find it challenging to detect and mitigate every possible fairness issue to retrain models accordingly. Often, it’s not because of bad intentions on the part of the data scientist – rather, it’s an inadvertent blind spot that is a corollary of the lack of diversity on teams.
The only real remedy to this is diversity – and explicit hiring goals along with accountability to get there in the form of executives being measured on the success of these efforts and transparency to the board or public.
2) Similar to how organizations manage privacy, enterprises should develop a codified ethical and risk governance framework for AI.
A siloed, technology-centric approach alone cannot mitigate every risk or make AI ethically responsible. The answer must involve implementing systems that identify both ethical and organizational risks throughout the company, from IT to HR to marketing to product and beyond, and incentivizing people to act on them. While technology is often a necessary prerequisite to surface the right problems, employees need to be empowered to act on these insights.
The good news is that there are a wealth of resources for an enterprise to kick off this process, from developing an organization-specific plan to operationalize AI ethics to ensuring technical teams implement procurement frameworks specifically designed with proactive model monitoring and ethics in mind.
3) Enterprises should ensure they have a modernized data policy that grants AI practitioners access to protected data where needed.
Data scientists and machine learning engineers can’t fix what they can’t see. According to a survey of over 600 data scientists and ML engineers by Arize AI, 79.9% of teams report that they “lack access to protected data needed to root out bias or ethics issues” at least some of the time. Nearly half (42.1%) say this is a frequent issue.
Moving toward a responsible AI framework means modernizing policies around access to data and in some cases expanding permissions by role. Most enterprises are good at this in software development – where access to production systems is tightly managed – but fewer have detailed governance and purpose-built systems to control access to customer data in machine learning.
It is worth noting that expanding data access need not be in conflict with broader compliance or privacy goals. While many ML teams historically lacked access to protected class data for legal liability reasons, that’s beginning to change precisely because such data across the full ML lifecycle is critical to delivering accountability and ensuring a model’s outputs are not biased or discriminatory.
4) Stop shipping AI blind.
The need for better tools to monitor and troubleshoot ML model performance in the real world – and help teams fix problems before they impact business results – is clear. Even the most sophisticated data science teams at decacorns still routinely uncover bugs impacting model performance hiding in plain sight.
Five years ago, only the most well-resourced companies had the manpower to build these tools in-house; today, a rapidly-maturing ecosystem exists to help even lean teams better validate and monitor models as they encounter issues in production.
Similar to how companies like Datadog or Splunk helped revolutionize developer operations, specialized ML platforms can help companies troubleshoot complex systems, achieve explainability of black box models, and provide guardrails when they make high-stakes decisions.
5) Grow internal visibility, open the black box and quantify AI ROI.
As AI becomes increasingly important to business-critical operations, the technical teams deploying ML models and their executive counterparts need to be in total alignment.
The following steps can help achieve that goal:
- Quantify and broadly share AI ROI: tying ML model performance metrics (i.e. F1 score) back to key business metrics – whether on customer lifetime value, churn, net promoter score or offline sales – and giving executives access to tools that visualize these metrics over time is worth the investment at the outset. Many modern tools can serve both technical and executive teams.
- Open the black box: in heavily regulated industries like finance, ML model transparency is required by law but the reality is that every industry should be able to introspect and understand why a model made a particular prediction. By investing to achieve model explainability at scale – and, more critically, perform root cause analysis when a model fails – ML teams can help executives better understand AI systems and ensure broader governance goals.
Conclusion
As companies prioritize sustainable value creation, it’s past time for AI to become a bigger part of the conversation. By taking a few simple steps, organizations can go a long way in achieving resilient and sustainable AI initiatives that benefit all stakeholders.